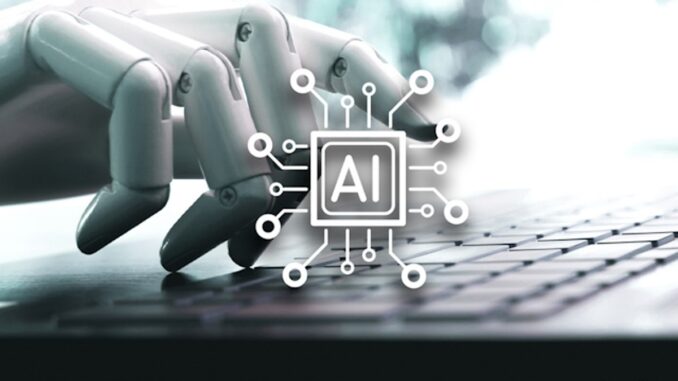
Prioritizing ethical AI involves adopting principles and practices that ensure AI systems are developed and deployed responsibly, transparently, and fairly.
This commitment is essential for gaining public trust, ensuring compliance with legal standards, and fostering an environment where technology serves the common good. Here are several strategies for prioritizing ethical AI:
### 1. Establish Clear Ethical Guidelines and Principles
– **Define Core Principles**: Organizations should establish a set of ethical principles that guide AI development and deployment, such as fairness, accountability, transparency, privacy, and security.
– **Public Commitment**: Make a formal commitment to ethical AI principles that can be shared with stakeholders, including customers, employees, and regulatory bodies.
### 2. Foster a Culture of Ethics
– **Training and Education**: Incorporate ethics training into AI and data science curricula for employees to understand the implications of their work.
– **Encourage Dialogues**: Create an open environment where employees can discuss ethical concerns and bring potential issues to light without fear of retaliation.
### 3. Implement Governance Structures
– **Ethics Review Boards**: Establish boards composed of diverse stakeholders (including ethicists, domain experts, and community representatives) to evaluate AI projects for ethical implications.
– **Clear Roles and Responsibilities**: Designate specific personnel to oversee ethical compliance in AI projects, ensuring accountability.
### 4. Engage Diverse Perspectives
– **Inclusive Teams**: Build diverse teams in terms of gender, ethnicity, background, and expertise to ensure a variety of perspectives are considered during AI development.
– **Community Involvement**: Engage with affected communities to understand their concerns and values, making sure their voices are heard in the development process.
### 5. Prioritize Transparency and Explainability
– **Explainable AI (XAI)**: Invest in research and development of AI models that provide interpretable insights about their decision-making processes.
– **Transparency in Data Use**: Clearly communicate how data is collected, used, and shared, providing users with information about AI and its implications for them.
### 6. Regular Auditing and Monitoring
– **Conduct Ethical Audits**: Perform regular audits of AI systems to assess compliance with ethical standards and identify potential biases or discrepancies.
– **Monitoring Outcomes**: Evaluate the impact of AI deployments on users and communities regularly to ensure that they align with ethical principles.
### 7. Emphasize Data Privacy and Security
– **Data Protection**: Implement robust measures to protect personal data, adhering to relevant regulations (like GDPR or CCPA).
– **Minimize Data Collection**: Collect only the data necessary for AI operations, reducing the risk of misuse or breaches.
### 8. Address Bias in AI Models
– **Bias Assessment**: Regularly assess AI models for biases in their training datasets, methodologies, and outputs.
– **Diverse Datasets**: Use diverse and representative datasets to train AI models to reduce the risk of reinforcing existing biases.
### 9. Promote Accountability
– **Clear Accountability Structures**: Define who is responsible for AI outcomes, including decision-makers, developers, and those deploying the technology.
– **Liabilities for Harmful Outcomes**: Establish policies that determine the extent of liability when AI systems cause harm or unintended consequences.
### 10. Collaborate with External Organizations
– **Partnerships and Alliances**: Collaborate with academia, civil society, and industry groups to share best practices and develop common ethical frameworks for AI.
– **Engagement with Regulators**: Work with lawmakers and regulatory bodies to craft informed regulations and guidelines governing AI use.
### 11. Promote User Empowerment
– **User Control**: Allow users to make informed choices about how their data is used and retained, enhancing user agency.
– **Feedback Mechanisms**: Create channels for users to provide feedback on AI systems, which can inform adjustments and improvements.
### Conclusion
Prioritizing ethical AI requires a multi-faceted approach that encompasses governance, transparency, inclusivity, and accountability. Organizations that commit to ethical principles are more likely to gain trust, reduce risks associated with AI deployment, and positively impact society. By fostering an ethical culture and engaging stakeholders, organizations can navigate the complexities of AI technology and its implications for individuals and communities.
Leave a Reply