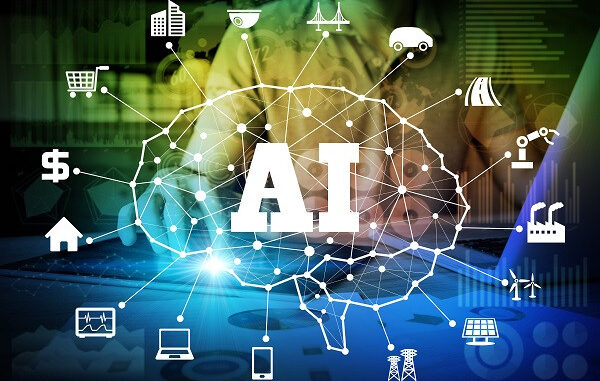
Ethical AI and data privacy are increasingly important as artificial intelligence (AI) and machine learning (ML) become more ubiquitous in various industries.
The use of AI and ML in data handling raises numerous questions about accountability, transparency, fairness, and the protection of sensitive information.
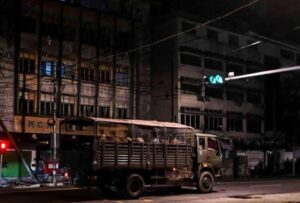
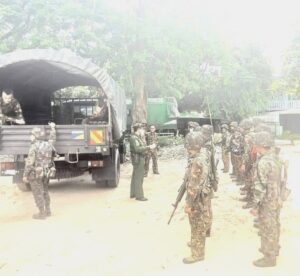
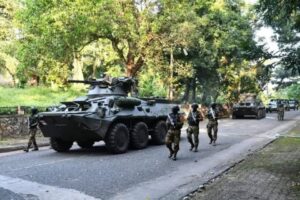
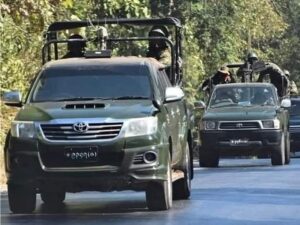
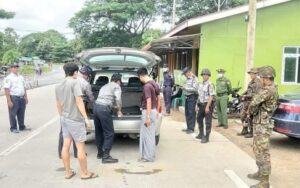
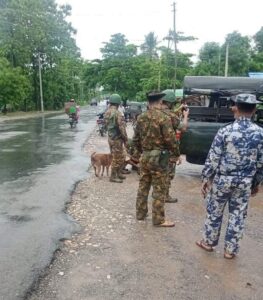

### Ethical AI Considerations
1. **Bias Detection and Mitigation**:
– AI systems can inherit biases present in the data used for model development.
– Regular auditing for bias and implementing measures to mitigate it ensures fairness.
2. **Transparency and Explainability**:
– Users have the right to understand how AI decisions are made, especially in critical areas like healthcare or finance.
– Techniques like feature attribution or local interpretable model-agnostic explanations (LIME) are used to make AI more transparent.
3. **Accountability**:
– Defining who is responsible when an AI system makes a decision or takes an action is crucial for accountability.
– Establishing clear governance and oversight mechanisms is essential.
4. **Fairness and Non-discrimination**:
– AI systems must not discriminate against individuals or groups based on protected characteristics (e.g., age, gender, race).
– Regular audits to detect and address unfair biases are necessary.
5. **Security and Confidentiality**:
– Sensitive data should be handled securely, and AI systems must adhere to data protection regulations (e.g., GDPR, CCPA).
– Secure data storage and transmission practices must be followed.
### Data Privacy Regulations
1. **General Data Protection Regulation (GDPR)**:
– EU regulation governing the handling of personal data, focusing on consent, data minimization, and security.
– Imposes significant fines for non-compliance.
2. **California Consumer Privacy Act (CCPA)**:
– US regulation aimed at consumer data protection in the digital era.
– Requires transparent data handling practices and provides consumers with more control over their data.
3. **Health Insurance Portability and Accountability Act (HIPAA)**:
– US regulation governing the handling of protected health information.
– Focuses on ensuring the security, confidentiality, and integrity of sensitive health data.
### Best Practices for Ethical AI and Data Privacy
1. **Data Minimization**:
– Collect only the data necessary for the intended purpose.
– Minimize the amount of personal identifiable information (PII).
2. **De-identification**:
– Remove identifying information from data to ensure anonymity.
– Use techniques like data masking or encryption to secure data.
3. **Informed Consent**:
– Clearly communicate how data will be used, shared, and protected.
– Obtain explicit consent from data subjects when collecting personal data.
4. **Regular Audits and Compliance**:
– Regularly assess AI systems and data handling practices for compliance with regulations.
– Address any identified issues promptly.
5. **Transparency and Communication**:
– Clearly explain AI-driven decisions to affected individuals.
– Provide information about data protection practices and rights.
### Use Cases for Ethical AI and Data Privacy
1. **Secure Personal Health Data**:
– Implementing secure storage and transmission mechanisms for personal health information.
– Using AI for personalized health predictions and recommendations while ensuring confidentiality.
2. **Compliant AI-Powered Customer Service**:
– Using AI in customer service that prioritizes data protection and transparency.
– Ensuring consent is obtained before collecting customer data.
3. **Fair and Transparent Credit Scoring**:
– Developing AI credit scoring models that are transparent and unbiased.
– Minimizing the impact of discriminatory biases and ensuring fairness.
4. **Enhanced Data Security through AI**:
– Utilizing AI for advanced threat detection and security analytics.
– Enhancing data protection by identifying security threats and vulnerabilities.
### Future Directions
1. **Developing Explainable AI**:
– Researching and developing AI that can provide clear explanations for its decisions.
– Improving transparency and accountability in AI-driven decision-making.
2. **AI for Data Protection**:
– Using AI for enhanced data protection measures, such as AI-driven threat detection and vulnerability assessment.
– Improving compliance with regulations and standards through AI-assisted monitoring and auditing.
3. **Human-AI Collaboration**:
– Designing AI systems that collaborate with humans, enhancing decision-making and data analysis.
– Fostering a culture of transparency and accountability within organizations.
4. **Data-Driven Governance**:
– Establishing governance structures that prioritize data protection and accountability.
– Leveraging data-driven insights for informed decision-making and improved data protection practices.
By prioritizing ethical AI and data privacy, organizations can build trust with their customers, users, and partners while staying compliant with regulations and maintaining a strong reputation.
Leave a Reply