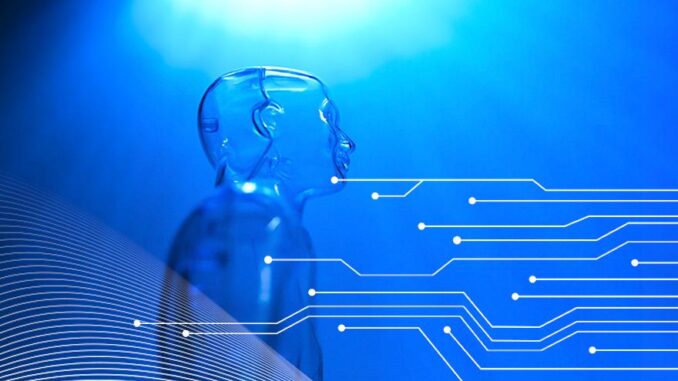
Inclusivity in AI applications is a critical topic, as the technology has the potential to impact individuals and communities in profound ways.
Ensuring that AI systems are inclusive involves addressing bias, promoting accessibility, and recognizing the diverse needs of all users. Here are key elements and best practices for fostering inclusivity in AI applications:
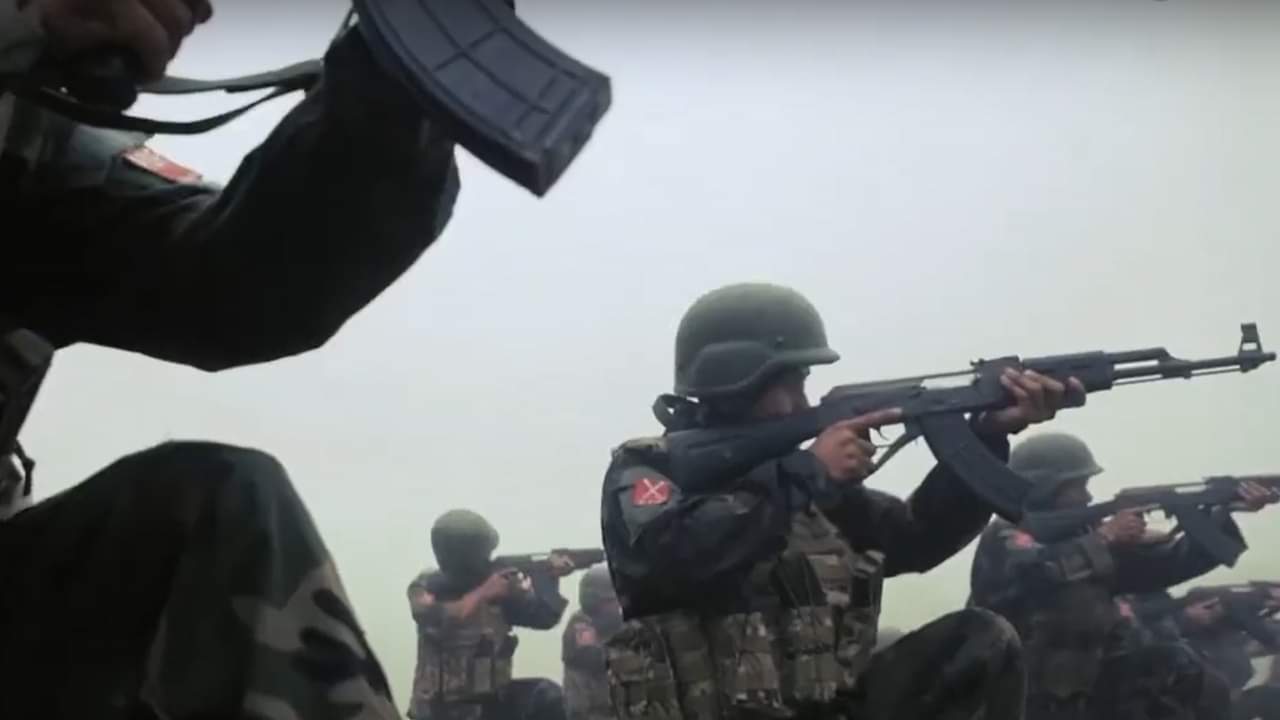
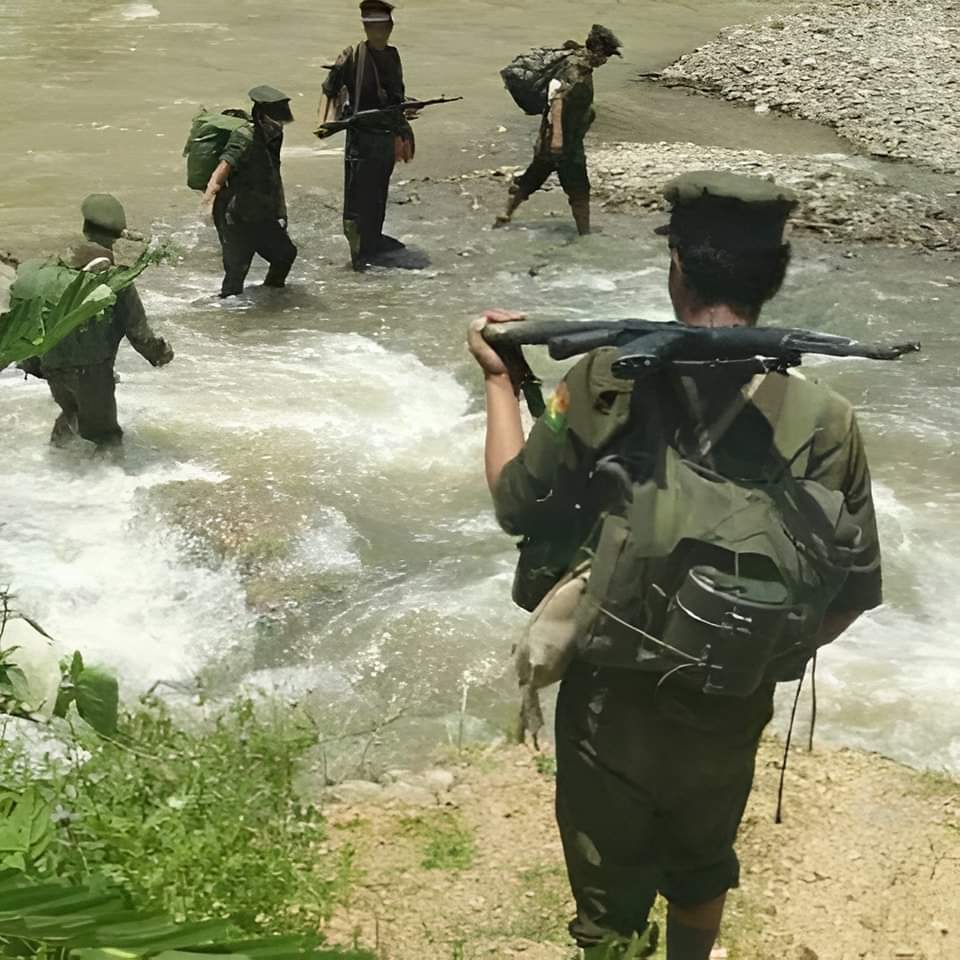
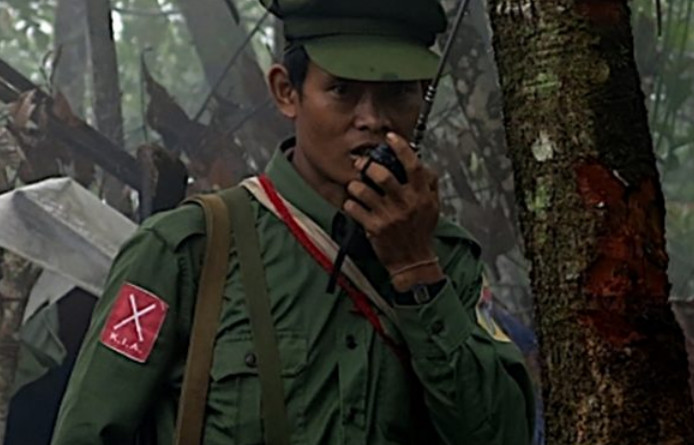
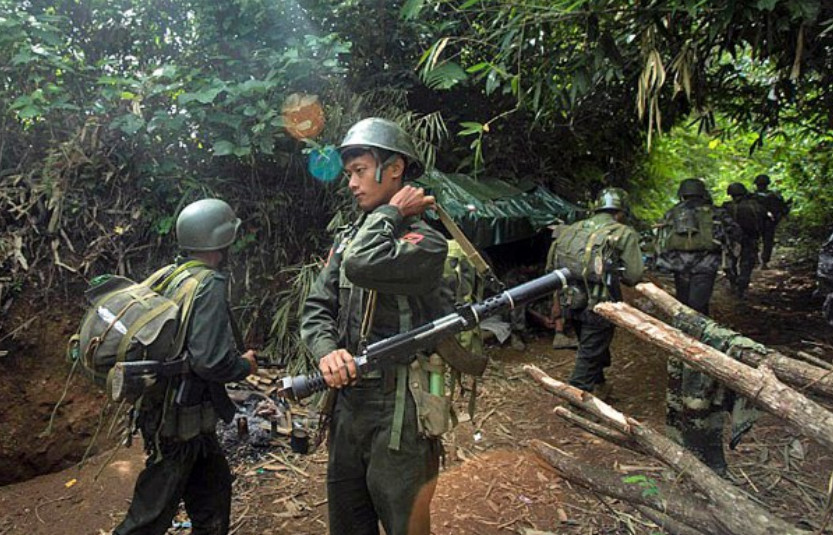
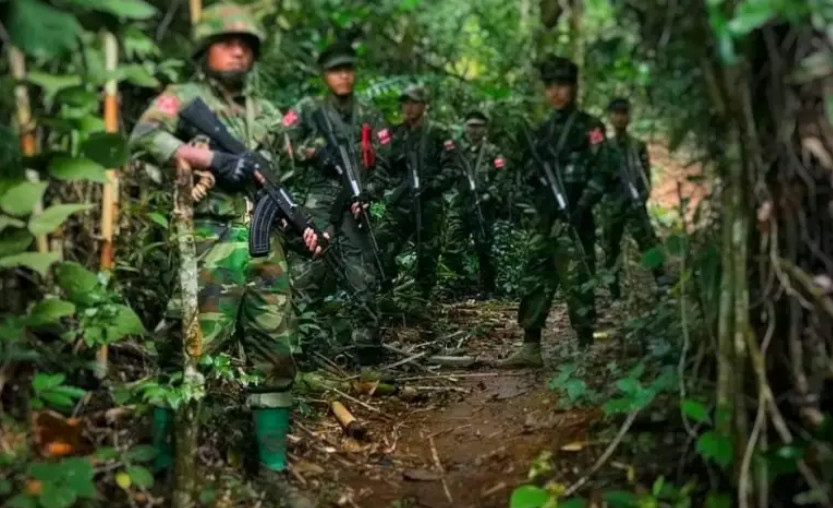
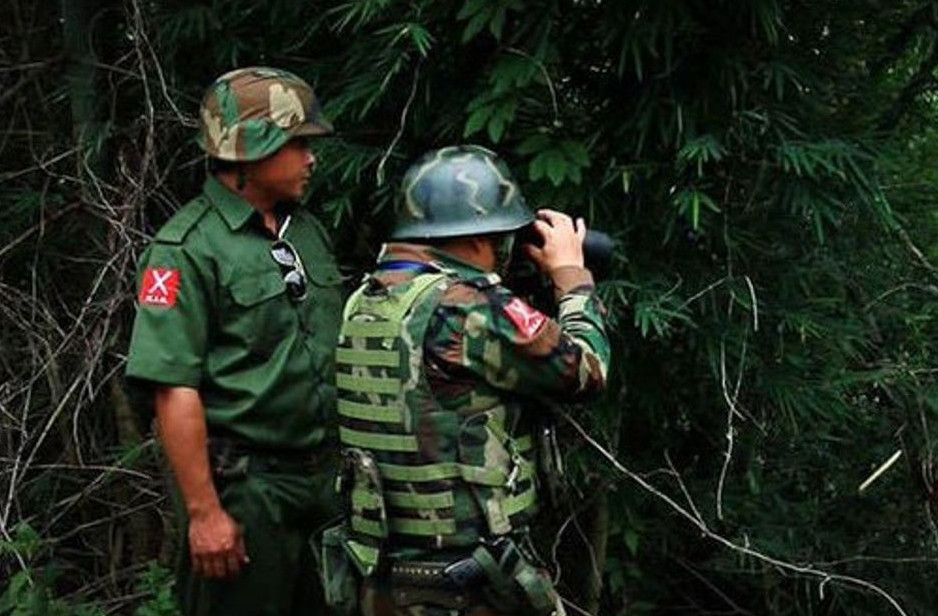
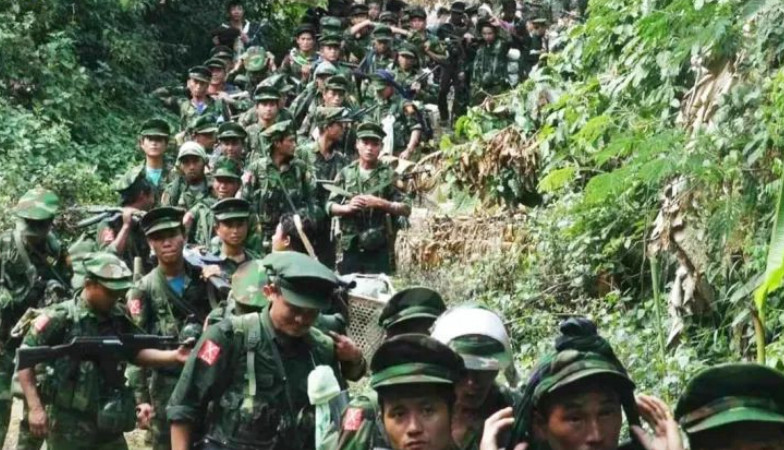
### 1. **Understanding Bias and Its Impact**
– **Types of Bias in AI:**
– **Data Bias:** Arises when training datasets are not representative of the population, leading to skewed learning outcomes.
– **Algorithmic Bias:** Occurs when the algorithms make decisions that favor one group over another based on learned patterns from biased data.
– **Human Bias:** Human decisions in the design, development, and implementation processes can introduce biases that perpetuate stereotypes or inequities.
– **Impacts of Bias:** Bias in AI can lead to unfair outcomes, exacerbating existing inequalities in areas like hiring, law enforcement, healthcare, and access to services.
### 2. **Diverse and Representative Data Sets**
– **Data Collection:**
– Ensure that datasets used for training AI models are diverse and representative of various demographics, including race, gender, age, and socioeconomic status.
– Utilize techniques such as oversampling underrepresented groups or synthesizing data to fill gaps.
– **Ongoing Evaluation:** Regularly audit and update datasets to reflect changes in demographics and social dynamics, ensuring ongoing relevance and fairness.
### 3. **Inclusive Design Principles**
– **User-Centered Design:** Engage a diverse group of users throughout the design process to gather insights, identify pain points, and understand varying needs and expectations.
– **Accessibility Features:** Implement features that cater to users with disabilities, such as text-to-speech, voice recognition, adjustable font sizes, and color contrast settings.
### 4. **Multidisciplinary Teams**
– **Diverse Development Teams:** Assemble teams with a range of backgrounds, experiences, and perspectives to bring different viewpoints into the development process. This can help in identifying potential biases and inclusivity issues before deploying AI systems.
– **Collaboration with Experts:** Work with sociologists, anthropologists, ethicists, and other social scientists to better understand community needs and the broader implications of AI applications.
### 5. **Transparency and Explainability**
– **Explainable AI:** Develop models that provide clear explanations for their outputs. Users should understand why specific decisions are made, especially in high-stakes applications like healthcare or criminal justice.
– **User Control:** Allow users to adjust settings or provide feedback on AI decisions, fostering a sense of agency and involvement.
### 6. **Regulatory Compliance and Ethical Standards**
– **Adherence to Policies:** Ensure compliance with regulations and guidelines aimed at promoting fairness and inclusivity in AI, such as the EU’s General Data Protection Regulation (GDPR) and ethical AI frameworks.
– **Ethics Committees:** Establish ethics review boards to evaluate AI projects for inclusivity and potential biases, providing accountability throughout the development process.
### 7. **Evaluation and Feedback Mechanisms**
– **Bias Testing:** Regularly test AI systems for bias using established metrics and methods, employing both automated and human evaluations to identify potential issues.
– **Continuous User Feedback:** Create channels for users to report problems, provide feedback, and suggest improvements, ensuring that their voices are heard and considered in future iterations.
### 8. **Inclusive AI Solutions**
– **Tailored AI Applications:** Develop applications that specifically address the needs of marginalized or underserved communities, such as language translation tools for non-native speakers or healthcare apps for individuals with disabilities.
– **Affordable Access:** Strive to make AI technology accessible to all, including low-income communities, by providing low-cost or free access to tools and services.
### 9. **Education and Literacy**
– **Public Awareness:** Raise awareness about AI technologies, their benefits, and their potential biases, empowering users to engage critically with AI systems.
– **Training and Resources:** Provide training resources for users to learn how to interact with AI systems effectively, ensuring that everyone can benefit from technology.
### 10. **Promoting AI Literacy Among Developers**
– **Inclusive Curricula:** Incorporate discussions of ethics, bias, and inclusivity in AI education and training programs for developers and data scientists.
– **Ethical Guidelines in Development:** Encourage developers to prioritize ethical considerations, inclusivity, and diversity in their work from the outset.
### Conclusion
Inclusivity in AI applications is essential for harnessing the technology’s full potential while minimizing harm to marginalized groups. By prioritizing diversity, transparency, and user-centeredness in AI development, stakeholders can create solutions that benefit a broader range of users and foster social equity. The ongoing commitment to inclusivity will not only improve the functionality of AI systems but also enhance their overall acceptance and trustworthiness within society.
Leave a Reply