
As of my last update in October 2023, the field of artificial intelligence has been rapidly evolving, with numerous advancements across various domains. Here are some notable developments and trends in AI:
### 1. **Generative AI Enhancements** – **Improved Language Models:** New iterations of large language models (LLMs) such as GPT-4 and similar architectures have been released, offering better contextual understanding and generation capabilities, with applications ranging from content creation to programming assistance.



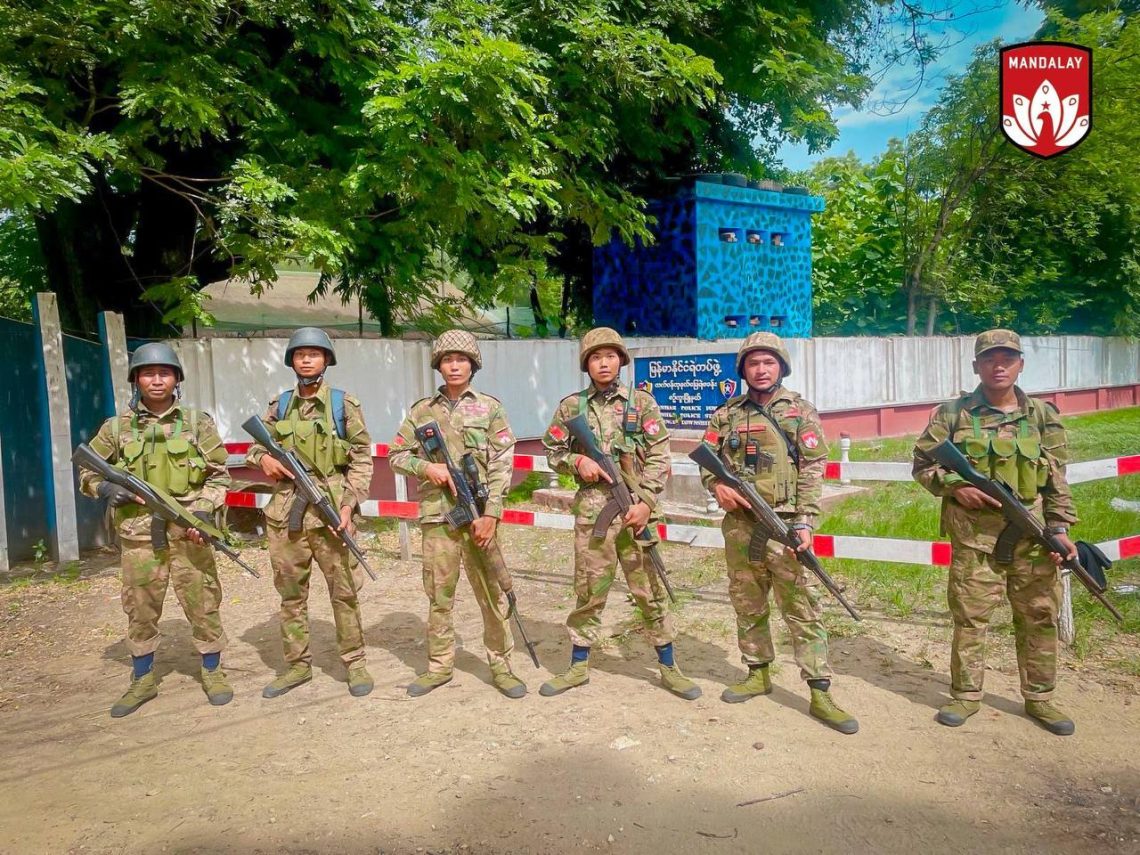
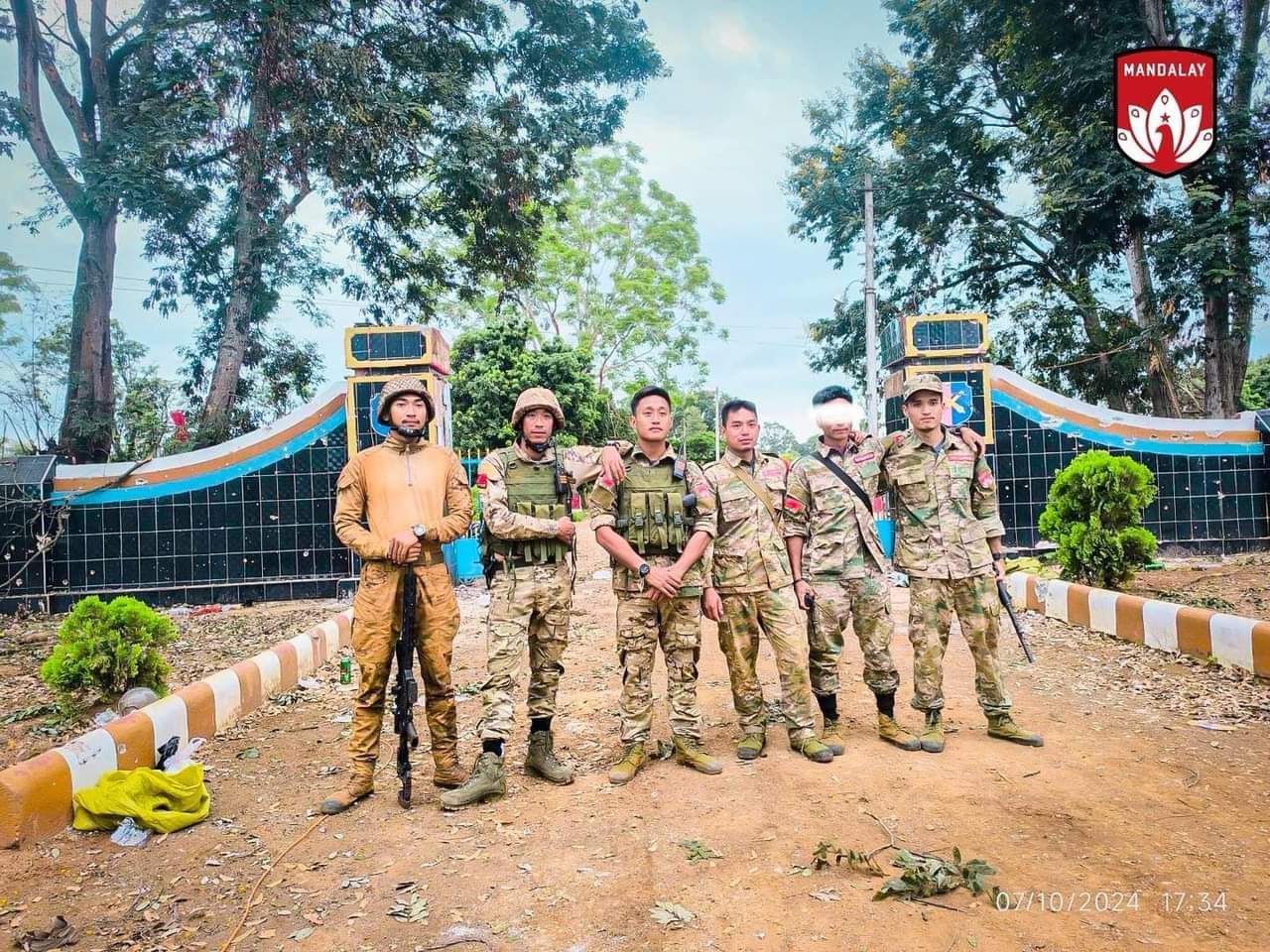
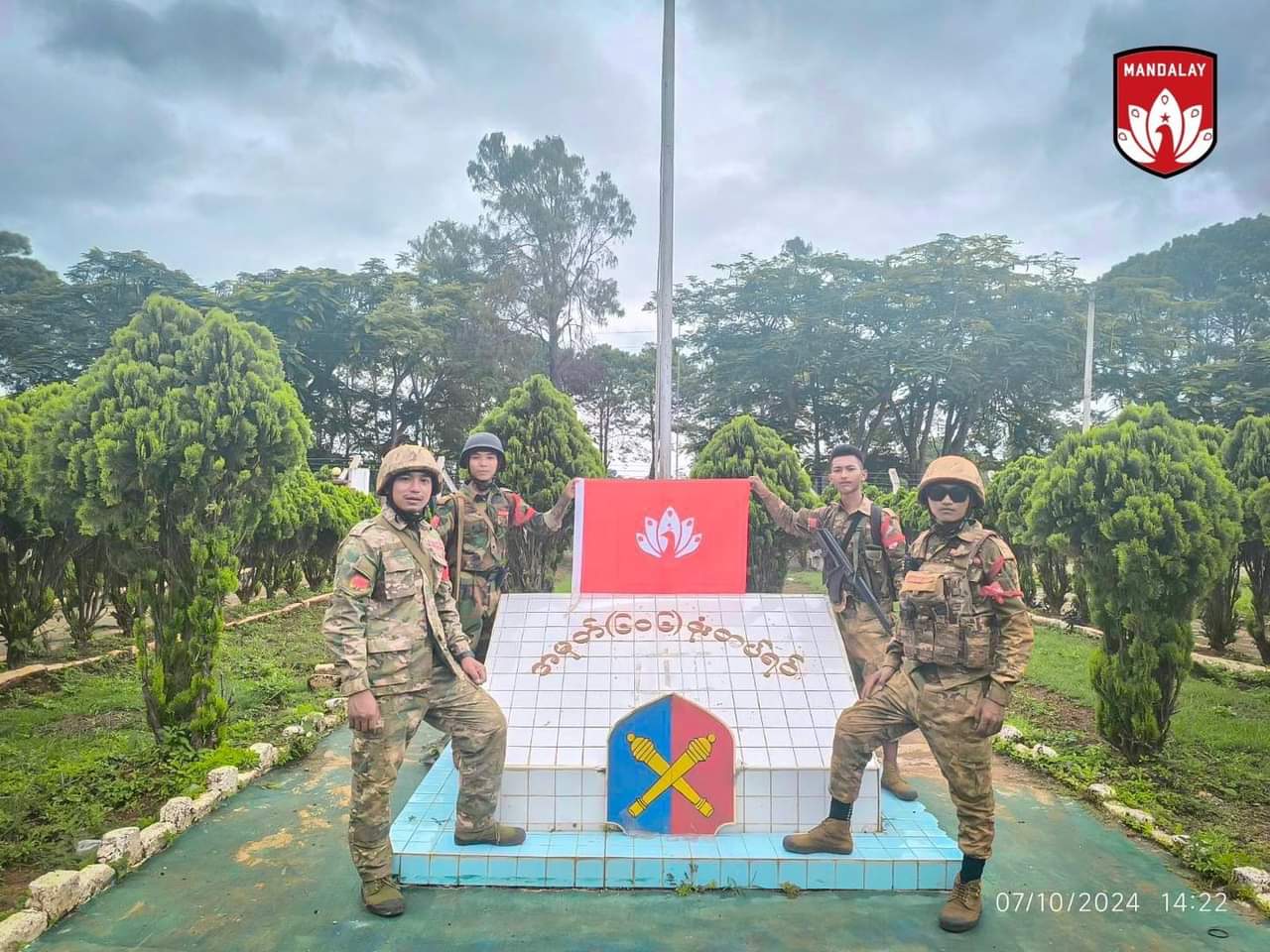
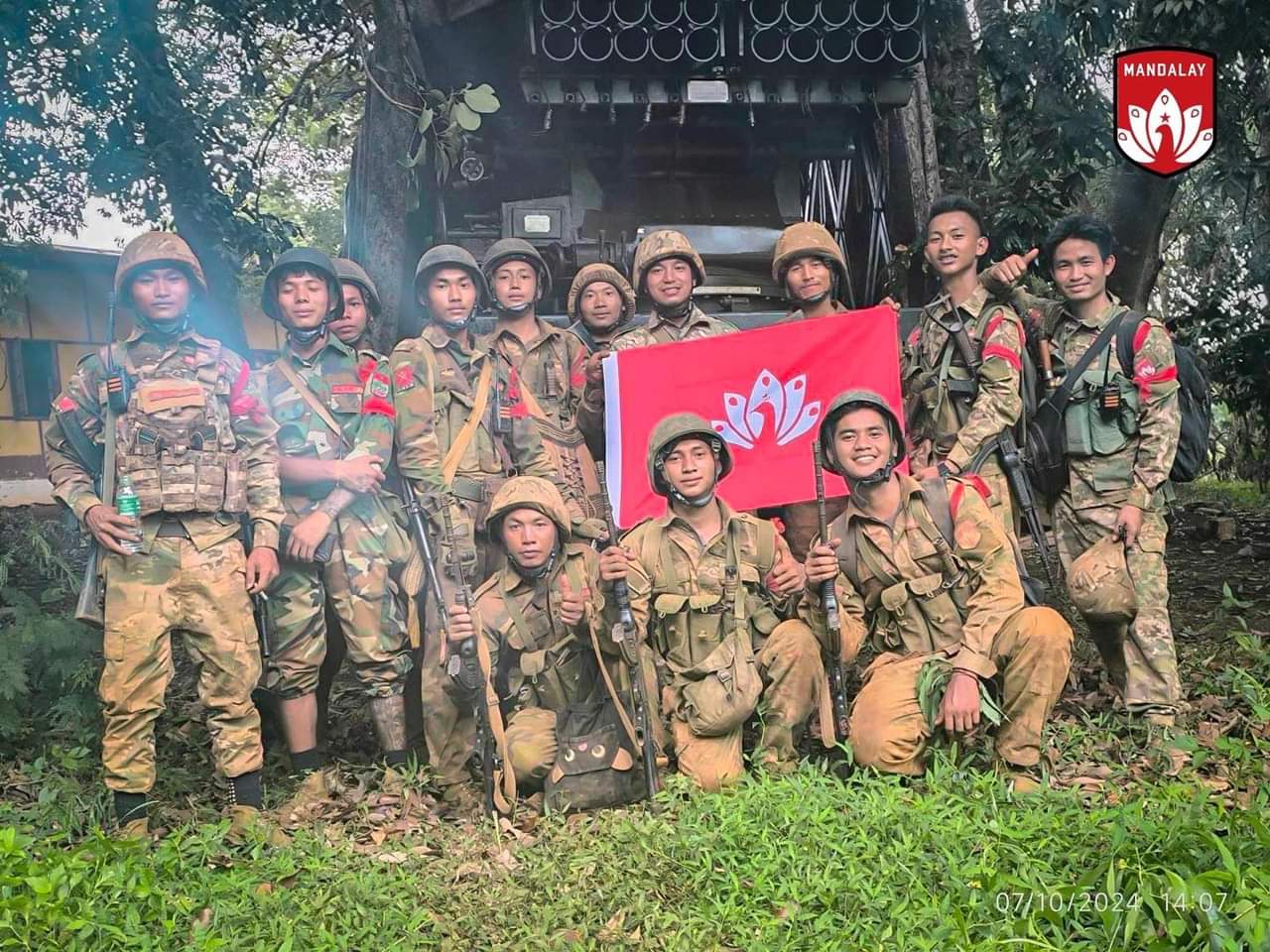
– **Image and Video Generation:** Tools like DALL-E and Stable Diffusion have seen improvements, allowing for high-quality image and video synthesis from textual descriptions, impacting creative industries, marketing, and design.
### 2. **AI Transparency and Explainability**
– **Explainable AI (XAI):** There has been a push towards creating models that are not only powerful but also interpretable. Techniques that help users understand how decisions are made (e.g., SHAP values, LIME) are evolving, promoting trust in AI systems, especially in sensitive areas like healthcare and finance.
### 3. **Multimodal AI**
– **Cross-Modal Learning:** AI systems are becoming better at understanding and integrating multiple types of data (text, images, audio) simultaneously. For example, models that can analyze both images and related text (like CLIP) are being used in nuanced applications such as content moderation and enhanced search capabilities.
### 4. **Reinforcement Learning and Robotics**
– **Advanced RL Techniques:** Reinforcement learning has progressed significantly, leading to more robust algorithms for training AI agents in simulation environments and real-world applications, such as robotics and autonomous systems.
– **Real-World Applications:** Robotics systems are increasingly being equipped with AI to perform complex tasks in unpredictable environments, from healthcare (e.g., surgical robots) to logistics (e.g., automated delivery).
### 5. **AI in Healthcare**
– **Predictive Medicine:** AI algorithms are now being used to predict disease outbreaks and personalize treatment plans based on a patient’s genetic makeup and health history, leading to better patient outcomes.
– **Medical Imaging:** Deep learning techniques are being employed to enhance image analysis for diagnostics, improving accuracy in detecting conditions like cancers or neurological disorders.
### 6. **Natural Language Processing (NLP) Innovations**
– **Conversational AI:** Virtual assistants have become more capable, understanding context better and engaging in more natural and meaningful conversations. The integration of emotion recognition and sentiment analysis has improved their application in customer service and therapy.
– **Language Translation:** Real-time translation apps have improved accuracy and speed, breaking down language barriers in communication and fostering global collaboration.
### 7. **Customizable AI Models**
– **Fine-Tuning Techniques:** Techniques like few-shot learning and transfer learning allow organizations to customize large pre-trained models for specific tasks with minimal data, making AI accessible to smaller companies and niche applications.
– **No-Code/Low-Code Platforms:** Tools that enable users to build AI applications without extensive programming knowledge have been emerging, empowering non-technical users to integrate AI into their workflows.
### 8. **Federated Learning and Privacy-Preserving Techniques**
– **Decentralized Training:** Federated learning allows AI models to be trained across multiple devices without sharing sensitive data, enhancing privacy while still benefiting from collective learning.
– **Differential Privacy:** Techniques to ensure that AI models do not leak personal information during training are being integrated more frequently, particularly in sectors like healthcare and finance.
### 9. **Sustainable AI Practices**
– **Energy-Efficient Algorithms:** With the rising concern over the environmental impact of AI, advancements are being made in developing algorithms that require less computational power and energy during training and inference.
– **AI for Climate Change:** AI is being utilized to model climate patterns, optimize energy consumption, and develop sustainable technologies.
### 10. **Ethics and Governance in AI**
– **Regulatory Developments:** Governments and organizations are focusing on creating regulations and ethical guidelines for the use of AI. Frameworks are being developed to address bias, accountability, and the impact of AI on society.
– **Bias Mitigation Tools:** New tools and techniques are being created to identify and reduce biases in AI models, promoting fairness and inclusivity in AI applications.
### Conclusion
The advancements in AI are wide-ranging and impactful, revolutionizing various industries and applications. Staying informed about these developments is crucial for businesses and practitioners aiming to leverage AI effectively and responsibly. As AI technology continues to evolve, it is likely to bring forth even more innovative solutions and applications that can address complex challenges across sectors.
Leave a Reply