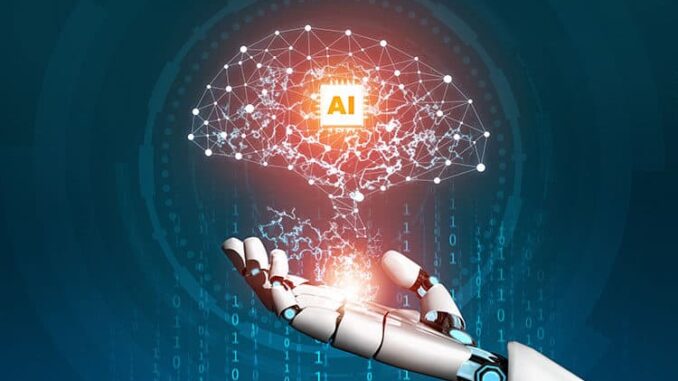
Understanding the AI value chain is critical for organizations looking to successfully implement data-driven transformation.
The AI value chain consists of several key stages that encompass the entire lifecycle of an AI project, from data acquisition to deployment and beyond.
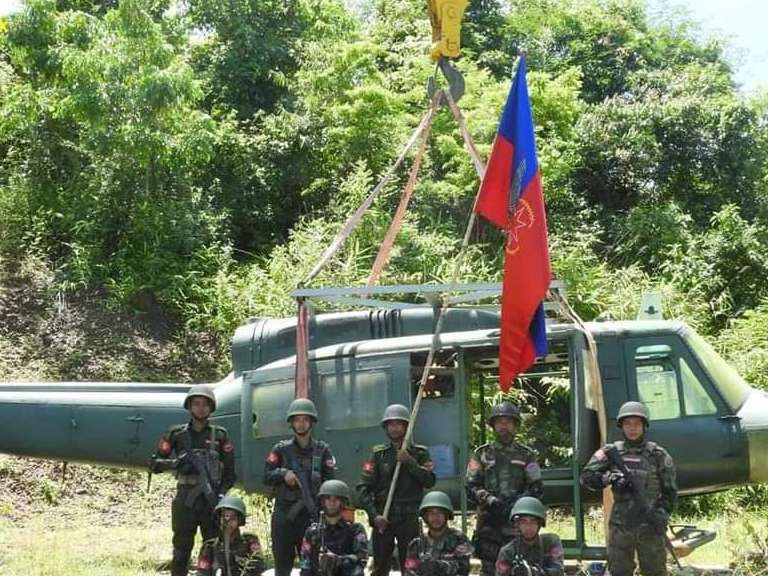
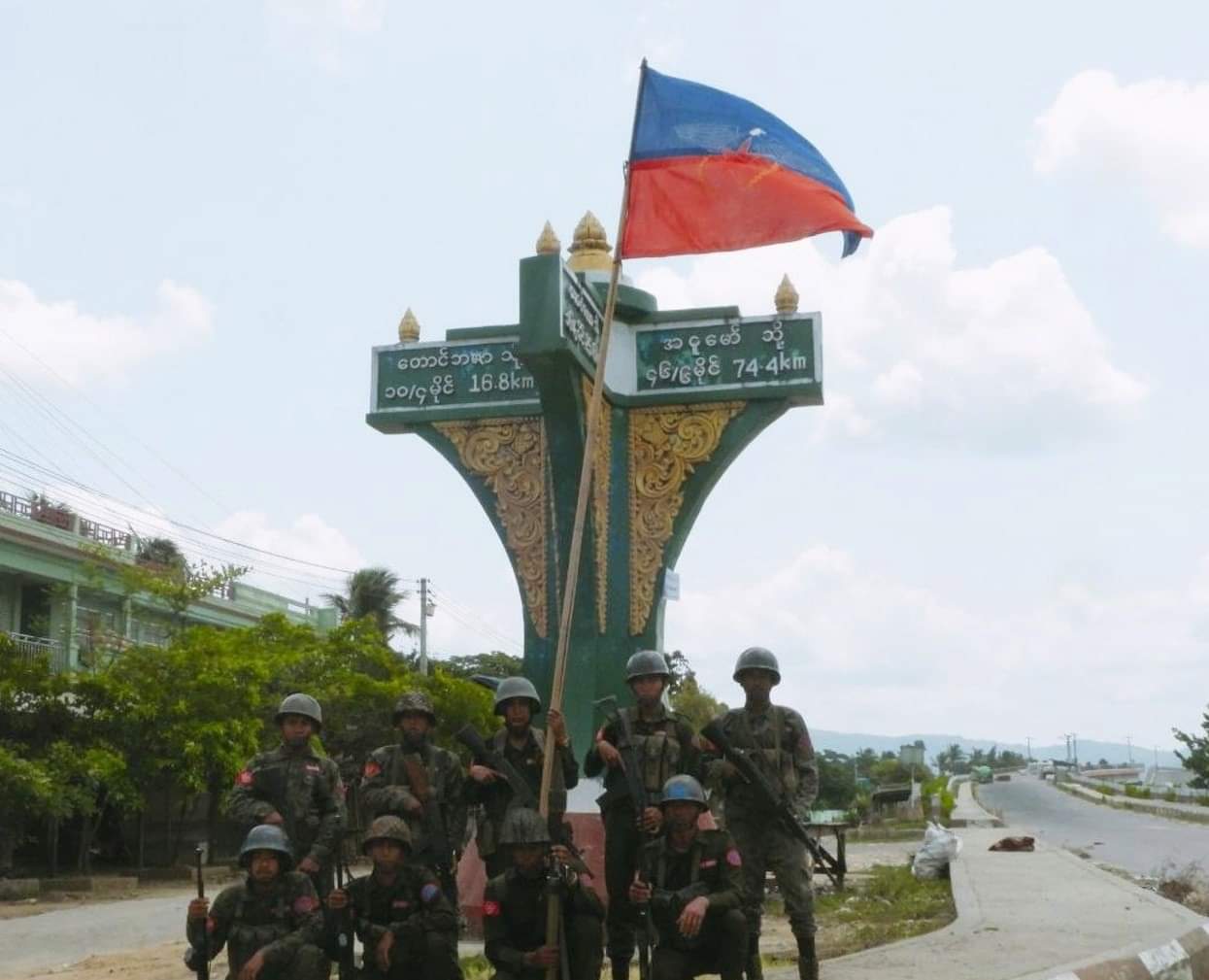
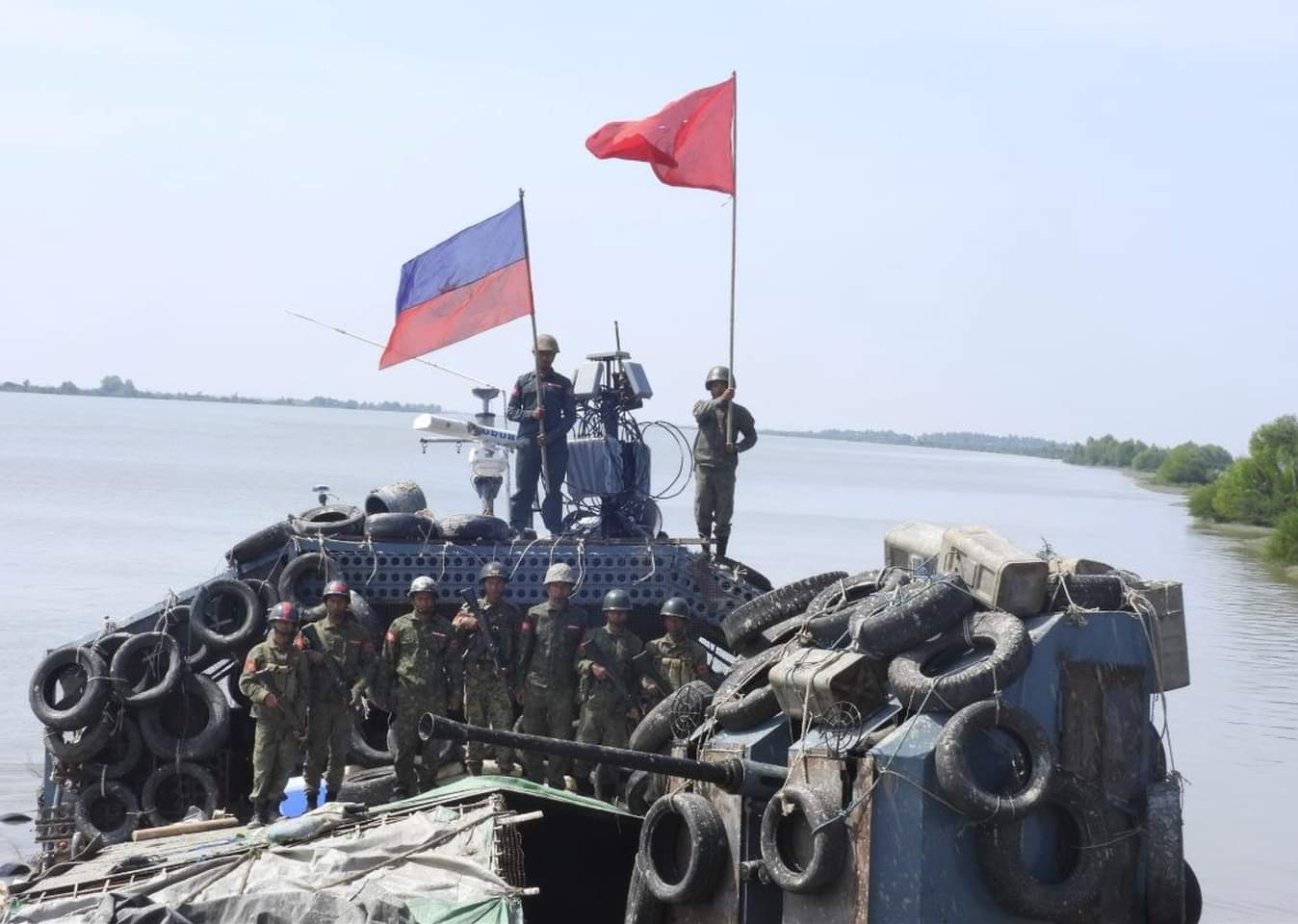
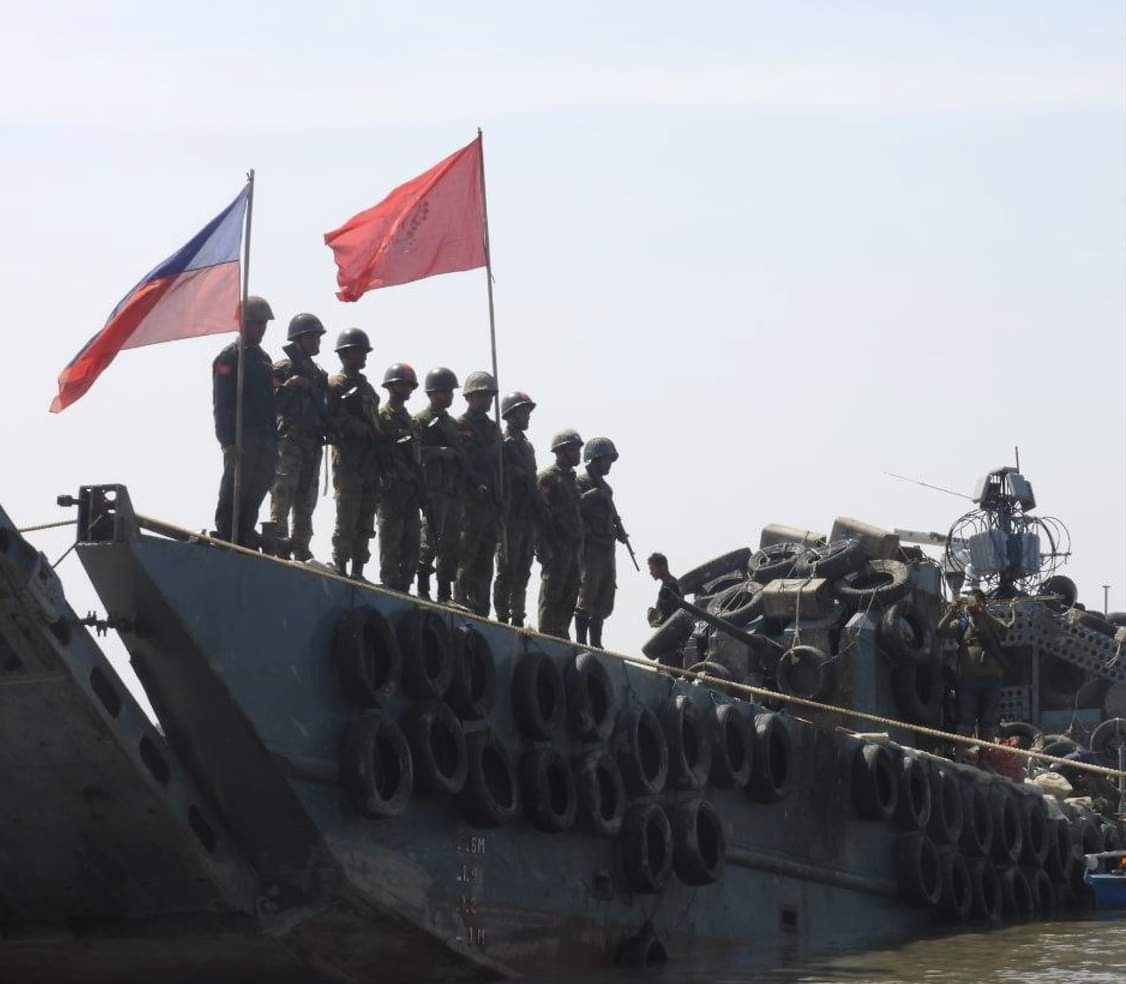
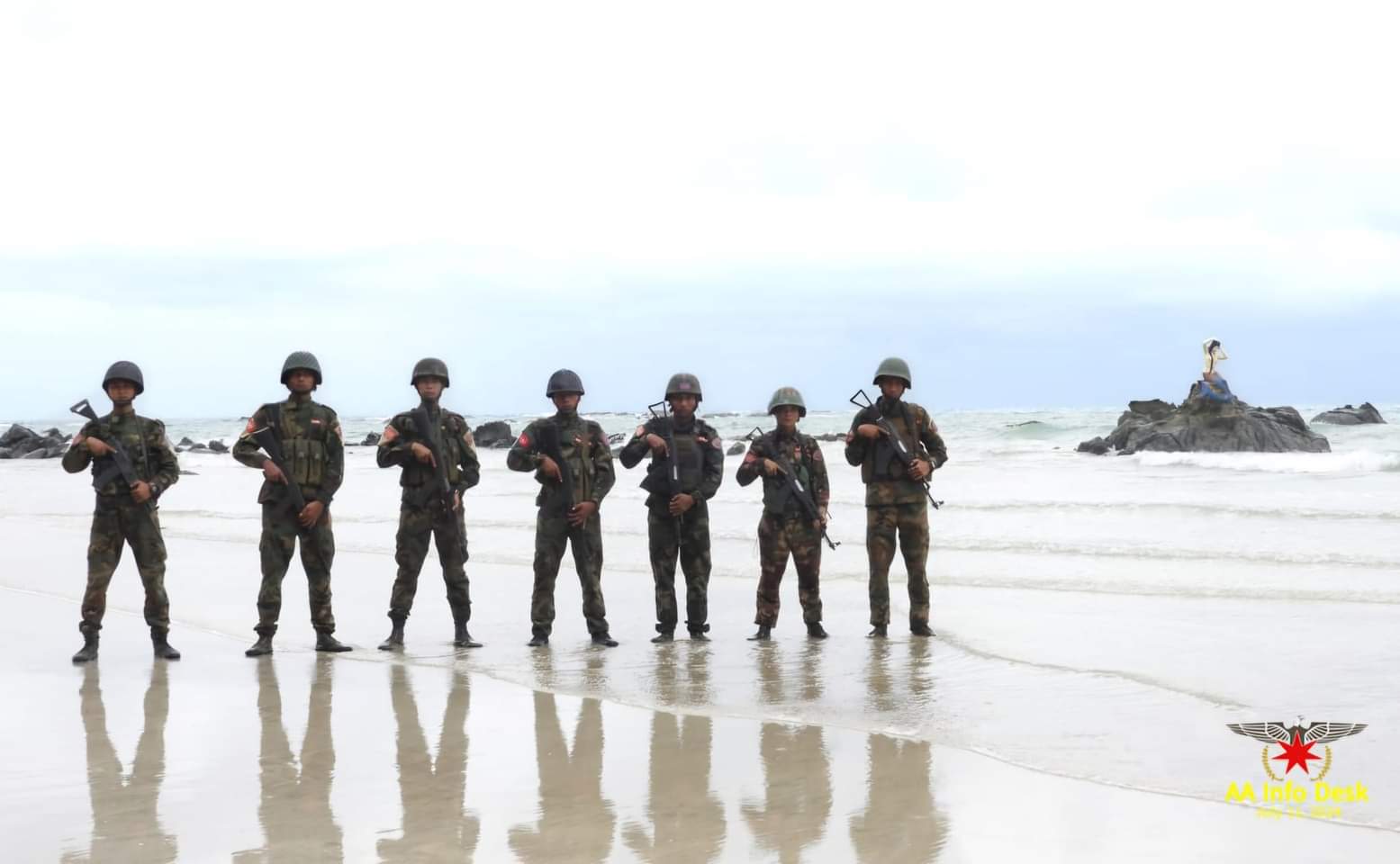
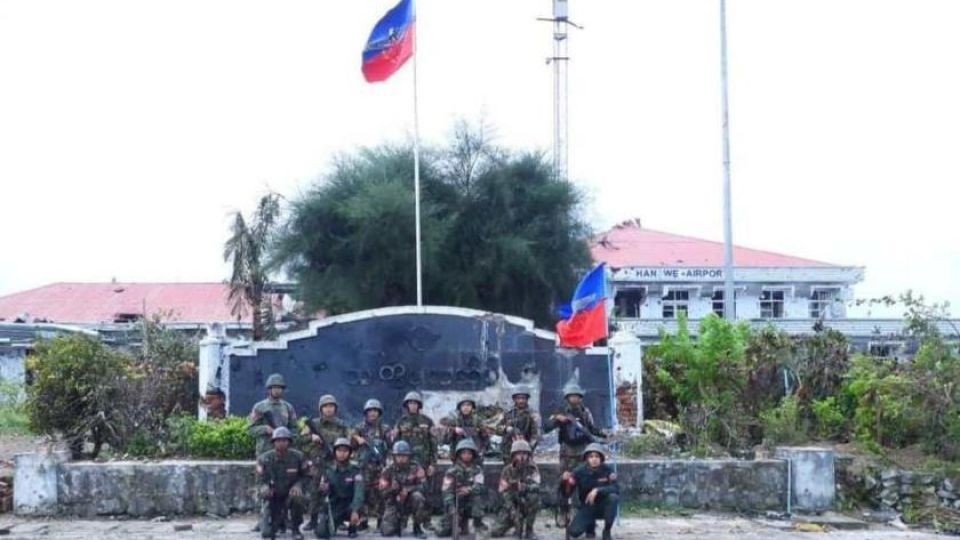
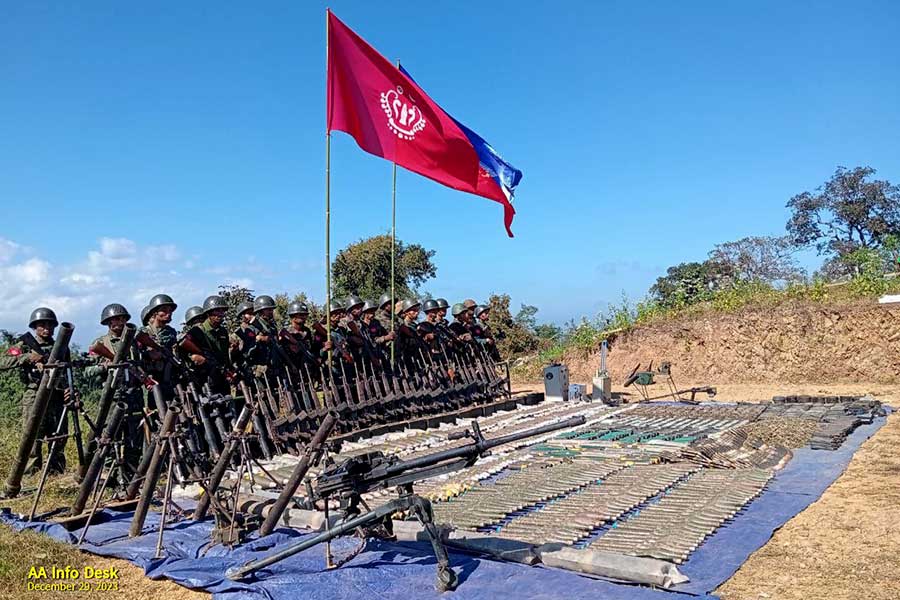
Here’s an overview of these stages and how they contribute to data-driven transformation:
### 1. Data Collection and Acquisition
– **Description**: This stage involves gathering relevant data from various sources such as internal systems, external APIs, IoT devices, and public datasets.
– **Importance**: High-quality, diverse data is the foundation of any AI initiative. Companies need to ensure they collect sufficient amounts of data that are relevant to their goals.
### 2. Data Preparation and Cleaning
– **Description**: Raw data is often messy and unstructured. This stage involves cleaning, transforming, and structuring the data to make it suitable for analysis.
– **Importance**: This step is crucial for improving data quality, addressing missing values, removing duplicates, and ensuring data consistency. Poor data quality can significantly hinder the performance of AI models.
### 3. Data Annotation and Labeling
– **Description**: For supervised learning tasks, data must be annotated and labeled relevantly. This could involve tagging images, categorizing text, or other forms of data labeling.
– **Importance**: Properly labeled data is essential for training AI models and ensuring they can make accurate predictions or classifications.
### 4. Model Development and Training
– **Description**: In this phase, AI models (e.g., machine learning algorithms) are selected and trained using the prepared datasets.
– **Importance**: The selection of the right algorithm and proper tuning of model parameters directly affects model performance. This stage may involve experimentation and iteration to find the best model.
### 5. Model Validation and Testing
– **Description**: After training, models must be validated to ensure they perform accurately on unseen data. Techniques like cross-validation, A/B testing, and performance metrics (e.g., accuracy, precision, recall) are used.
– **Importance**: Validation is necessary to avoid overfitting and to ensure that the model generalizes well to real-world scenarios.
### 6. Deployment
– **Description**: Once validated, models are deployed into production environments, where they can be integrated into applications or systems.
– **Importance**: This stage involves considerations around scalability, reliability, and real-time performance. Successful deployment ensures that AI solutions create value in actual business processes.
### 7. Monitoring and Maintenance
– **Description**: Post-deployment, models need ongoing monitoring to assess their performance and identify drifts in data or changes in the underlying trends.
– **Importance**: Continuous monitoring helps in maintaining the relevance and accuracy of AI models over time. Models may need retraining or adjustment based on new data or changing business requirements.
### 8. Feedback Loop
– **Description**: Incorporating feedback from end-users and performance metrics to improve data collection, model training, and overall process.
– **Importance**: A feedback loop ensures that the AI systems evolve based on user interactions and outcomes, driving continuous improvement.
### Key Considerations for Data-Driven Transformation
– **Governance and Ethics**: Organizations must establish data governance frameworks to ensure compliance with regulations, ethical AI considerations, and responsible data usage.
– **Cultural Change**: Fostering a data-driven culture within the organization, where data is valued and leveraged for decision-making, is vital for success.
– **Skill Development**: Building internal capabilities through training and hiring the right talent ensures that the organization can effectively navigate each stage of the AI value chain.
– **Collaboration**: Working across departments (e.g., IT, operations, marketing) and fostering collaboration between data scientists and business stakeholders can drive alignment and ensure that AI initiatives meet business objectives.
When organizations understand and effectively manage the AI value chain, they can harness the power of data-driven transformation to improve decision-making, optimize operations, and create new business opportunities.
Leave a Reply