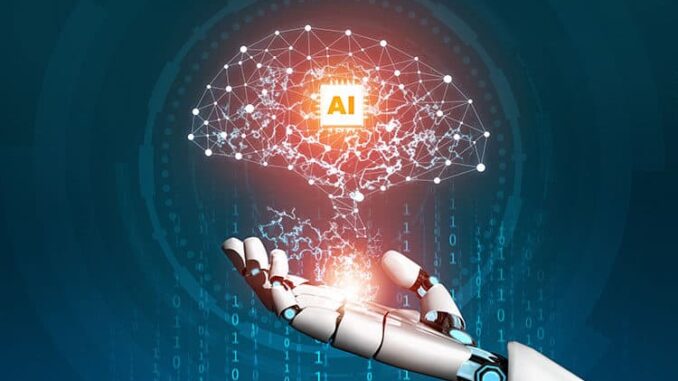
Delivering data-driven transformation solutions using the AI value chain involves strategically employing each component of the value chain to enhance business processes,
drive innovation, and achieve measurable outcomes. Below is a breakdown of how the AI value chain can facilitate data-driven transformation:
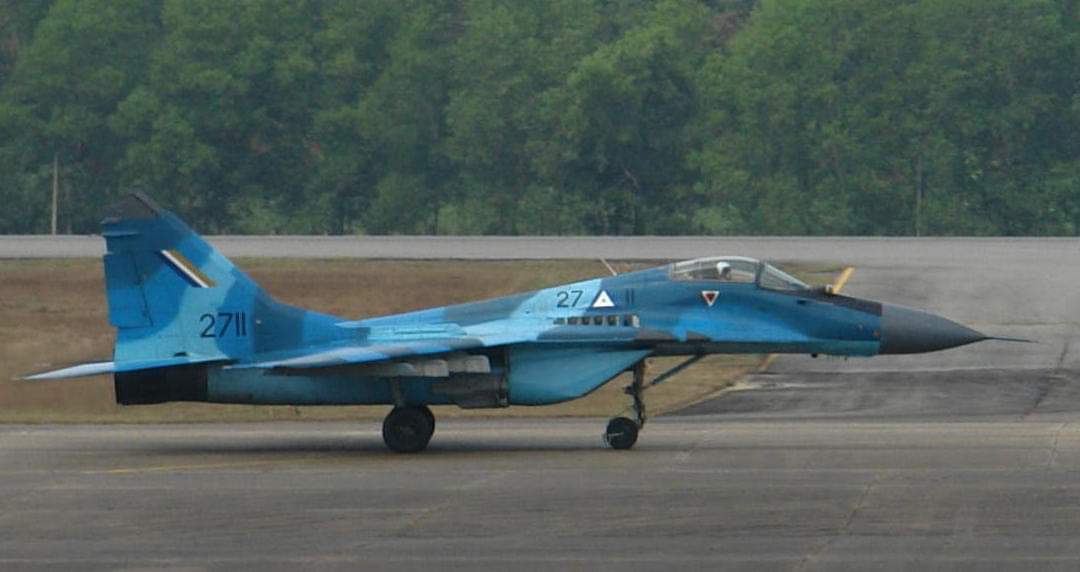
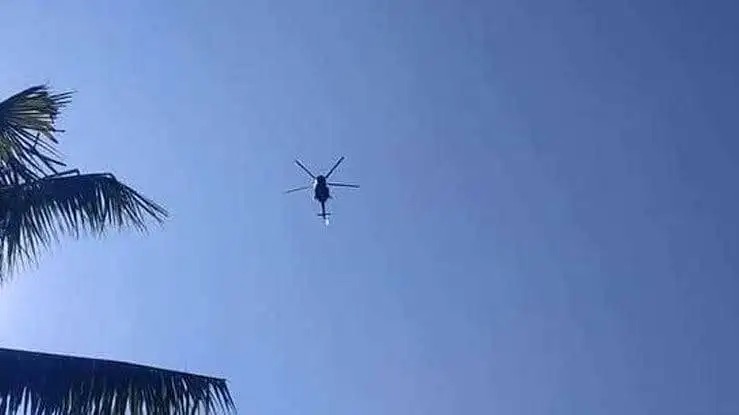
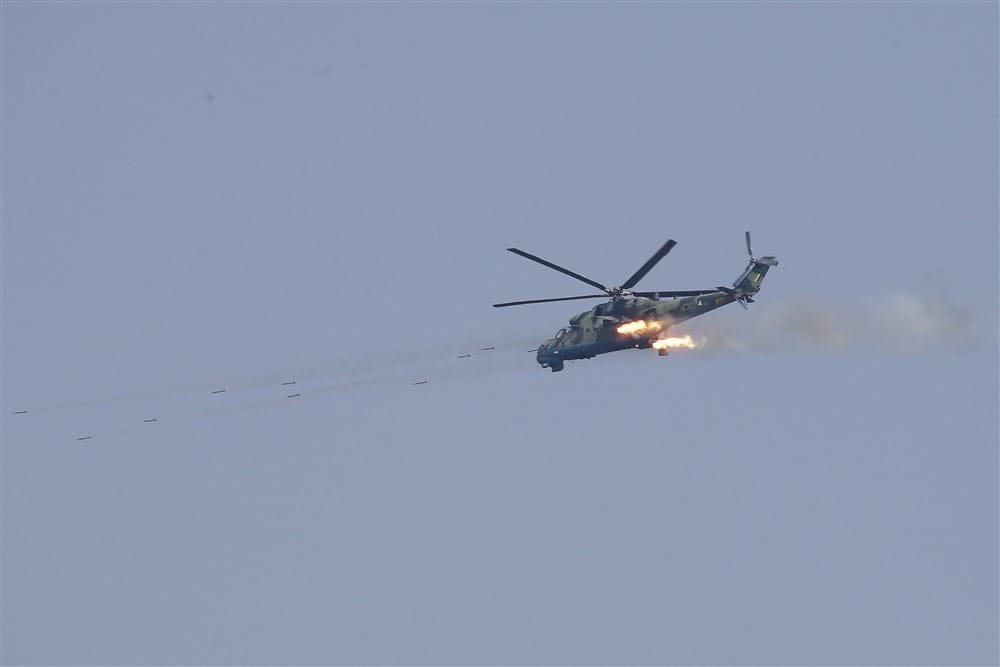
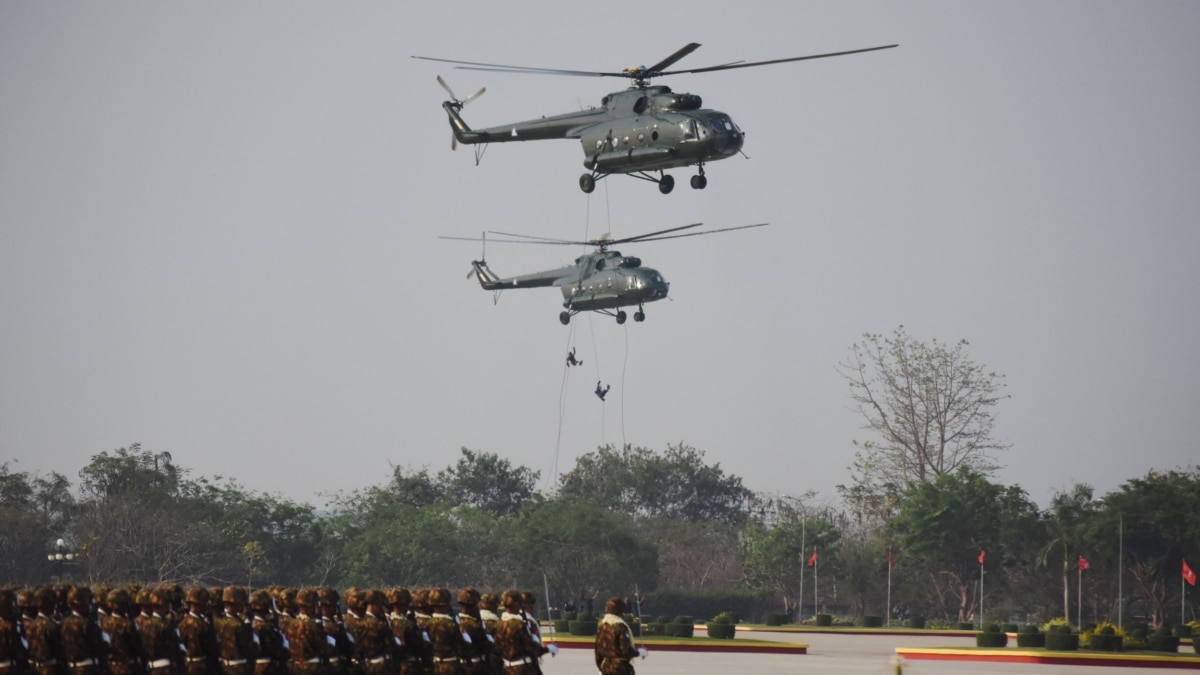
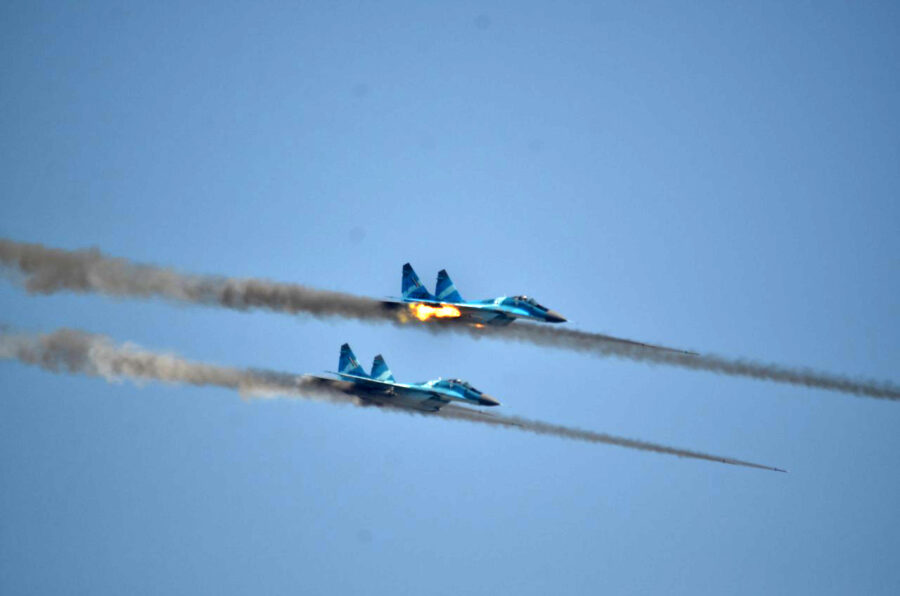
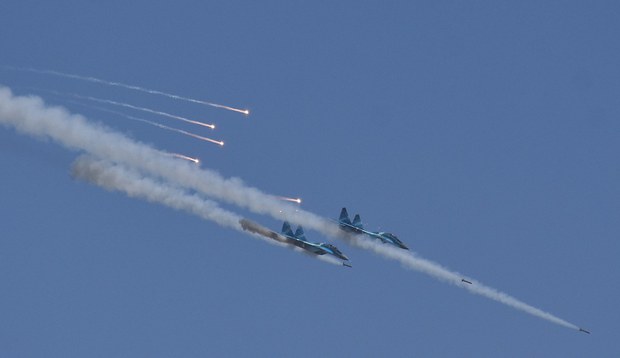
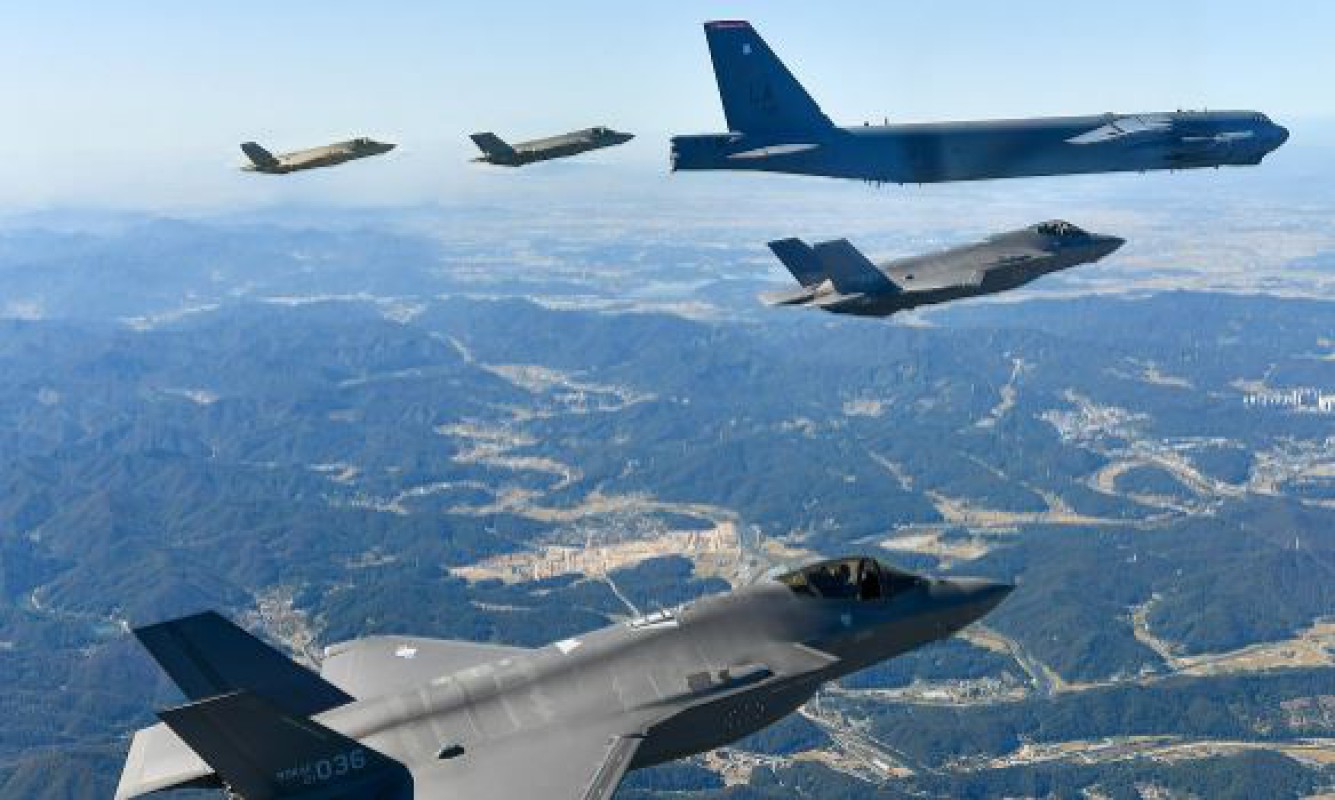
### 1. **Data Collection & Management**
– **Identify Relevant Data Sources:** Analyze existing business processes to identify and aggregate data from various sources, including operational systems, CRM, ERP, social media, and IoT devices.
– **Centralized Data Management:** Implement a data warehouse or data lake to centralize data storage, making it accessible for analysis and reducing silos.
– **Data Quality Assurance:** Establish protocols for ongoing data validation and cleaning to ensure high data quality essential for accurate analysis and model training.
### 2. **Data Processing & Annotation**
– **Automated Data Processing:** Use ETL (Extract, Transform, Load) tools to automate the data processing pipeline, enhancing efficiency and reducing human error.
– **Scalable Annotation Processes:** Leverage both manual and automated methods (e.g., crowdsourcing, AI-assisted labeling) for annotating data, especially for image, text, or complex datasets.
### 3. **Model Development**
– **Use Case Identification:** Collaborate with business stakeholders to identify specific use cases where AI can add value (e.g., predictive maintenance, customer segmentation, fraud detection).
– **Rapid Prototyping:** Employ agile methodologies to quickly develop prototypes and iterate based on feedback, allowing for flexibility in model development.
– **Diverse Algorithm Selection:** Experiment with a range of algorithms and techniques to find the best fit for each specific problem, ensuring robust solutions.
### 4. **Model Deployment**
– **Seamless Integration:** Ensure that AI solutions are integrated into existing business processes and technology stacks to provide real-time insights and recommendations where needed.
– **Cloud or Edge Deployment:** Decide on the deployment strategy based on latency and scalability requirements—cloud services can offer scalability, while edge computing may be necessary for real-time operations and IoT devices.
### 5. **Decision Making & Insights**
– **Actionable Insights:** Transform model outputs into actionable insights, providing managers and decision-makers with clear recommendations based on data analysis.
– **Visualization Tools:** Use data visualization tools (e.g., dashboards and BI tools) to provide stakeholders with clear, understandable representations of analytics and performance metrics.
### 6. **Feedback Loop**
– **Establish Continuous Learning:** Develop processes for continuous feedback from the use of AI solutions, allowing models to adapt over time with ongoing data and user interactions.
– **Performance Monitoring:** Implement real-time monitoring systems to track model performance and business outcomes, enabling quick adjustments as necessary.
### 7. **Value Realization**
– **Set Clear KPIs:** Define specific metrics and KPIs to measure the impact of AI initiatives on business goals, such as increased efficiency, reduced costs, enhanced customer experiences, or new revenue streams.
– **ROI Assessment:** Conduct regular evaluations to assess the return on investment of AI initiatives, ensuring alignment with broader business objectives and identifying areas for further investment.
### 8. **Ethics & Compliance**
– **Framework for Ethical AI:** Develop a framework to guide ethical considerations in AI applications, ensuring fairness, accountability, and equity in AI decision-making processes.
– **Regulatory Compliance:** Stay updated with regulations in the regions where the organization operates and ensure compliance, particularly regarding data protection and ethical AI use.
### Conclusion
By following the AI value chain, organizations can effectively implement data-driven transformation solutions that enhance decision-making, drive innovation, and lead to more efficient operations. This structured approach enables businesses to exploit their data assets fully, harnessing AI’s capabilities to remain competitive in an increasingly data-centric world. Successful implementation not only improves operational outcomes but can also foster a culture of innovation and continuous improvement.
Leave a Reply