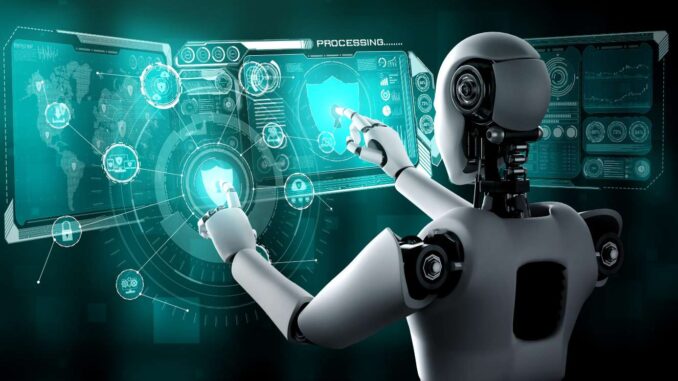
Machine Learning (ML) and Artificial Intelligence (AI) are closely related fields that involve the development of algorithms and systems that allow computers to perform tasks that typically require human intelligence.
1. Definitions: Artificial Intelligence (AI): Refers to the simulation of human intelligence in machines that are programmed to think and learn like humans.
AI encompasses a variety of technologies, including ML, natural language processing, robotics, and more.
Machine Learning (ML): A subset of AI that focuses on building systems that learn from and make predictions or decisions based on data. ML algorithms improve their performance as they are exposed to more data over time.
2. Types of Machine Learning:
Supervised Learning: The model is trained on labeled data, meaning that the input data is paired with the correct output. Common algorithms include regression analysis, decision trees, and support vector machines.
Unsupervised Learning: The model is trained on data without explicit labels. It seeks to find patterns or groupings in the data. Examples include clustering algorithms like k-means and hierarchical clustering.
Reinforcement Learning: The model learns by interacting with an environment and receiving rewards or penalties for its actions. It is often used in scenarios like game playing and robotics.
3. Applications:
Natural Language Processing (NLP): Used in applications like chatbots, translation services, and sentiment analysis.
Computer Vision: Involved in facial recognition, object detection, and image classification.
Recommendation Systems: Found in platforms like Netflix and Amazon, recommending content based on user behavior.
Autonomous Vehicles: Implementing AI for navigation, obstacle detection, and decision-making.
4. Challenges:
Data Quality: The performance of ML models heavily depends on the quality and quantity of data.
Bias and Fairness: AI models can perpetuate or even exacerbate biases present in training data.
Interpretability: Many ML models, especially deep learning models, operate as “black boxes,” making it difficult to understand their decision-making process.
Ethics: Issues around privacy, surveillance, and the socio-economic impact of AI technologies are significant concerns.
5. Future Trends:
Explainable AI (XAI): Focuses on creating models that can provide understandable explanations for their predictions or decisions.
Integration of AI with IoT: Connecting AI with Internet of Things (IoT) devices for smarter applications.
Generative AI: Technologies like GANs (Generative Adversarial Networks) and language models (e.g., GPT) that can create new content, whether it’s images, text, or music.
AI in Healthcare: Leveraging ML for diagnostic tools, personalized medicine, and drug discovery.
Machine Learning and AI are rapidly evolving fields with profound implications across many domains. With advancements in technology and continued research, their capabilities will likely expand even further, shaping the future of work, communication, and daily life. If you have specific questions or topics within ML and AI you’d like to explore further, feel free to ask!
Leave a Reply