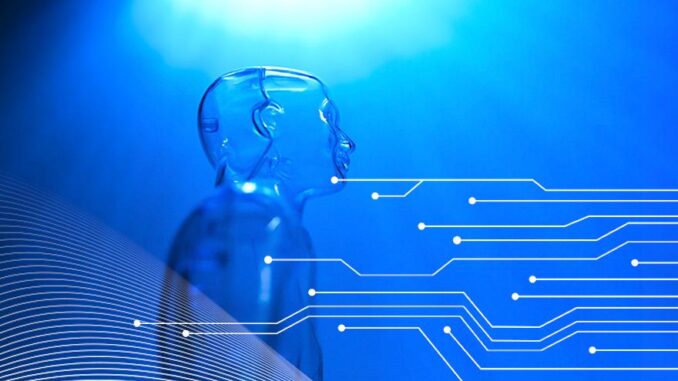
To ensure that AI investments deliver ongoing value, organizations need to adopt a strategic approach that encompasses several key practices and considerations. Below are some of the methods and strategies that contribute to sustained value from AI investments:
– Clear Use Cases: Focus on specific, well-defined use cases that align with overall business goals. Prioritize AI projects that target critical pain points or opportunities for improvement.

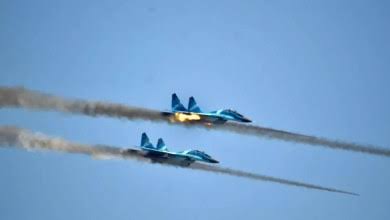
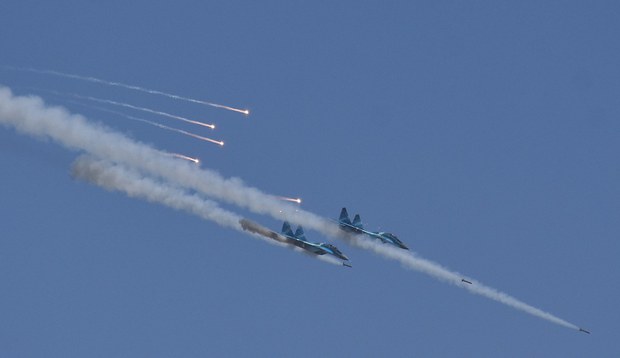
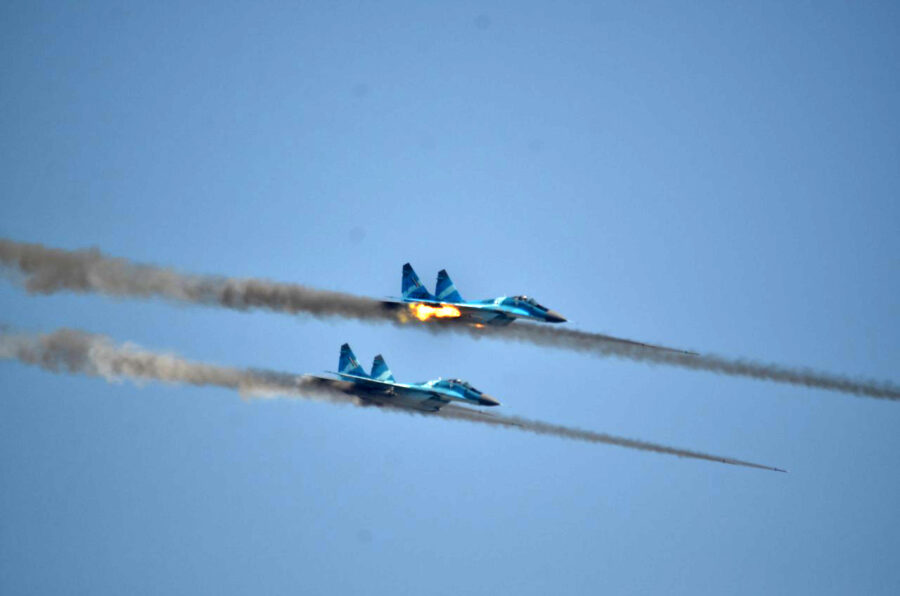
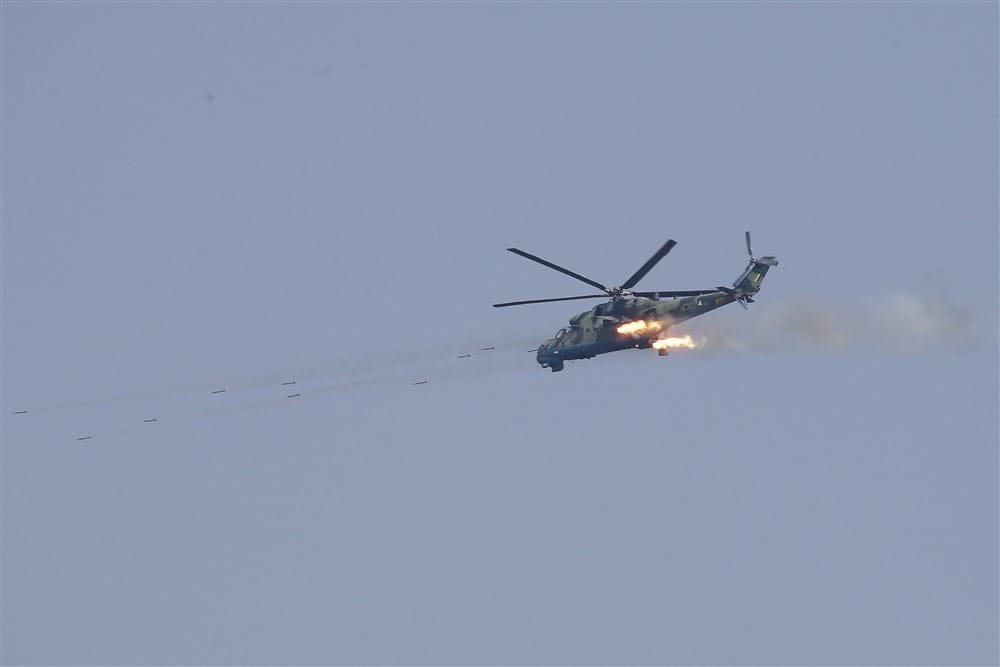
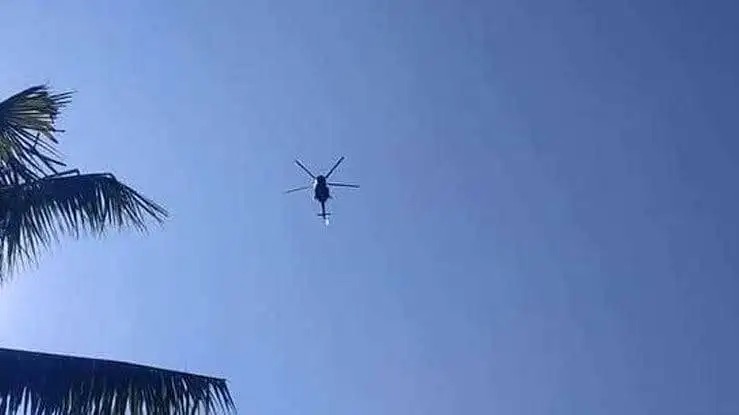
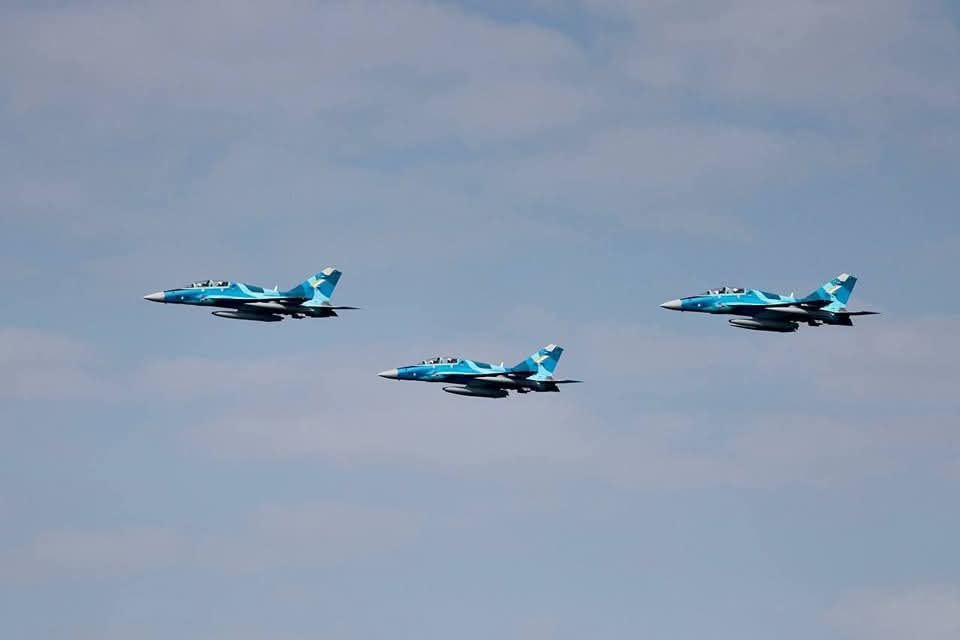
– **Regular Review**: Conduct periodic reviews to assess how AI initiatives are contributing to strategic goals and adjust them as necessary to maintain relevance.
### 2. **Change Management and Culture**
– **Foster an Innovative Culture**: Encourage a culture of experimentation and innovation among employees. This involves promoting openness to AI technologies and how they can enhance workflows.
– **Stakeholder Engagement**: Engage stakeholders at all levels (from executives to end-users) in the development and deployment of AI solutions to ensure buy-in and reduce resistance to change.
### 3. **Robust Data Governance**
– **Quality Data Management**: Invest in processes that ensure high data quality, accuracy, and relevance. Clean, high-quality data is essential for effective AI performance.
– **Ethical Data Practices**: Establish guidelines for ethical data collection and usage, including compliance with privacy regulations, to build trust and maintain compliance.
### 4. **Scalable Infrastructure**
– **Cloud Solutions**: Utilize cloud-based platforms to scale AI capabilities as needed, enabling rapid deployment of models and access to computing resources.
– **Modular Architecture**: Design AI systems with a modular architecture that allows for easy upgrades and integration with existing technologies, facilitating future enhancements.
### 5. **Performance Measurement and Feedback Loops**
– **Real-Time KPIs**: Implement and track key performance indicators in real-time to assess AI performance and impact on business processes.
– **Feedback Mechanisms**: Establish mechanisms for gathering user feedback and experiences to inform iterative improvements in AI systems.
### 6. **Continuous Learning and Adaptation**
– **Model Retraining**: Regularly retrain AI models with new data to improve accuracy and relevance, ensuring that they adapt to changing conditions and trends.
– **Stay Updated with Trends**: Monitor advancements in AI technologies and methodologies to implement cutting-edge solutions that can enhance performance.
### 7. **Integration with Business Processes**
– **Seamless Integration**: Ensure AI systems are integrated with existing business processes and tools to maximize efficiency and minimize disruption.
– **Automation of Workflows**: Leverage AI to automate repetitive tasks, allowing employees to focus on higher-value activities, thus maximizing productivity.
### 8. **Cross-Functional Collaboration**
– **Interdisciplinary Teams**: Create cross-functional teams that combine expertise from data science, engineering, and business operations to drive more effective AI development and implementation.
– **Knowledge Sharing**: Promote knowledge sharing among teams to disseminate insights and best practices related to AI applications.
### 9. **User-Centric Design**
– **User-Friendly Applications**: Design AI systems with the end-user in mind, ensuring that interfaces are intuitive and accessible to facilitate adoption and usability.
– **Training Programs**: Provide ongoing training and support to users, helping them understand how to best leverage AI tools in their day-to-day tasks.
### 10. **Proactive Maintenance and Support**
– **Regular System Audits**: Implement periodic audits of AI systems to identify areas for improvement and ensure they are functioning as intended.
– **Support Infrastructure**: Develop a strong support framework to address technical issues and user inquiries promptly, maintaining high levels of user satisfaction.
### 11. **Economic Justification**
– **ROI Assessment**: Regularly assess the return on investment (ROI) of AI initiatives to quantify their economic impact, justifying ongoing and future investments.
– **Cost-Benefit Analysis**: Periodically perform cost-benefit analyses to evaluate the financial viability of AI projects and reallocate resources as needed.
### 12. **Establish Partnerships**
– **Collaborate with Experts**: Form partnerships with AI experts or academic institutions to access cutting-edge research and techniques that can enhance AI capabilities.
– **Engage with AI Communities**: Participate in AI forums and communities to share experiences and learn from peers in the industry, tapping into a broader pool of knowledge.
By implementing these strategies, organizations can ensure that their investments in AI deliver ongoing value, driving performance improvements, operational efficiency, and enhanced customer experiences. This holistic approach not only maximizes the returns on AI investments but also positions the organization to adapt to evolving market dynamics and technological advancements.
Leave a Reply