
The effectiveness of AI deployments can vary widely based on several factors, including industry, application, data quality, organizational readiness, and the specific AI technologies used. Here are some key aspects that influence the effectiveness of AI deployments:
1. Clear Objectives and Use Cases – Problem Definition: Successful AI deployments start with clearly defined goals and specific use cases that address real business problems. Organizations need to assess whether AI is the right tool for the job.
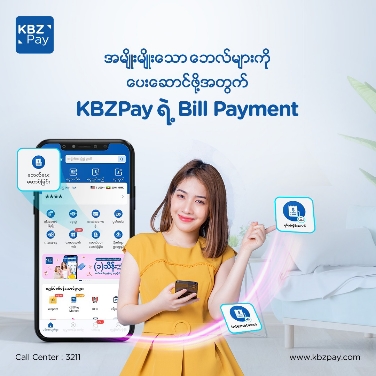
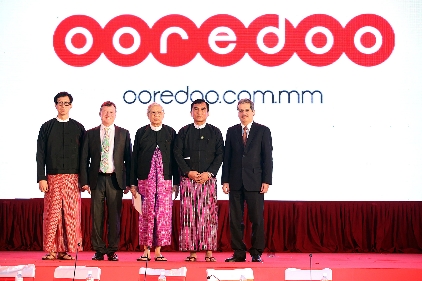
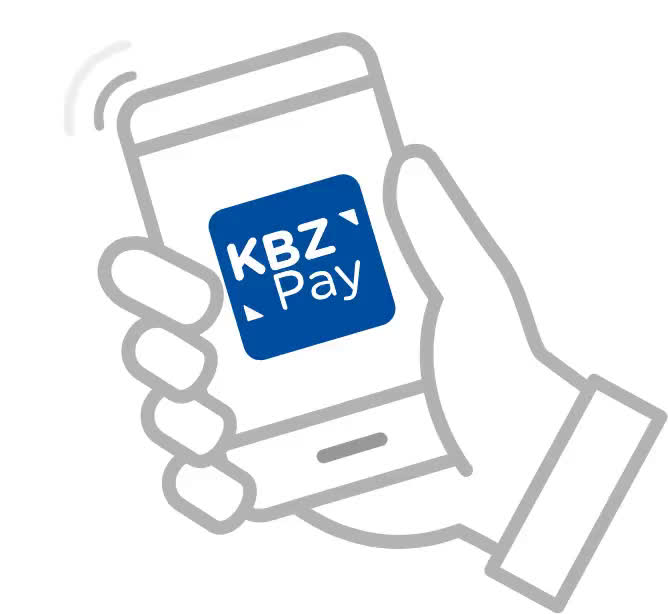
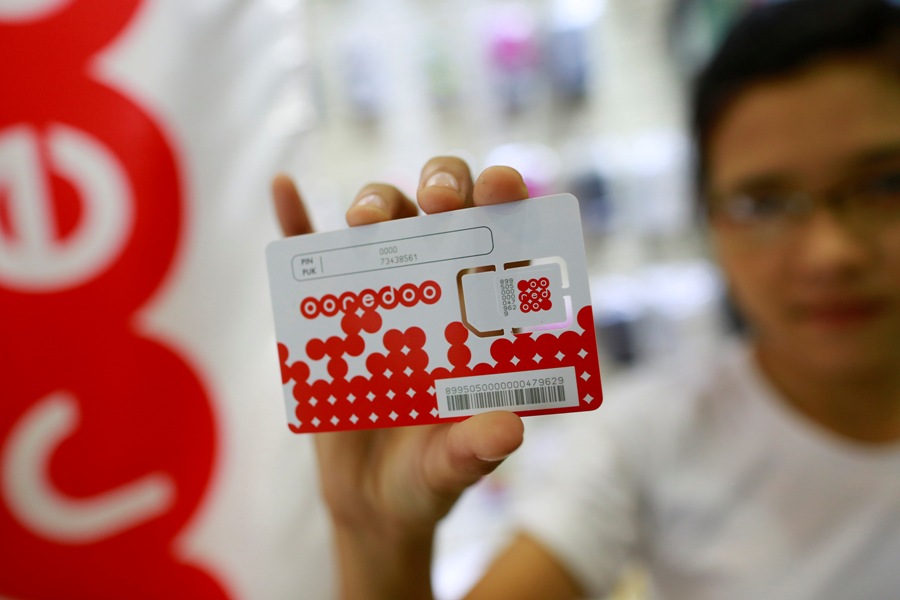
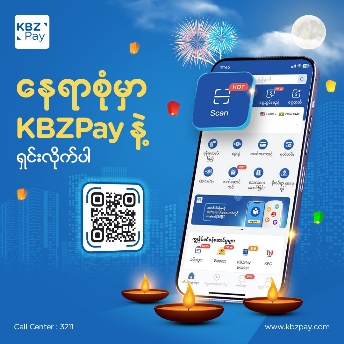
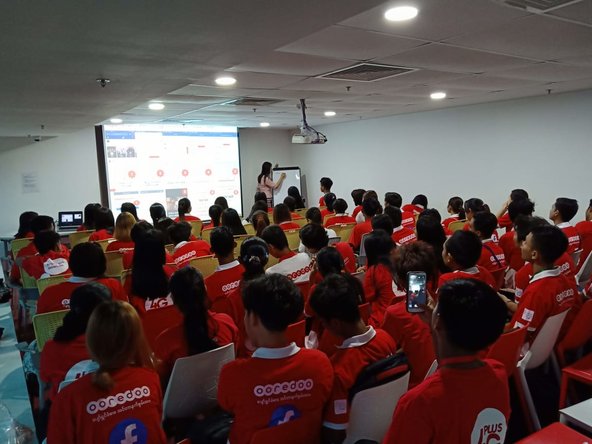
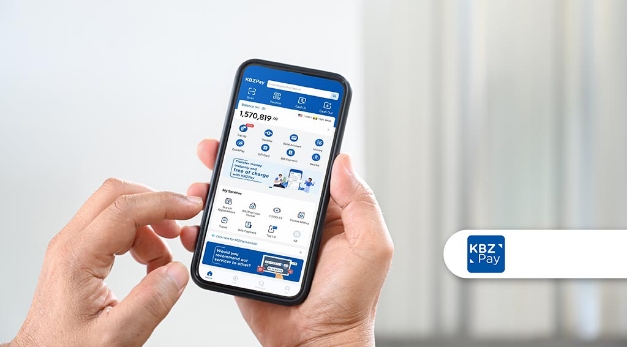
– **Alignment with Business Goals:** The AI solution should align with the overarching business strategies and objectives.
### 2. **Data Quality and Management**
– **High-Quality Data:** Effective AI systems require high-quality, comprehensive data. Poor data can lead to inaccurate models and decision-making.
– **Data Governance:** Organizations need strong data management practices to ensure the reliability and security of their data.
### 3. **Technological Infrastructure**
– **Scalable Infrastructure:** Deploying AI solutions often requires robust infrastructure, including computational resources and cloud services that can handle large volumes of data.
– **Integration with Existing Systems:** AI should seamlessly integrate with existing technologies and workflows within the organization.
### 4. **Skillset and Talent**
– **Expertise:** Effective deployment often requires skilled personnel, including data scientists, AI specialists, and domain experts who understand both the technology and the industry context.
– **Training and Development:** Organizations need to invest in training their workforce to understand and leverage AI technologies effectively.
### 5. **Collaboration Across Teams**
– **Cross-Functional Teams:** Collaboration between different departments—such as IT, operations, and business units—can enhance AI effectiveness by ensuring that various perspectives are considered.
– **Feedback Loops:** Incorporating feedback from end users can improve AI models and their applications over time.
### 6. **Change Management**
– **Cultural Shift:** Successful AI deployment often requires a cultural shift within the organization, fostering an environment that embraces innovation and change.
– **User Adoption:** Ensuring that users understand and trust the AI systems is critical for widespread adoption and effective use.
### 7. **Monitoring and Evaluation**
– **Performance Metrics:** Organizations should define and measure specific KPIs to evaluate the performance and impact of AI deployments.
– **Continuous Improvement:** Regularly updating and refining AI models based on performance data and evolving business needs can enhance effectiveness over time.
### 8. **Ethical Considerations**
– **Ethical AI:** Organizations must consider ethical implications, including bias, fairness, and transparency, to build trust and ensure compliance with regulations.
### 9. **Industry Context**
– **Sector-Specific Challenges:** Different industries (healthcare, finance, retail, etc.) face unique challenges and opportunities in AI deployment, influencing the approach and success rates.
### 10. **Regulatory Compliance**
– **Adhering to Regulations:** Compliance with laws and regulations, especially regarding data privacy and security, is essential to avoid legal repercussions and build customer trust.
### Conclusion
The effectiveness of AI deployments largely depends on how well organizations navigate these factors. Organizations that take a strategic, informed approach while remaining flexible and adaptive are more likely to achieve successful AI implementations that provide meaningful business value.
Leave a Reply