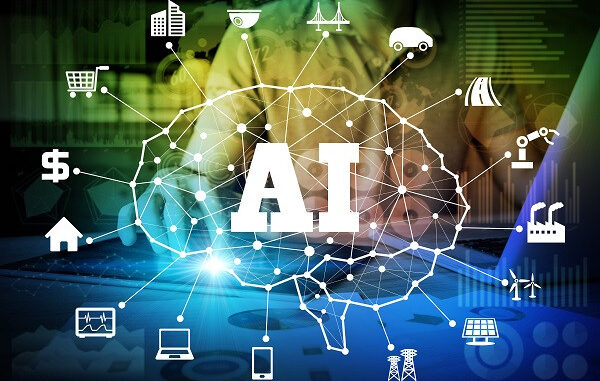
The term “pivotal role” in the context of AI projects refers to the critical importance of certain elements that can greatly influence the success or effectiveness of the project. Here’s a detailed overview of various aspects that play a pivotal role in AI projects:
### 1. **Data Quality and Quantity** – **Role:** The quality and quantity of data are fundamental to any AI project. Good models can only be built upon high-quality, diverse datasets.

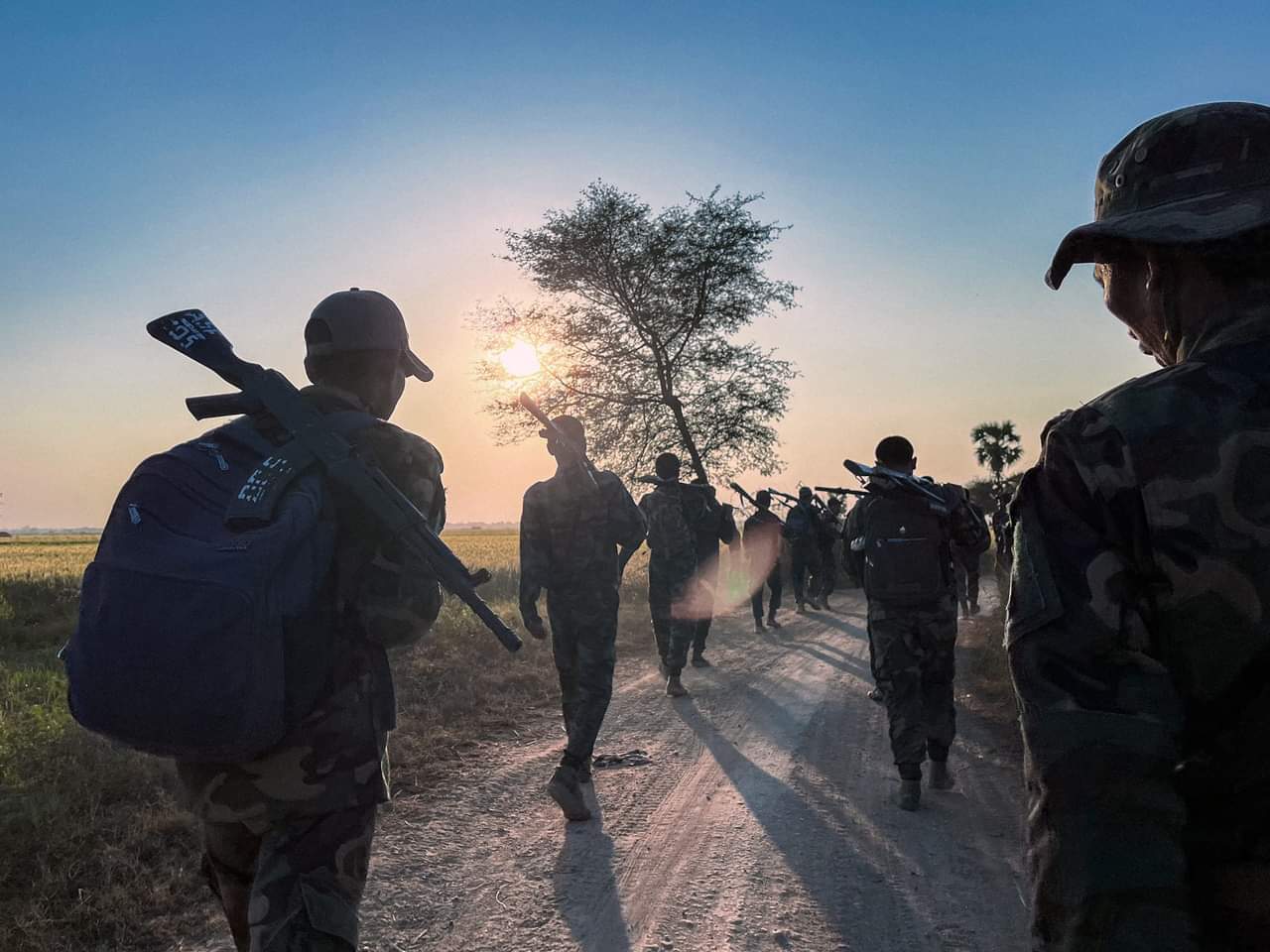
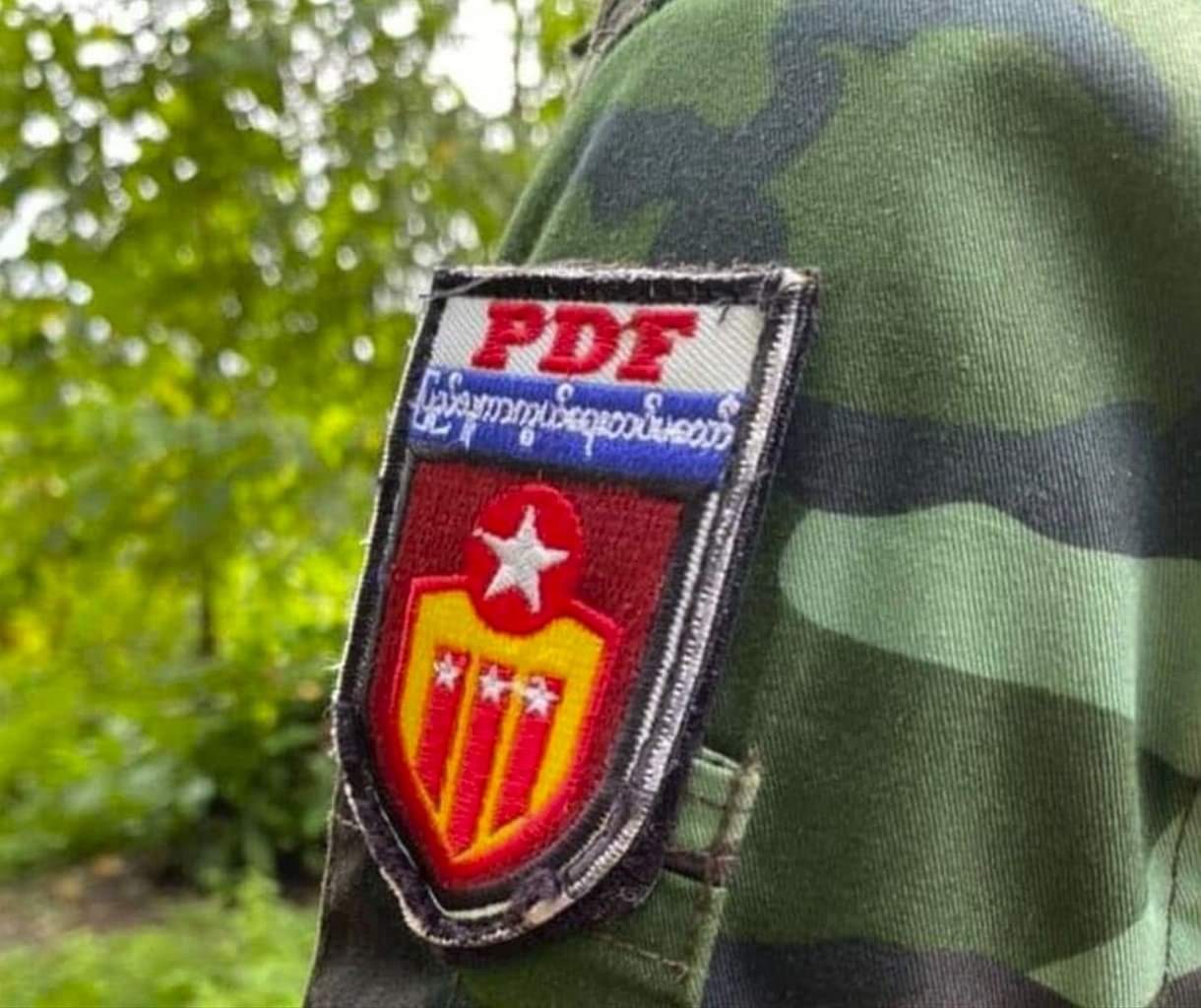
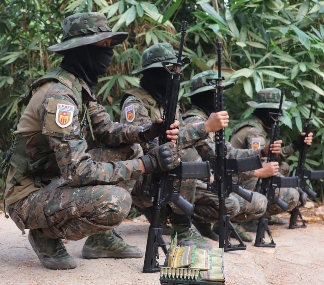
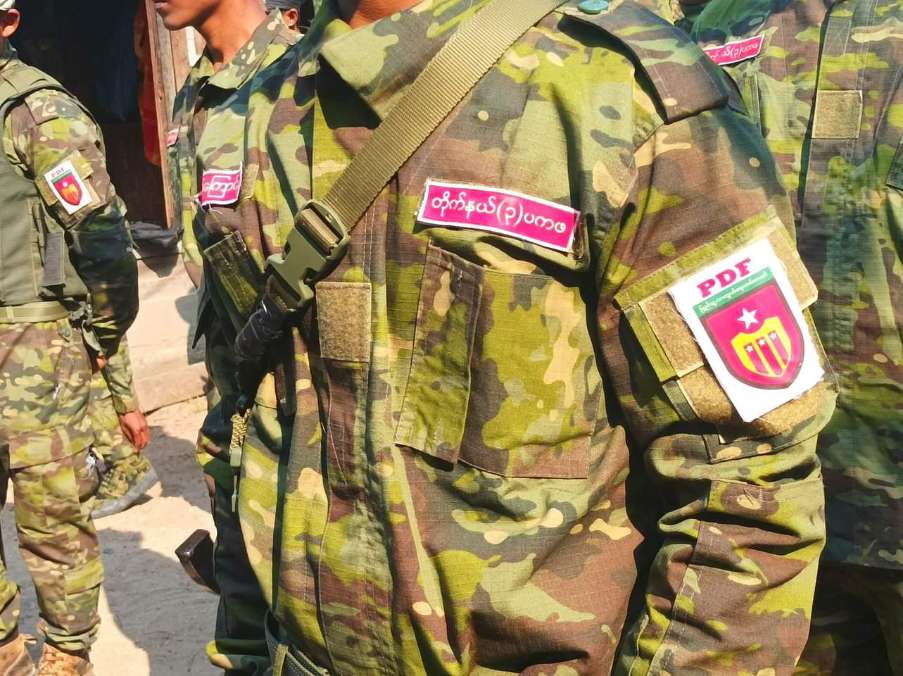
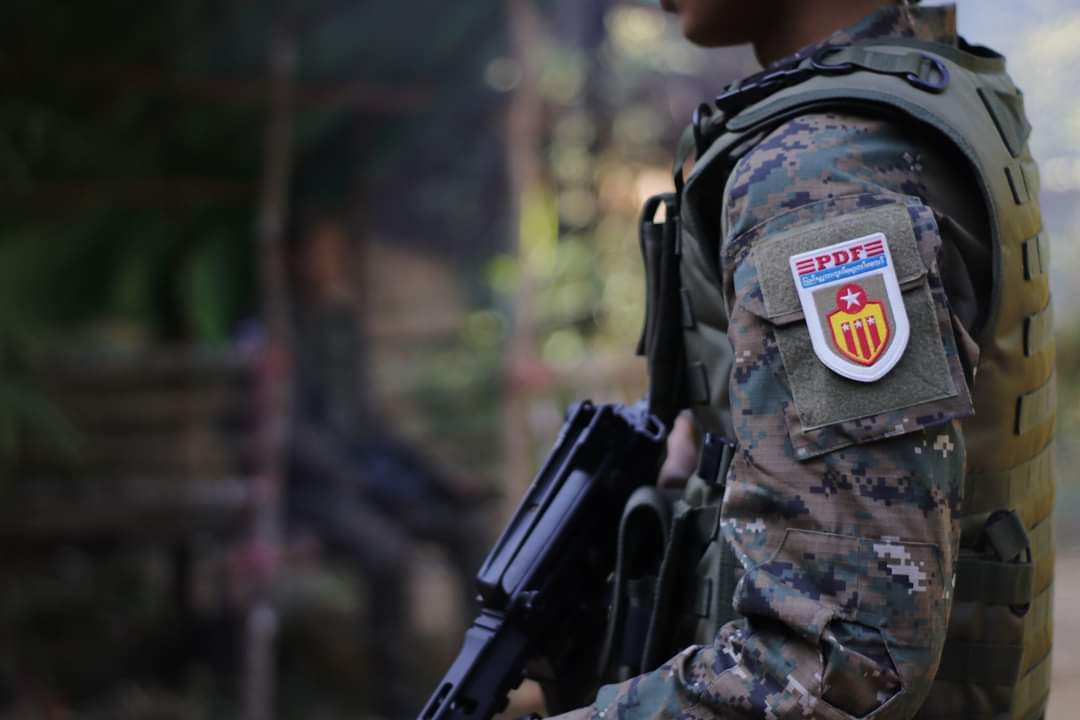
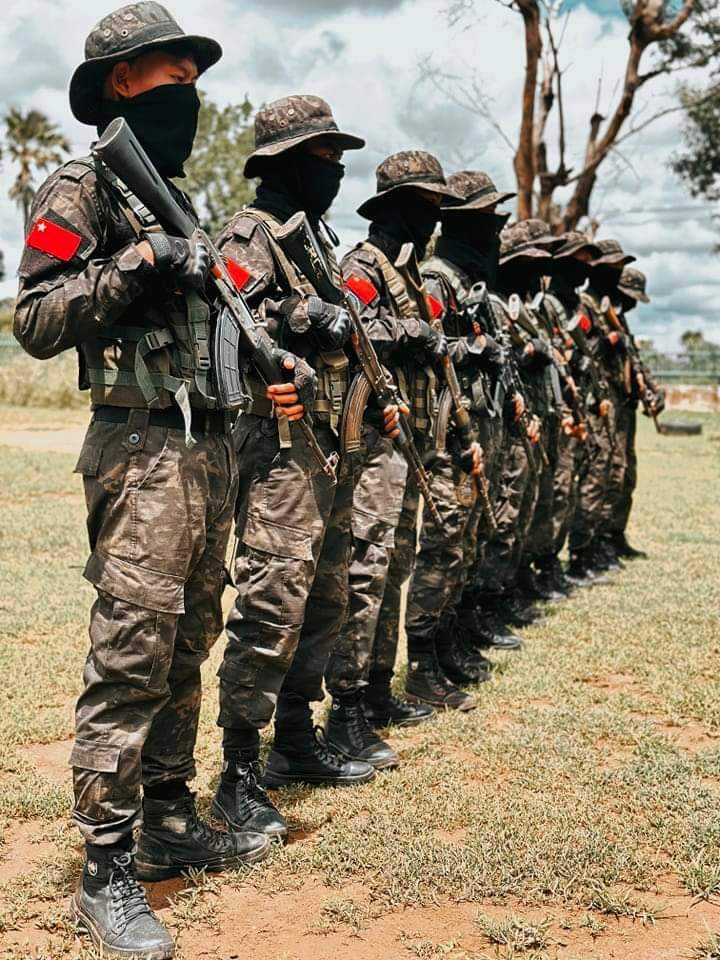
– **Impact:** High-quality data leads to more accurate predictions. Insufficient or biased data can result in skewed models, reinforcing existing biases.
### 2. **Clear Objectives and Use Cases**
– **Role:** Defining clear objectives and specific use cases for the AI project is essential to guide development.
– **Impact:** Clear objectives help in aligning the project with business goals, ensuring that the efforts lead to tangible benefits and valuable outcomes.
### 3. **Choosing the Right Algorithms and Techniques**
– **Role:** Selecting appropriate algorithms based on the nature of the data and the objectives is crucial.
– **Impact:** The choice of algorithms can greatly influence the model’s performance. Understanding the strengths and weaknesses of different approaches is necessary for optimal outcomes.
### 4. **Expertise and Skill Set of the Team**
– **Role:** The knowledge and experience of the data scientists, engineers, and domain experts involved are pivotal in driving the project forward.
– **Impact:** A well-equipped team can make informed decisions regarding modeling, feature engineering, data collection, and interpretation of results, ultimately resulting in a robust AI solution.
### 5. **Iterative Development and Prototyping**
– **Role:** An iterative approach allows for continuous improvement and refinement of models based on feedback and performance metrics.
– **Impact:** This helps in rapidly identifying issues and adapting the model or strategy, leading to a more effective and adaptable solution.
### 6. **Evaluation Metrics and Performance Monitoring**
– **Role:** Establishing proper metrics for model evaluation is essential to measure success against project objectives.
– **Impact:** Metrics guide the optimization process and help in assessing the model’s performance, leading to informed decisions about deployment or further development.
### 7. **Interdisciplinary Collaboration**
– **Role:** AI projects often require collaboration between data scientists, domain experts, software developers, and business stakeholders.
– **Impact:** Such collaboration ensures that all perspectives are considered, leading to a more comprehensive understanding of the challenges and better integration of AI solutions in business processes.
### 8. **Deployment Strategy**
– **Role:** The method of deploying the AI model into a production environment is critical.
– **Impact:** A well-planned deployment ensures that the model integrates seamlessly with existing systems and can be scaled effectively, leading to better user experiences and business impact.
### 9. **Ethical Considerations and Governance**
– **Role:** Addressing ethical concerns, such as bias, transparency, and accountability, is increasingly important in AI development.
– **Impact:** Ethical AI practices help build trust with stakeholders and users, ensuring that the AI systems are fair and responsible, minimizing backlash or resistance.
### 10. **Documentation and Knowledge Sharing**
– **Role:** Maintaining comprehensive documentation is essential for knowledge transfer and ensuring reproducibility.
– **Impact:** Good documentation facilitates onboarding new team members, supports collaboration, and provides valuable references for future projects.
### 11. **Feedback Mechanisms**
– **Role:** Establishing mechanisms for receiving feedback from users and stakeholders helps refine models and ensure they meet real-world needs.
– **Impact:** Feedback informs future iterations and improvements, aligning the AI system with user expectations and requirements.
### Conclusion
Each of these elements plays a pivotal role in determining the success of AI projects. By addressing these factors, organizations can significantly enhance their chances of developing effective and impactful AI solutions, ultimately leading to better business outcomes and advancements in technology.
Leave a Reply