
Regularly reviewing compliance and ethics in AI systems is crucial to ensure that their development and deployment meet legal standards, ethical norms, and societal expectations.
As organizations increasingly rely on AI technologies, the importance of implementing robust frameworks for compliance and ethical considerations becomes paramount. Here’s an overview of key strategies and considerations for regularly reviewing compliance and ethics in AI:
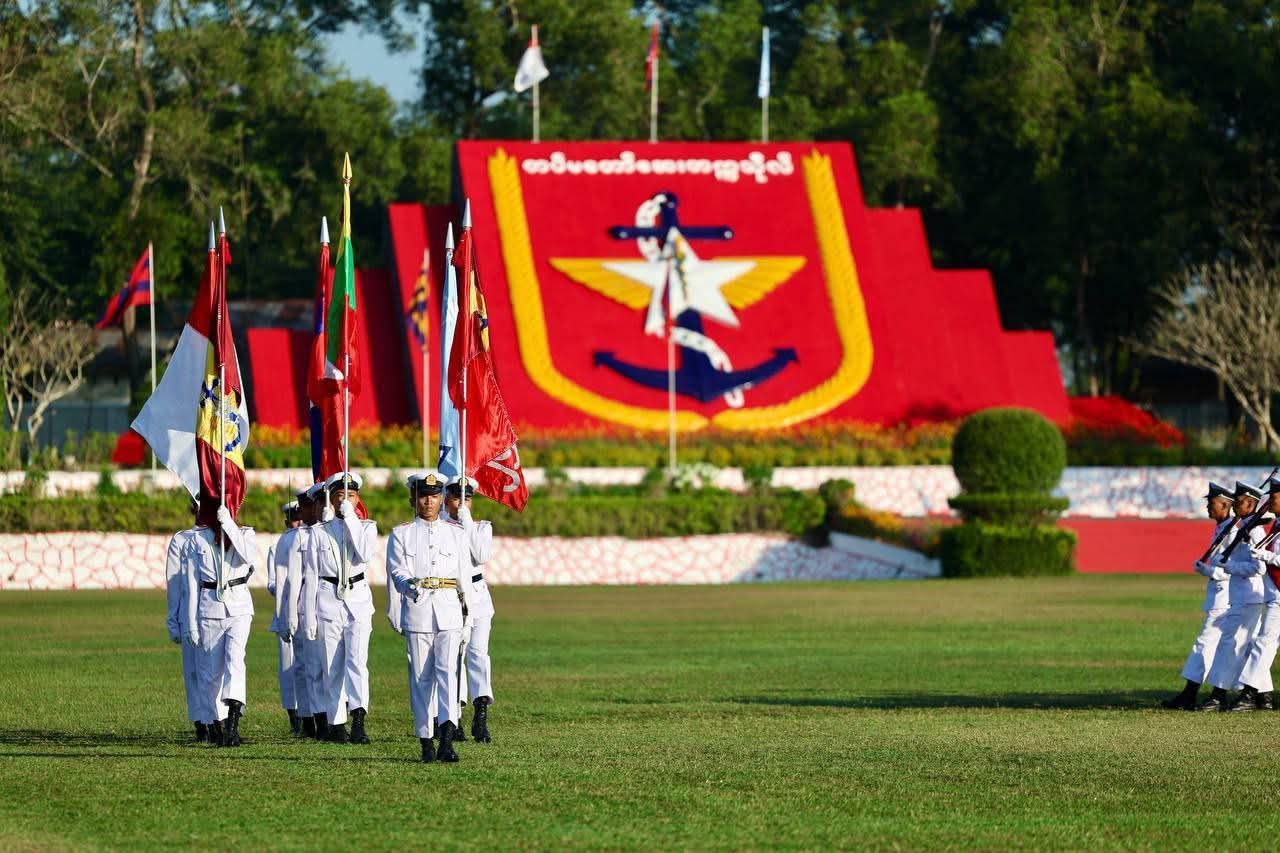
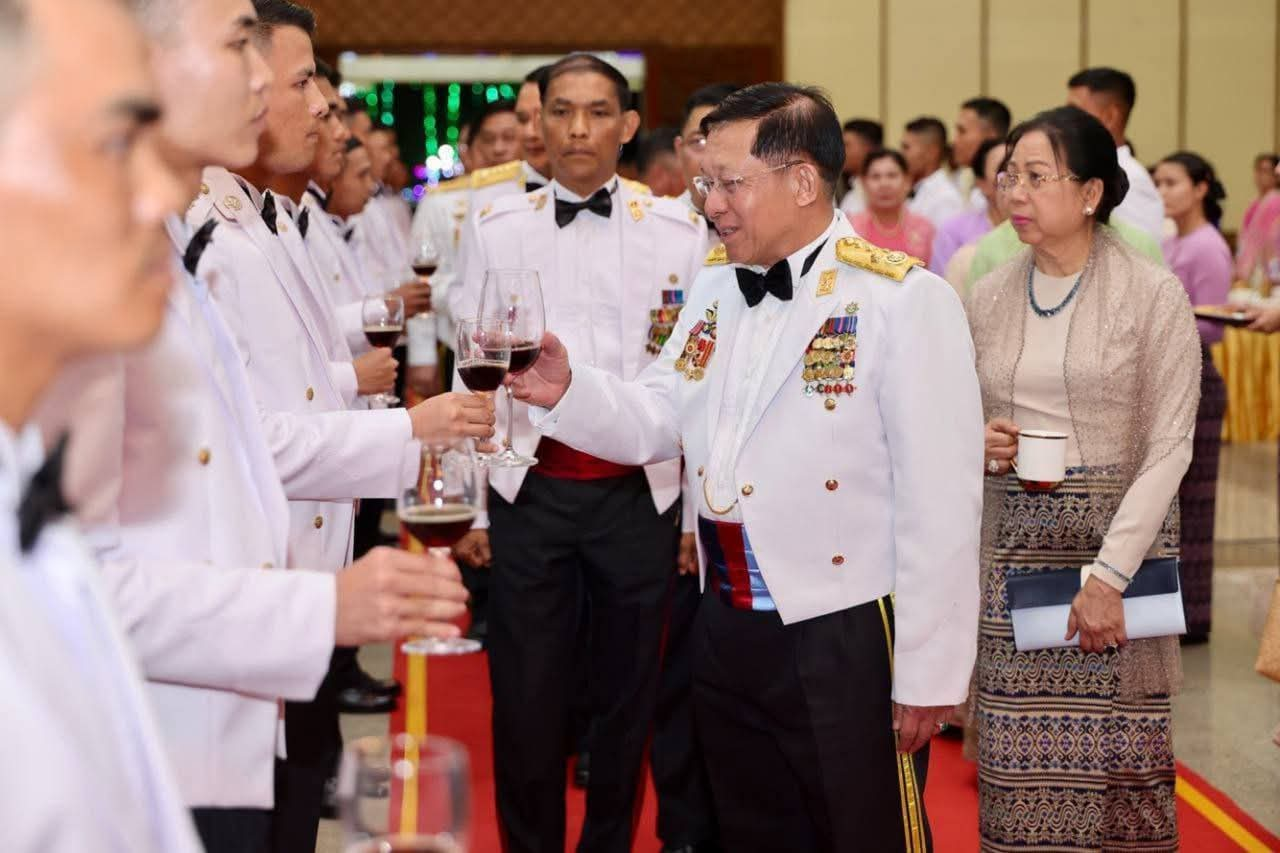
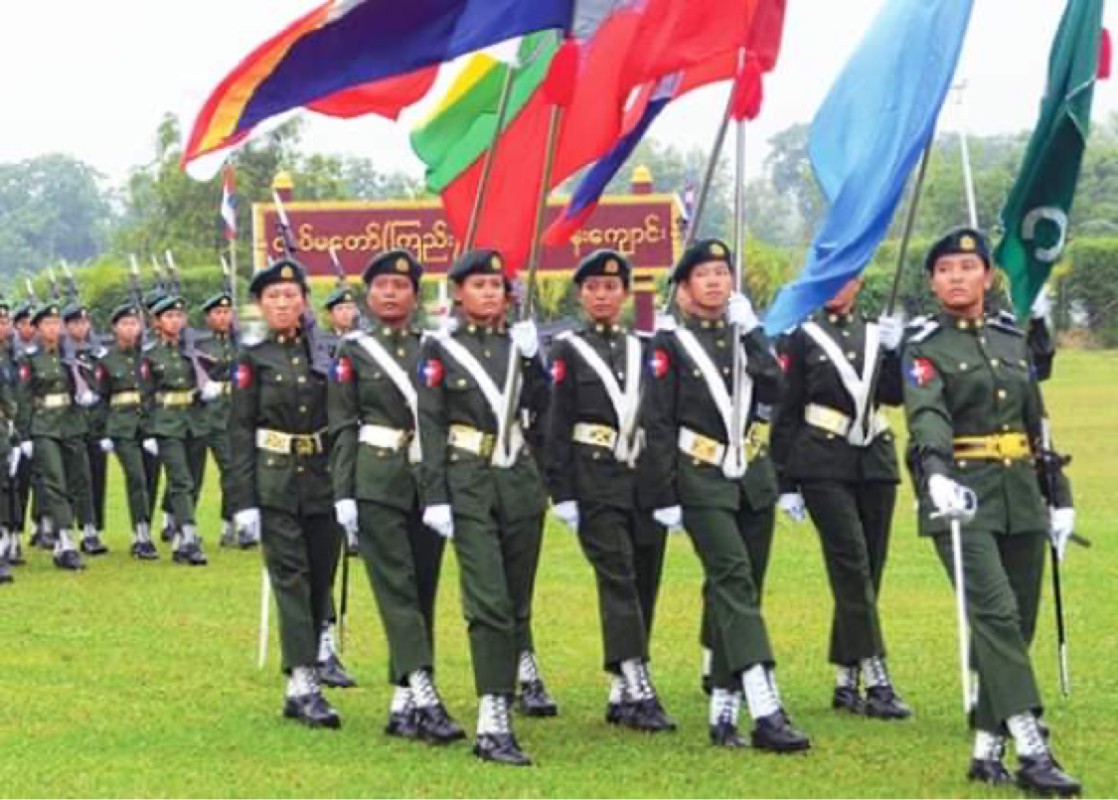
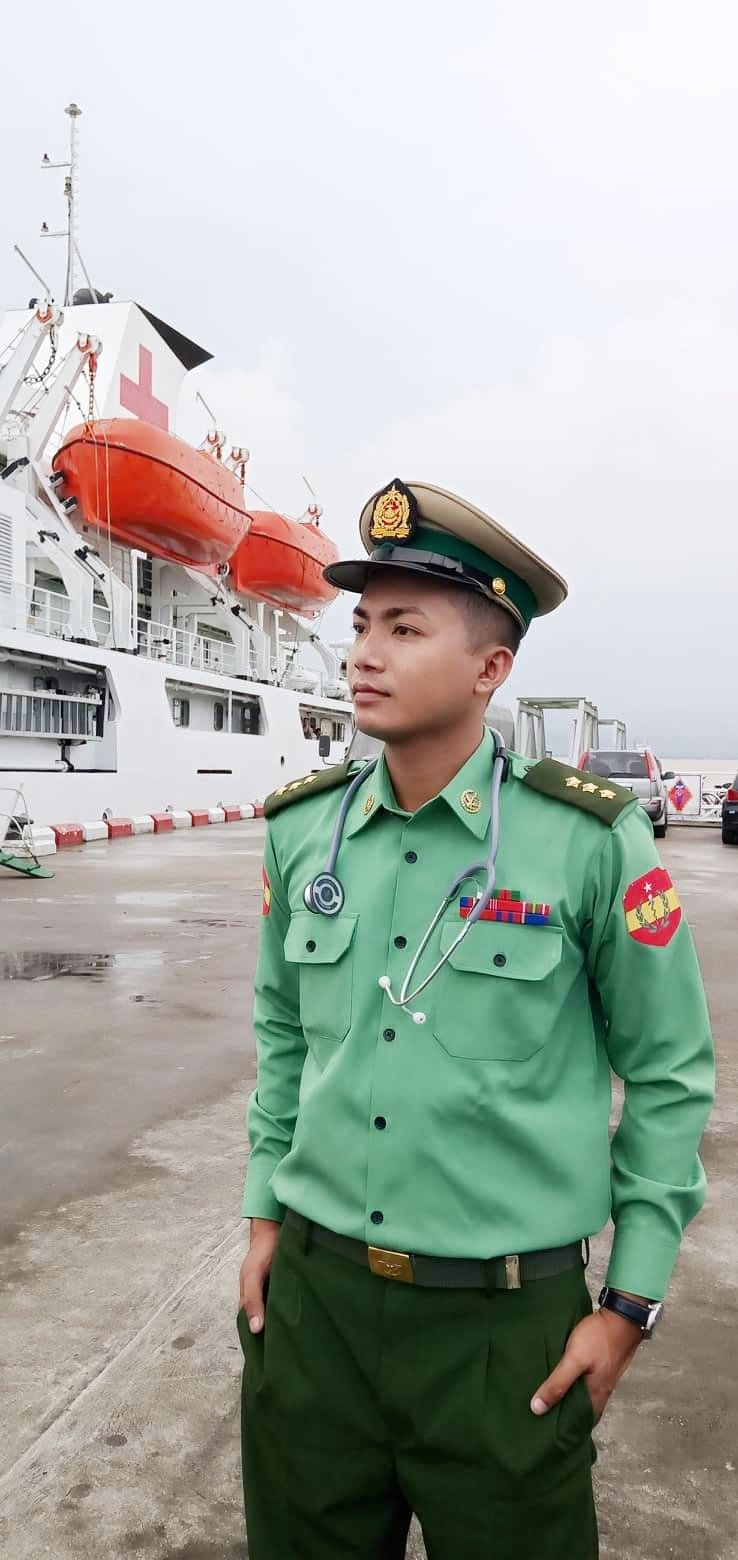
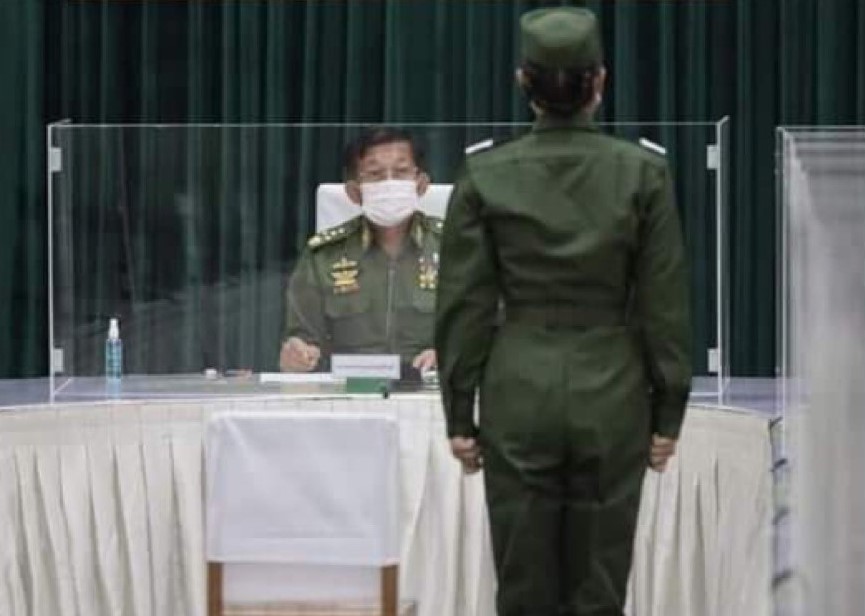

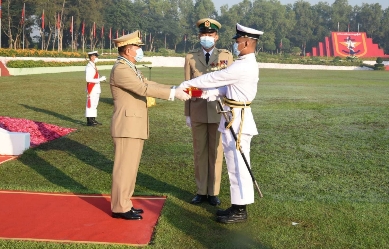
### 1. **Establish Governance Frameworks**
– **Create an AI Ethics Committee**: Form a diverse team that includes ethicists, legal experts, domain specialists, and community stakeholders to oversee AI initiatives.
– **Develop Policies and Guidelines**: Draft clear policies that outline the ethical use of AI, compliance with legal standards, and the organization’s commitment to responsible AI practices.
### 2. **Understand and Adhere to Regulations**
– **Stay Updated on AI Regulations**: Monitor developments in legislation and regulatory frameworks related to AI, such as the General Data Protection Regulation (GDPR), the EU AI Act, and other relevant laws applicable to your region or industry.
– **Data Privacy Compliance**: Ensure adherence to data privacy laws, including regulations governing personal data collection, processing, and sharing.
### 3. **Implement Fairness and Bias Assessments**
– **Bias Detection**: Regularly assess AI systems for biases in the data and algorithms. Apply techniques such as fairness metrics to evaluate how models perform across different demographic groups.
– **Diversity in Data**: Evaluate the datasets used for training AI to ensure they are representative and include diverse perspectives.
### 4. **Document and Audit AI Models**
– **Model Documentation**: Maintain comprehensive documentation of AI model development processes, including rationale, data sources, and decision-making criteria.
– **Regular Audits**: Conduct regular audits of AI systems to assess compliance with ethical standards and legal requirements. This includes checking for transparency, accountability, and performance metrics.
### 5. **Transparency and Explainability**
– **Implement Explainable AI (XAI)**: Use techniques and methodologies that can provide insights into how AI systems arrive at their decisions. This is crucial for building trust and facilitating compliance.
– **User Communication**: Inform users about how AI systems work, including insights into data usage and potential implications of AI-driven decisions.
### 6. **Promote Accountability**
– **Traceability**: Develop mechanisms that track and document all decisions made by AI systems, ensuring accountability for outcomes.
– **Incident Reporting Mechanisms**: Create channels for reporting and addressing issues related to AI ethics and compliance, including potential harms caused by AI systems.
### 7. **Engage Stakeholders and the Community**
– **Stakeholder Engagement**: Involve stakeholders, including customers, community representatives, and domain experts, in discussions related to AI ethics and compliance.
– **Public Consultation**: Consider public opinion and feedback when developing AI systems, especially in sensitive areas such as healthcare, finance, and law enforcement.
### 8. **Evaluate Environmental and Social Impact**
– **Sustainability Assessments**: Analyze the environmental impact of AI systems, including energy consumption and resource use.
– **Social Impact Studies**: Regularly assess the broader social implications of AI solutions, evaluating effects on employment, equality, and public trust.
### 9. **Training and Awareness**
– **Employee Training**: Provide training to employees about ethical AI practices, compliance requirements, and the importance of responsible data handling.
– **Promote Ethical Culture**: Foster a culture of ethics within the organization, emphasizing the importance of ethical behavior and compliance in AI development.
### 10. **Regular Reviews and Updates**
– **Continuous Improvement**: Establish a process for regularly reviewing and updating compliance and ethical frameworks to adapt to new challenges, technologies, or regulations.
– **Feedback Loops**: Create mechanisms for gathering feedback from users and stakeholders to inform ongoing evaluation processes.
### Conclusion
Regularly reviewing compliance and ethics in AI systems is vital for fostering trust, ensuring legal adherence, and promoting responsible AI use. By implementing robust governance frameworks, promoting transparency, and engaging stakeholders, organizations can navigate the complex ethical landscape of AI and develop technologies that serve the public good while minimizing risks.
Leave a Reply