
Prioritizing accessibility and inclusivity in AI is essential for creating technologies that serve all users, regardless of their abilities, background, or environment.
This approach not only aligns with ethical standards and legal requirements but also enhances user satisfaction, expands market reach, and fosters innovation. Here’s a detailed exploration of strategies and best practices for integrating accessibility and inclusivity into AI systems:
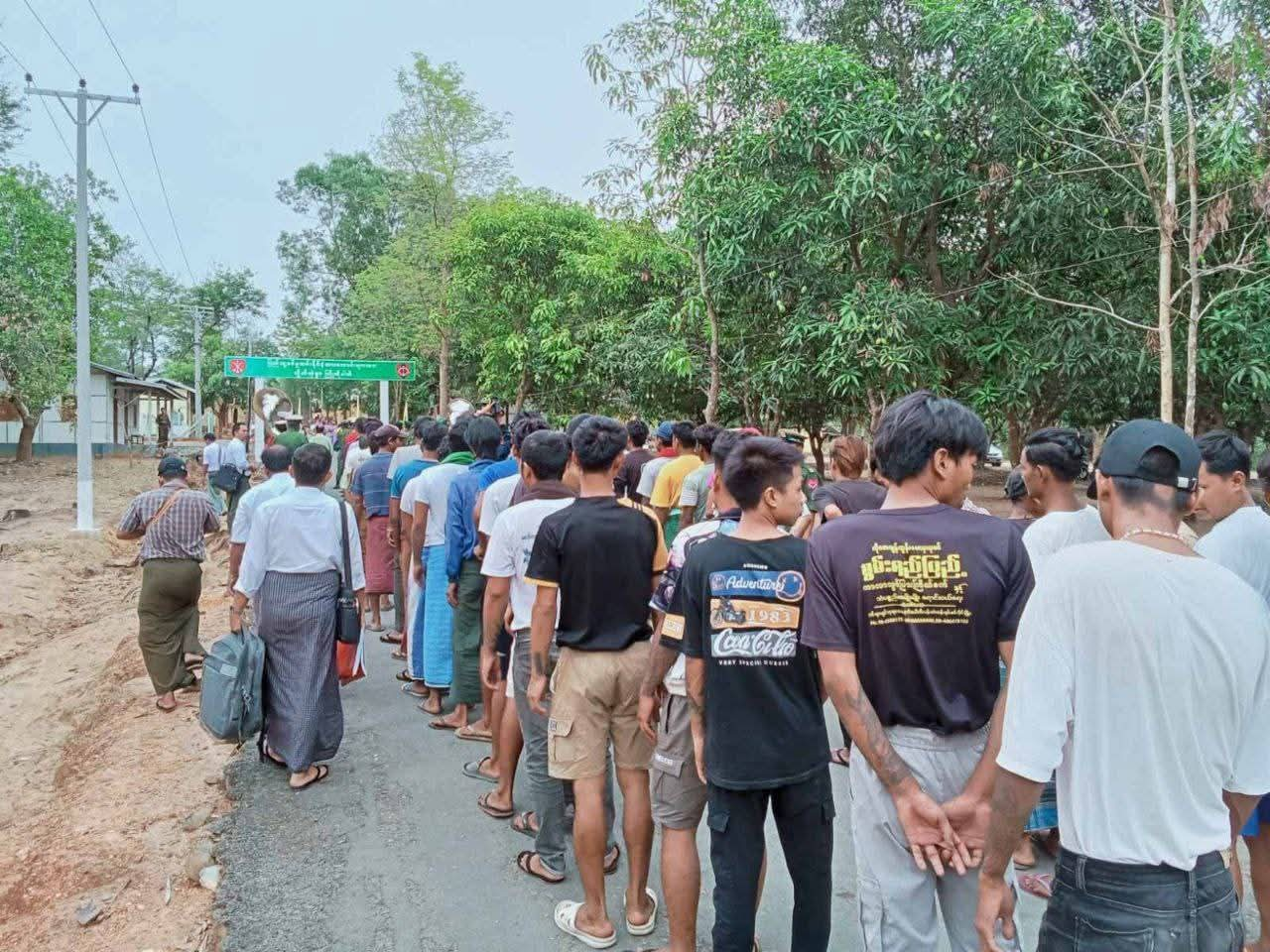




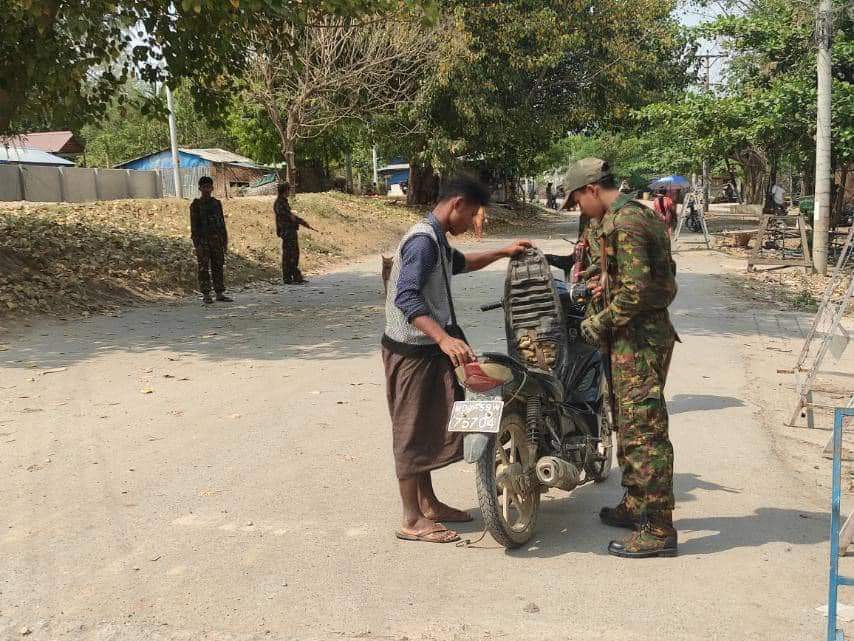
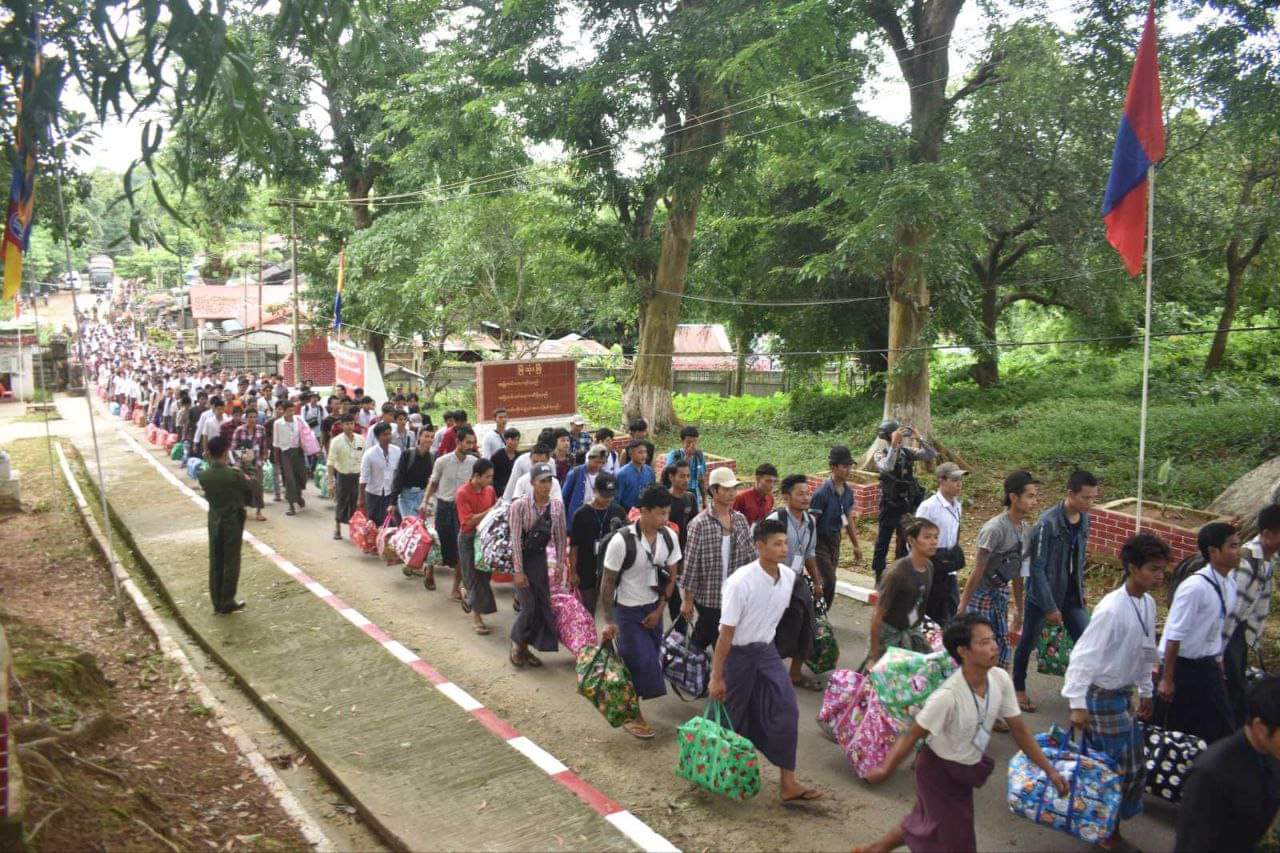
### Why Accessibility and Inclusivity Matter in AI
1. **Diverse User Needs**: Users come from different backgrounds, with various abilities and preferences. Accessibility ensures that AI products can be used by individuals with disabilities, while inclusivity caters to diverse cultural and socio-economic backgrounds.
2. **Legal Compliance**: Many countries have laws and regulations (like the Americans with Disabilities Act in the U.S. and the European Accessibility Act) that require digital products to be accessible.
3. **Enhanced User Experience**: Making AI systems accessible and inclusive often leads to a better overall user experience for all users, not just those with disabilities.
4. **Market Expansion**: Creating inclusive products allows businesses to reach a larger audience and enter new markets by catering to underserved populations.
### Key Principles of Accessibility and Inclusivity
1. **Universal Design**: Aim for an approach that meets the needs of all users without the need for adaptation or specialized design, enhancing usability for everyone.
2. **User-Centered Design**: Place users at the heart of the design process by incorporating their feedback and involving them throughout development and testing.
3. **Continuous Improvement**: Recognize that accessibility and inclusivity are ongoing goals that require regular feedback, updates, and iterations based on user experiences.
### Strategies for Prioritizing Accessibility and Inclusivity in AI
#### 1. **Inclusive User Research**
– **Diverse Focus Groups**: Organize focus groups that include users with disabilities and people from various cultural backgrounds to gather insights into their needs and preferences.
– **Field Studies**: Conduct ethnographic studies to understand how people from different demographics interact with technology in real-world settings.
#### 2. **Design Considerations**
– **Text and Language**: Use clear, simple language. Avoid jargon and idioms that may confuse users from different linguistic backgrounds. Consider language preferences and local dialects.
– **Visual Design**: Ensure high color contrast, provide alternative text for images, and allow users to customize text sizes and colors. Test color palettes for accessibility for colorblind users.
– **Interaction Design**: Design intuitive, easy-to-navigate interfaces with predictable layouts. Ensure that all interactive elements are clearly labeled.
#### 3. **Integration with Assistive Technologies**
– **Compatibility**: Ensure your AI application is compatible with screen readers, voice recognition software, and other assistive technologies.
– **Voice Activation**: Implement voice recognition capabilities that accommodate various accents, dialects, and speech patterns to cater to users with different linguistic backgrounds and those who may have difficulty using traditional input devices.
#### 4. **AI Model Training and Data Diversity**
– **Diverse Data Sets**: Train AI models on diverse datasets that represent various demographics and experiences to ensure that the AI can recognize and serve a broad array of users.
– **Bias Identification**: Regularly audit and test AI models for biases, ensuring that they do not discriminate against certain groups based on race, gender, disability, or other attributes.
#### 5. **User Feedback Loop**
– **Feedback Mechanisms**: Implement straightforward channels for users to provide feedback on accessibility issues and suggestions for improvements.
– **Beta Testing**: Engage users from diverse backgrounds in beta testing programs to gain insights that can lead to early identification of accessibility issues.
#### 6. **Education and Awareness**
– **Team Training**: Educate development teams about accessibility standards and the importance of inclusivity in AI design. Provide resources and training on tools and techniques for creating accessible products.
– **Advocate for Accessibility**: Foster a culture within the organization that values and prioritizes accessibility and inclusivity in every project stage.
#### 7. **Adherence to Guidelines and Standards**
– **Compliance with WCAG**: Follow the Web Content Accessibility Guidelines (WCAG) to meet industry standards for web accessibility.
– **Relevant Regulations**: Stay informed about laws and regulations surrounding digital accessibility and ensure the product complies with legal standards.
### Examples of Inclusive and Accessible AI Applications
– **Screen Readers**: Implementing features that allow text-to-speech and voice commands for visually impaired users.
– **Smart Assistants**: Voice-activated smart assistants can be designed to understand a broad range of accents and speech patterns to cater to diverse user groups.
– **AI Chatbots**: Chatbots that employ natural language processing should be trained on diverse conversations and contexts to understand and respect cultural differences in communication.
### Conclusion
Prioritizing accessibility and inclusivity in AI is not just a regulatory obligation; it is a commitment to serving all users and leveraging the full potential of technology. By integrating inclusive design principles, engaging diverse user groups, and continuously iterating based on feedback, organizations can create AI systems that are not only functional but also empowering for all users. This approach leads to innovation, enhanced user loyalty, and a positive social impact, ultimately contributing to a more equitable digital landscape.
Leave a Reply