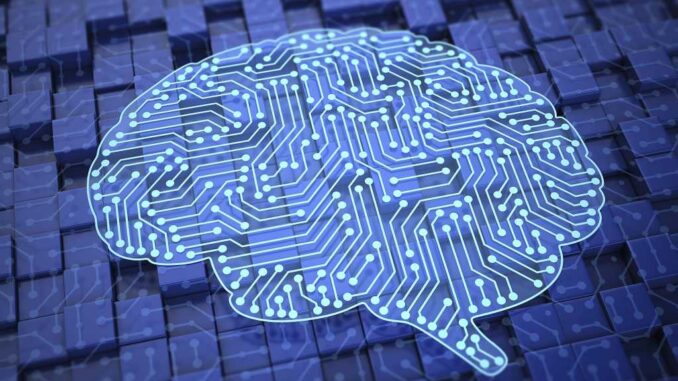
AI democratization refers to the process of making artificial intelligence (AI) technologies and tools accessible to a broader range of individuals and organizations, regardless of their technical expertise or resources.
The goal is to empower more people to leverage AI for various applications, thereby fostering innovation, creativity, and problem-solving capabilities across diverse sectors. Here are some key aspects and implications of AI democratization:



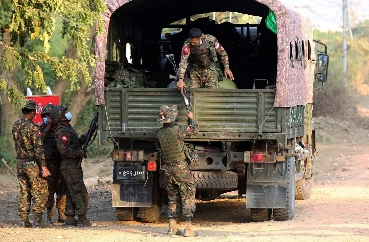
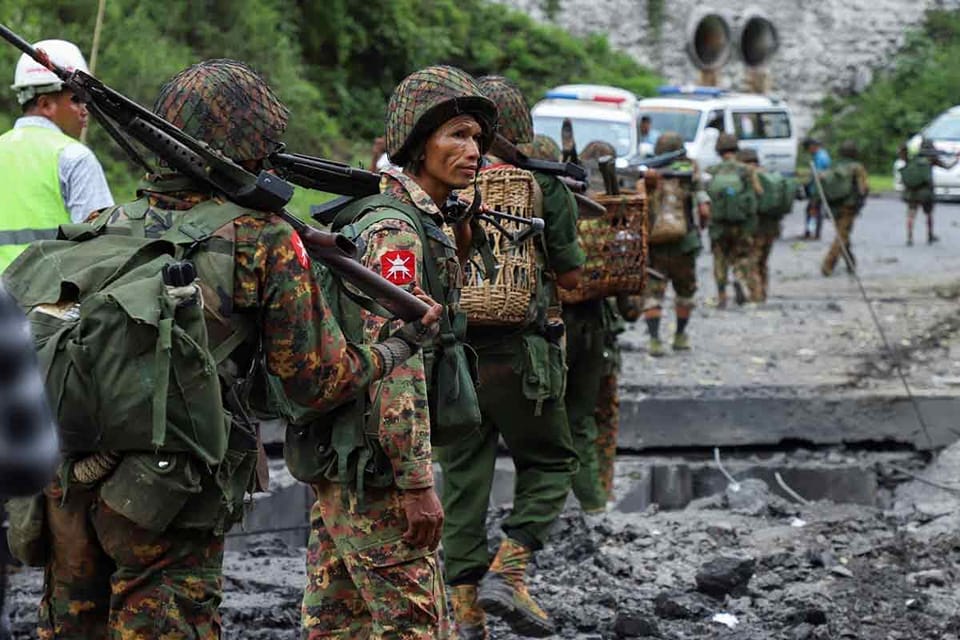
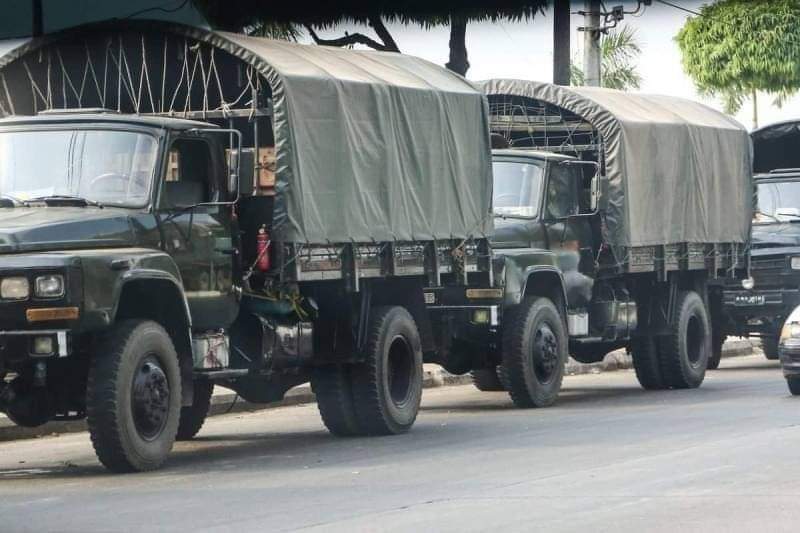
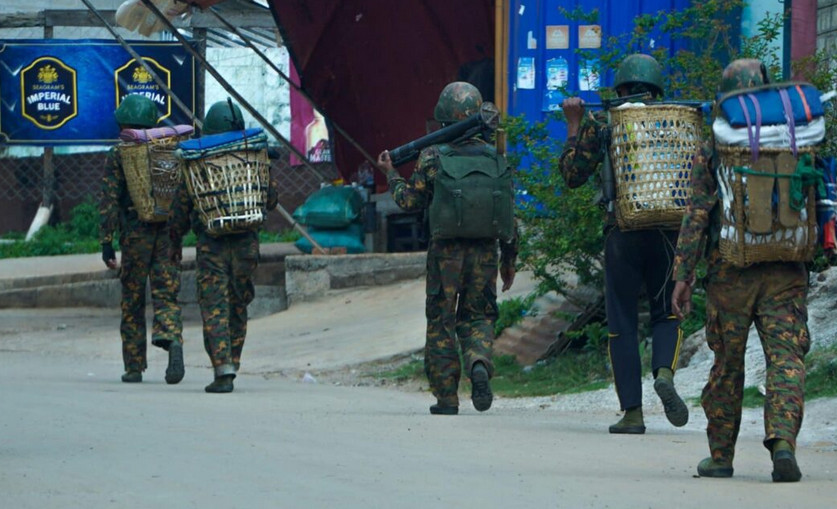
### 1. **Accessibility of Tools and Resources**
– **User-Friendly Interfaces**: Development of low-code or no-code platforms that allow users with limited technical skills to create and deploy AI models. Examples include platforms like Google AutoML and Microsoft Power Automate.
– **Open-Source Frameworks**: Availability of open-source libraries and frameworks such as TensorFlow, PyTorch, and Scikit-learn, which provide tools for developing AI applications without the need for extensive financial investment.
### 2. **Education and Training**
– **Online Courses and Resources**: Growth of online education platforms (like Coursera, edX, and Udacity) offering courses in AI and machine learning, making quality education accessible around the world.
– **Community-Driven Learning**: Initiatives such as meetups, hackathons, and forums that foster community engagement and knowledge sharing among aspiring AI practitioners.
### 3. **Data Accessibility**
– **Public Datasets**: Availability of open datasets (such as those on Kaggle, UCI Machine Learning Repository, and government portals) that allow individuals to train and experiment with AI models effectively.
– **Synthetic Data Generation**: Techniques that generate synthetic data for training, further reducing reliance on large, real-world datasets that might not be easily available.
### 4. **Affordability**
– **Cloud Computing**: The rise of cloud platforms (e.g., AWS, Google Cloud, Azure) that provide scalable and cost-effective resources for running AI workloads, enabling small businesses and startups to utilize advanced AI technologies without significant upfront investment.
### 5. **Collaboration and Innovation**
– **Cross-Disciplinary Applications**: Democratization enables professionals from various fields (healthcare, education, agriculture) to apply AI to their specific domains, leading to innovative solutions that address niche problems.
– **Open Innovation Platforms**: Crowdsourcing solutions through platforms that invite external contributors to develop or improve AI models, leading to collaborative innovation.
### 6. **Ethical Considerations**
– **Bias and Fairness**: As more people engage with AI, the risk of perpetuating biases increases. Democratization must involve efforts to educate users about ethical AI practices and the importance of diverse and representative training data.
– **Transparency**: Encouraging transparency in AI development to ensure that the models created by non-experts are understandable and interpretable, reducing the “black box” problem.
### 7. **Emerging Trends**
– **AI for Good Initiatives**: Initiatives aimed at leveraging AI to tackle global challenges (like climate change, healthcare, and education) by enabling a diverse set of participants to contribute solutions.
– **Citizen Data Scientists**: The rise of individuals who utilize AI tools to analyze data and derive insights without formal data science training.
### Conclusion
AI democratization holds the promise of unleashing creativity and innovation by enabling wider access to AI technologies. However, it also necessitates a balanced approach that emphasizes ethical practices, education, and inclusivity. By empowering individuals and organizations with the tools and knowledge to use AI responsibly, society can harness the transformative potential of this technology for the benefit of all.
Leave a Reply