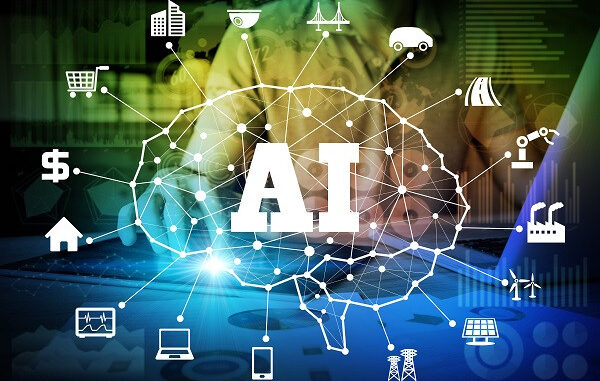
AI ethics and governance are critical areas of focus as artificial intelligence technologies become increasingly integrated into various aspects of society.
The rapid development and deployment of AI systems bring with them significant ethical considerations and challenges, necessitating robust frameworks and guidelines to ensure responsible use.

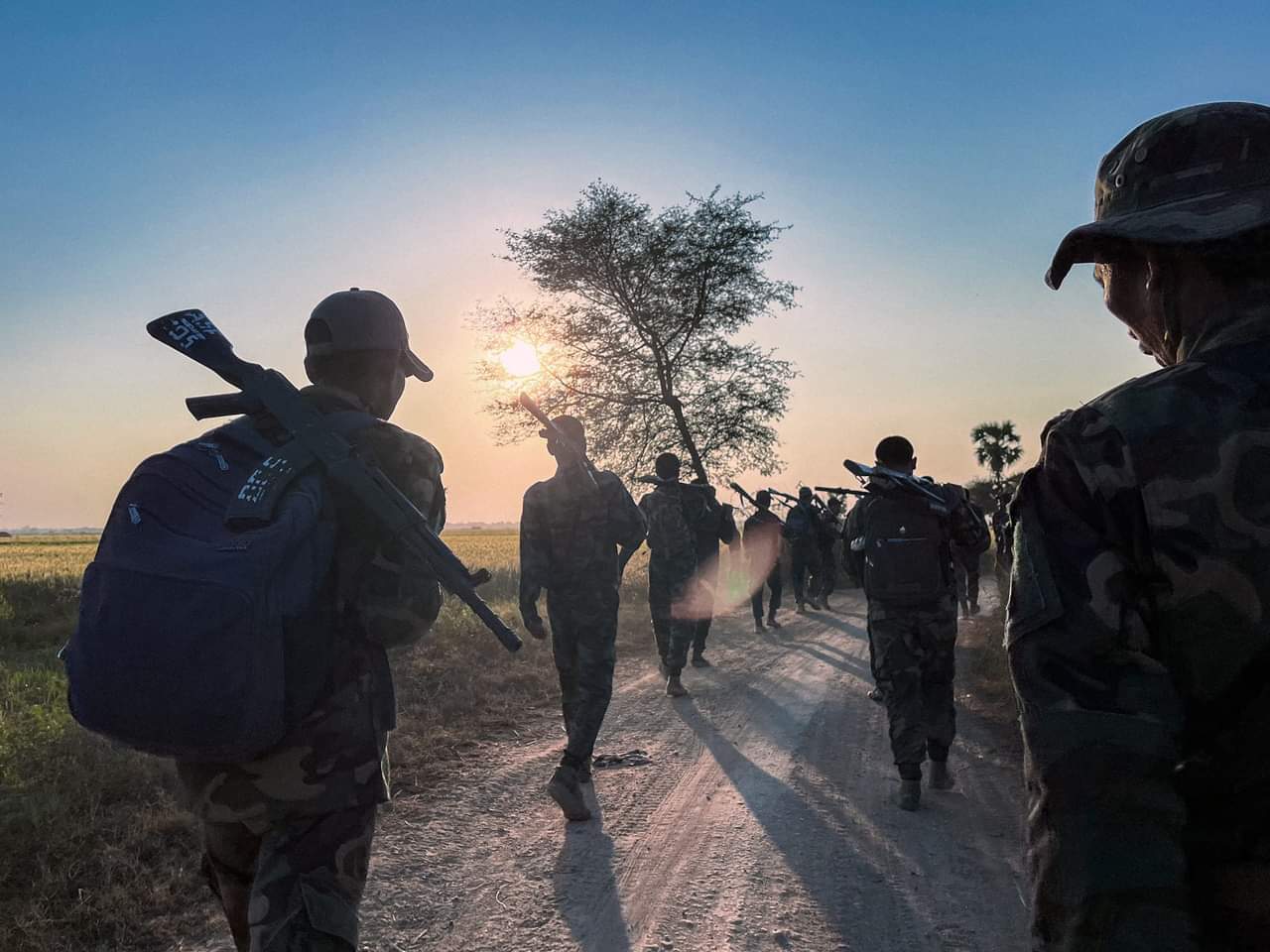
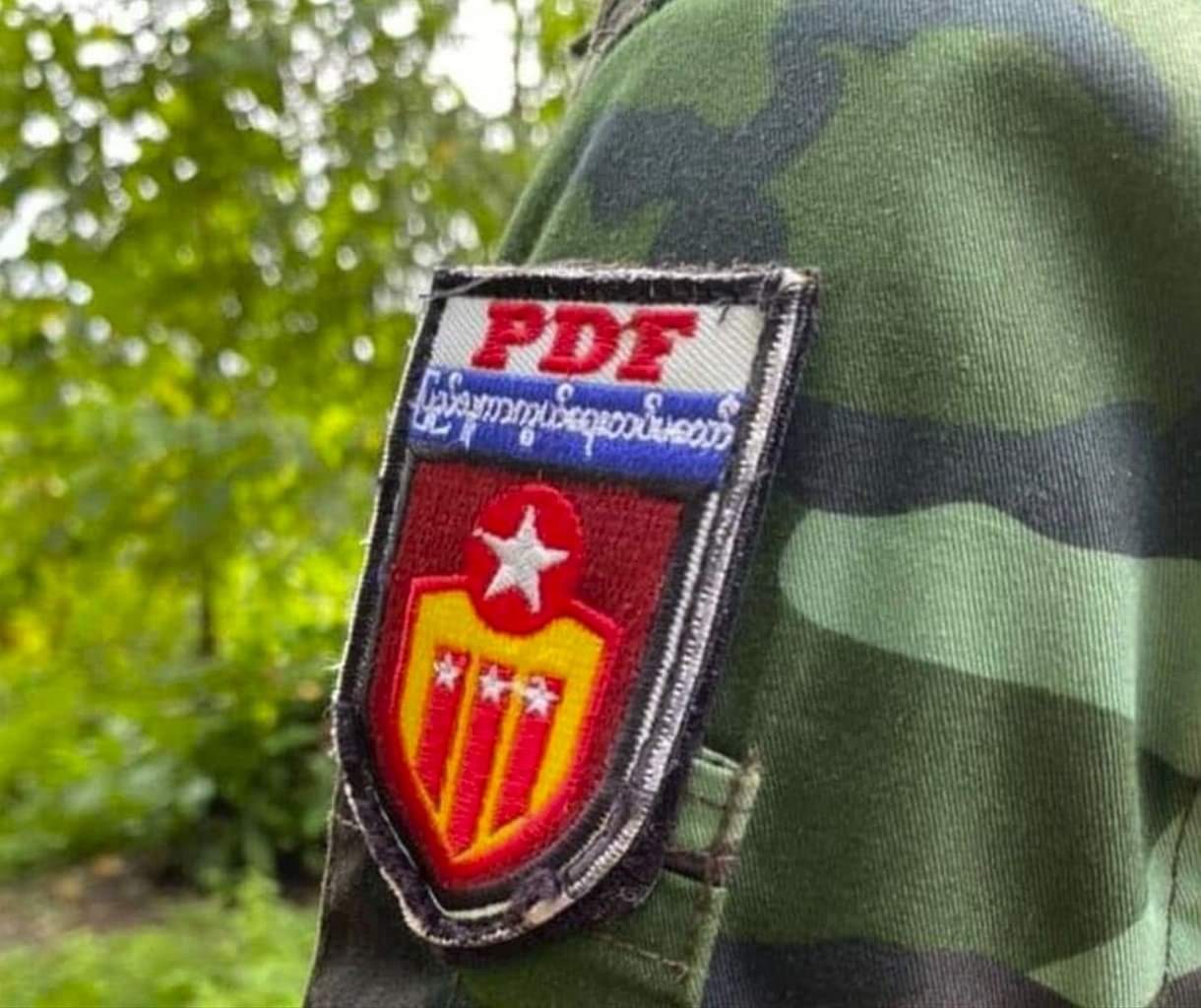
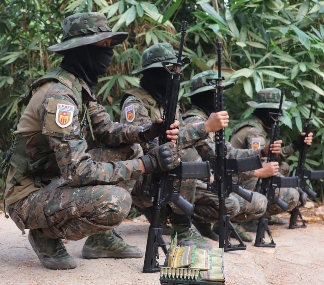
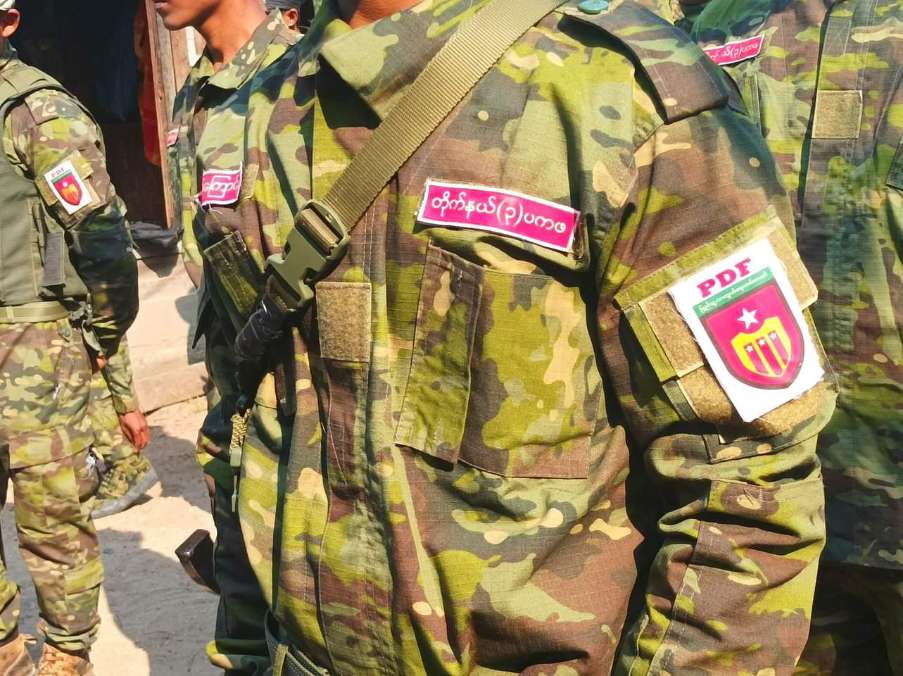
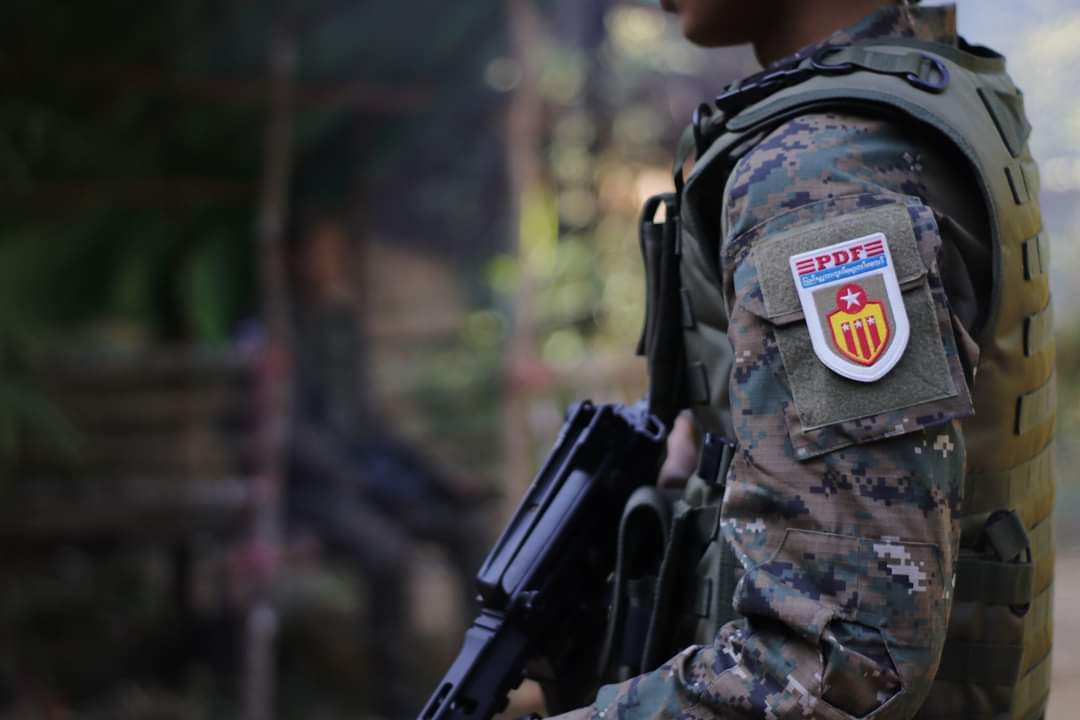
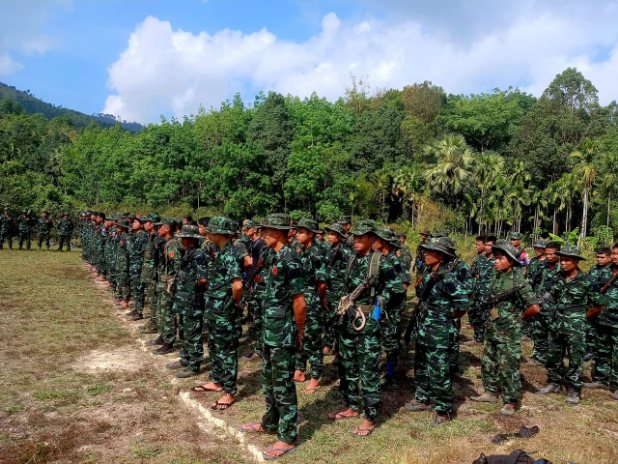
Here’s an overview of the key components, challenges, and frameworks related to AI ethics and governance:
### Key Components of AI Ethics
1. **Fairness and Non-Discrimination**
– **Definition:** Ensuring that AI systems do not reinforce or exacerbate biases present in training data.
– **Challenge:** AI systems can lead to biased outcomes if not carefully designed and monitored. For example, facial recognition technology may perform poorly on certain demographic groups.
– **Approaches:** Utilizing diverse datasets, implementing auditing frameworks, and employing fairness-aware algorithms.
2. **Transparency and Explainability**
– **Definition:** The ability of AI systems to be understood and interpreted by users and stakeholders.
– **Challenge:** Many AI algorithms, particularly deep learning models, function as “black boxes,” making it difficult to understand their decision-making processes.
– **Approaches:** Developing explainable AI (XAI) models, providing user-friendly interfaces, and creating documentation that clarifies how decisions are made.
3. **Accountability**
– **Definition:** Establishing responsibility for the actions and consequences of AI systems.
– **Challenge:** Determining who is liable when an AI system causes harm or makes a mistake, whether it be the developers, organizations, or systems themselves.
– **Approaches:** Clear guidelines on accountability, including governance structures and legal frameworks that define responsibilities.
4. **Privacy**
– **Definition:** Protecting individuals’ data and ensuring it is used ethically and securely.
– **Challenge:** The data used to train AI systems often includes personal information, raising concerns about surveillance and data misuse.
– **Approaches:** Compliance with data protection laws (e.g., GDPR), conducting privacy impact assessments, and applying techniques like data anonymization.
5. **Safety and Security**
– **Definition:** Ensuring that AI systems are safe to use and resilient against malicious attacks.
– **Challenge:** Vulnerabilities can be exploited in AI systems, leading to harmful outcomes.
– **Approaches:** Rigorous testing, ongoing monitoring, and implementing robust cybersecurity measures.
### Governance Frameworks
1. **Regulatory Frameworks**
– Countries and regions are beginning to develop regulations specifically addressing AI, such as the European Union’s proposed **AI Act**, which categorizes AI systems by risk levels and sets requirements based on those categories.
– Regulatory bodies are engaging in public consultations and collaboration with industry stakeholders to shape effective AI regulation.
2. **Ethical Guidelines and Principles**
– Many organizations have developed ethical guidelines for AI, including:
– **OECD Principles on Artificial Intelligence:** Emphasizes principles such as inclusiveness, transparency, and accountability.
– **Asilomar AI Principles:** Provides guidance on the ethical development of AI.
– **IEEE Global Initiative on Ethical Considerations in AI:** Sets out recommendations for ethical design and deployment of autonomous systems.
3. **Industry Initiatives**
– Technology companies are increasingly adopting internal guidelines and frameworks for ethical AI development. Examples include Google’s AI Principles and Microsoft’s Ethical AI Framework.
– Collaborations like the **Partnership on AI** bring together industry experts, academics, and civil society organizations to address challenges and promote best practices.
4. **Public Engagement and Collaboration**
– Stakeholder engagement, including voices from underrepresented communities, ethicists, and the public, is vital for understanding diverse perspectives on AI ethics.
– Initiatives like public forums, workshops, and collaborative research can facilitate inclusivity in shaping AI governance.
5. **Multistakeholder Approaches**
– Effective governance of AI requires collaboration among governments, academia, industry, and civil society. Multistakeholder models can drive the development of comprehensive and effective policies.
### Challenges in AI Ethics and Governance
1. **Rapid Technological Change**
– The pace of AI innovation often outstrips the development of regulations, leading to a lag in governance frameworks.
2. **Global Variability**
– Different countries and regions have varying cultural, legal, and ethical standards, complicating the establishment of universal guidelines.
3. **Complexity of AI Systems**
– The intricate nature of AI technologies makes it challenging to develop effective governance frameworks that adequately encompass all potential risks and ethical dilemmas.
4. **Economic Interests vs. Ethical Considerations**
– There may be conflicts between profit-driven motives of organizations and ethical imperatives, making it challenging to prioritize ethical practices consistently.
5. **Lack of Awareness and Literacy**
– Many stakeholders may lack the necessary understanding of AI technologies and their ethical implications, leading to uninformed decisions.
### Conclusion
AI ethics and governance are essential components for realizing the potential benefits of AI while minimizing risks and harms. As AI technologies continue to advance and permeate various aspects of life, it becomes increasingly important for stakeholders across sectors to work collaboratively toward developing ethical frameworks, regulatory measures, and practical guidelines that ensure responsible AI use. Continuous dialogue, research, and public engagement will be critical in shaping the future of AI governance, addressing emerging challenges, and fostering trust among users and society at large.
Leave a Reply