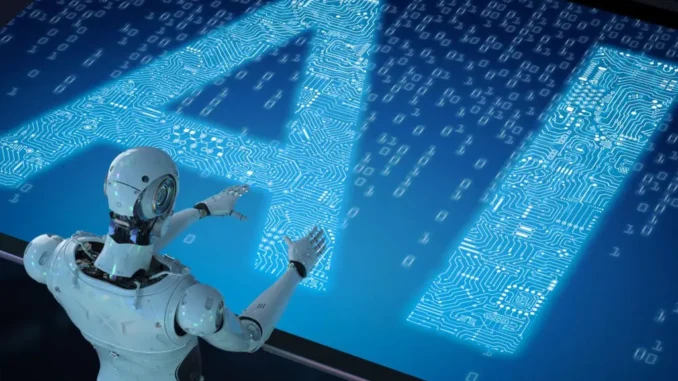
Post-disaster analysis using artificial intelligence (AI) involves applying data analytics, machine learning, and computer vision techniques to assess
the impact of natural disasters, enhance recovery efforts, and inform future preparedness strategies. Here are some ways AI can contribute to post-disaster analysis:
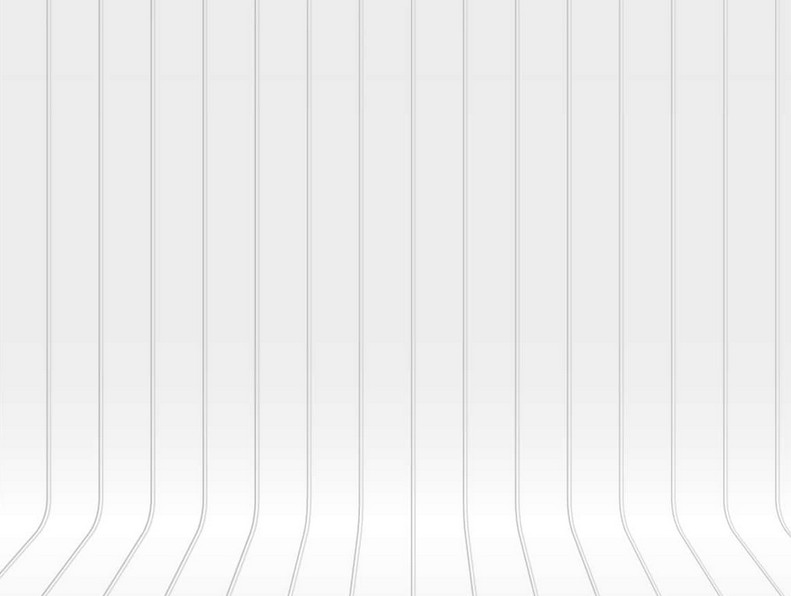
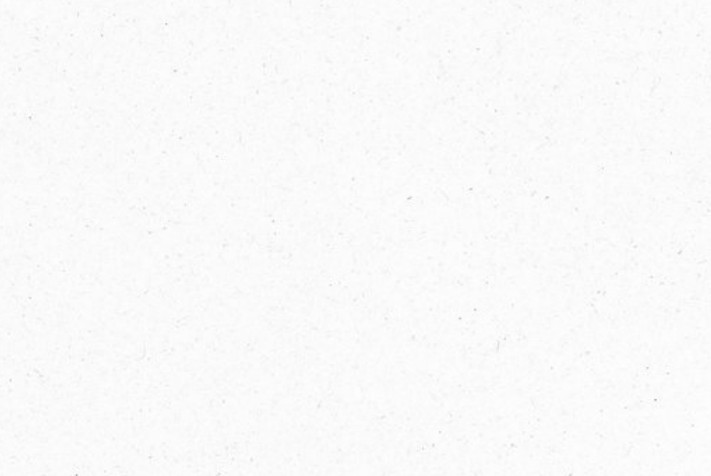
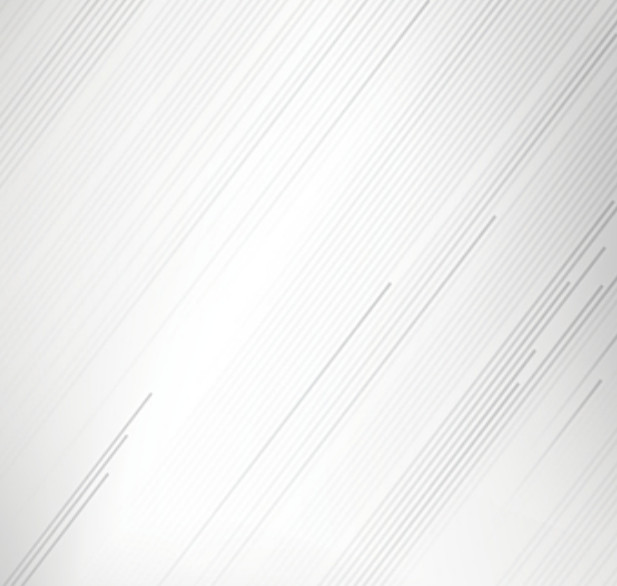
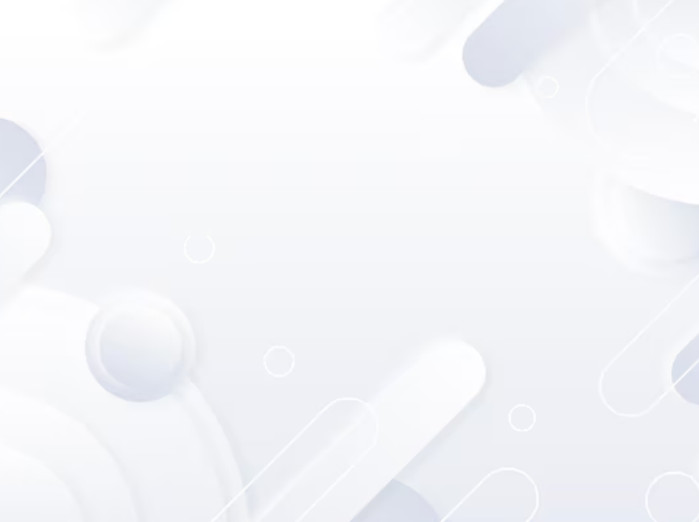
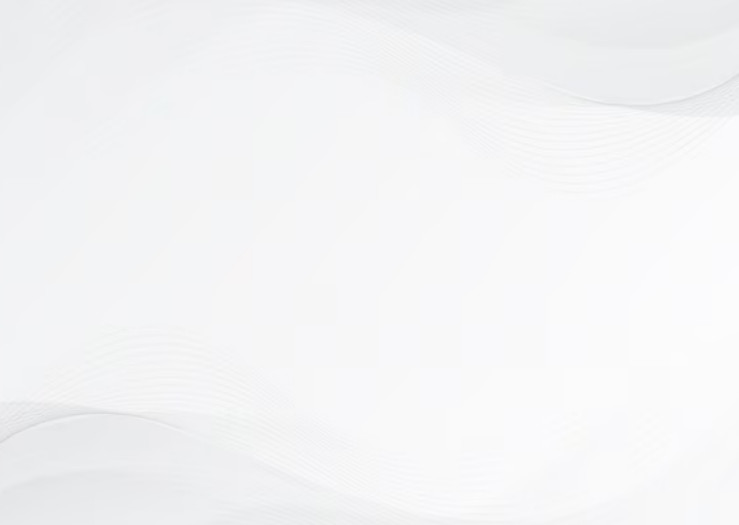
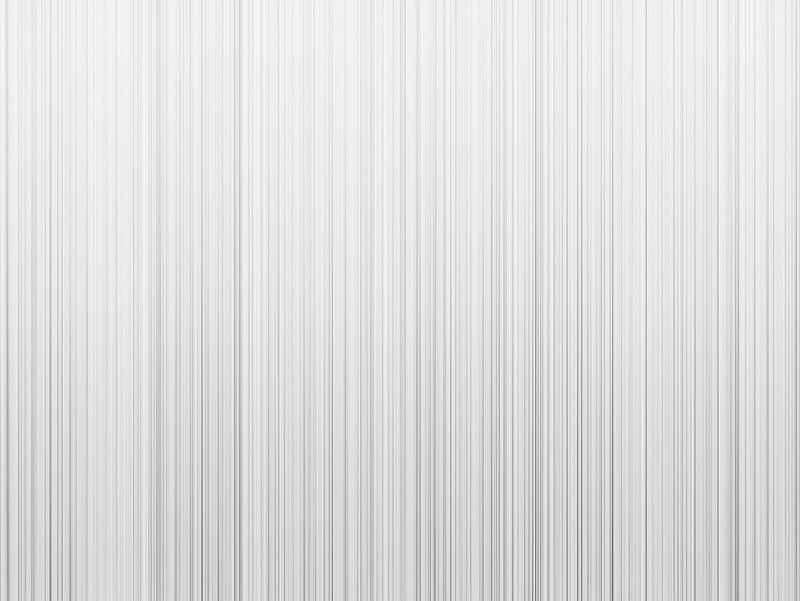
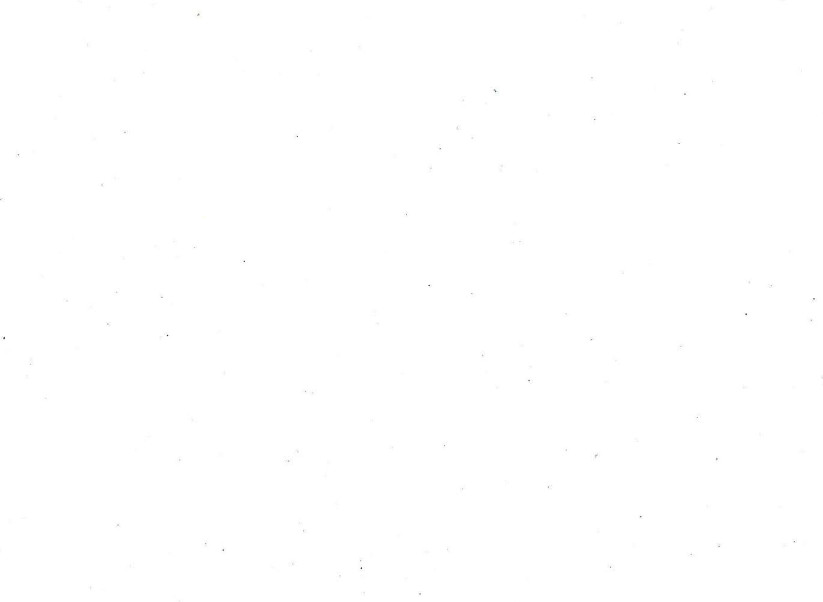
### 1. **Damage Assessment**
AI can analyze satellite imagery, aerial drone footage, and ground-level photographs to assess the extent of damage. Machine learning algorithms can be trained to identify and categorize affected areas, such as buildings, infrastructure, and natural landscapes, enabling rapid and accurate damage assessments.
– **Change Detection**: AI can compare pre- and post-disaster images to quantify changes in land use, structural integrity, and vegetation cover, providing valuable information for response teams.
### 2. **Resource Allocation**
AI can optimize resource allocation and logistics by analyzing real-time data on affected populations, infrastructure damage, and available resources. This ensures that supplies and aid are directed where they are most needed.
– **Predictive Modeling**: Machine learning models can predict community needs based on historical data and current conditions, allowing for proactive resource management.
### 3. **Impact Evaluation**
AI tools can evaluate the socioeconomic impacts of disasters by analyzing demographic data, economic indicators, and infrastructure loss. This analysis can inform policymakers about recovery needs and long-term planning.
– **Sentiment Analysis**: Natural language processing (NLP) can analyze social media posts and community feedback to gauge public sentiment and needs following a disaster.
### 4. **Vulnerability Assessment**
AI can help identify vulnerable populations and critical infrastructure at risk in future disasters. By integrating geographic information systems (GIS) with machine learning, communities can better understand vulnerabilities based on demographic data, historical disaster impacts, and environmental factors.
### 5. **Urban Planning and Resilience**
Post-disaster analysis can inform urban planning and development strategies to enhance resilience. AI can model different scenarios and assess the potential impacts of proposed infrastructure changes or disaster mitigation measures.
– **Scenario Simulation**: AI can simulate various disaster scenarios to help planners understand how different effective urban designs could mitigate future impacts.
### 6. **Workflow Automation**
AI can streamline post-disaster response workflows by automating data collection and analysis. This can speed up the assessment process and free up human resources for on-the-ground decision-making.
– **Automated Reporting**: AI systems can generate reports on damage assessments, recovery progress, and resource allocation, facilitating better communication among stakeholders.
### 7. **Integration of Multi-Source Data**
AI can integrate data from various sources, such as government agencies, NGOs, social media, and local reporting. This creates a comprehensive picture of the disaster’s impact and recovery efforts, leading to more informed decision-making.
### 8. **Monitoring Recovery Progress**
AI can track recovery progress over time by continuously analyzing data on rebuilding efforts, infrastructure restoration, and community well-being. This can help assess the effectiveness of recovery strategies and inform necessary adjustments.
### 9. **Training and Preparedness**
AI can be used to simulate disaster scenarios for training emergency responders and local authorities. These simulations can enhance preparedness by providing realistic training experiences, improving the skills and readiness of responders.
### 10. **Community Engagement**
AI can facilitate engagement with affected communities through chatbots and interactive platforms that collect feedback and provide information on recovery efforts. This can empower communities to play active roles in their recovery.
### Challenges and Considerations
While AI has significant potential for post-disaster analysis, it comes with challenges:
– **Data Quality and Availability**: The accuracy of AI analysis depends on the quality and comprehensiveness of the data available. In disaster situations, data can be sporadic or incomplete.
– **Ethical Considerations**: Ensuring the ethical use of data and privacy protection in AI applications is critical, particularly when dealing with sensitive information about affected individuals and communities.
– **Interdisciplinary Collaboration**: Effective post-disaster analysis requires collaboration between data scientists, disaster response teams, urban planners, and community leaders to ensure AI insights are actionable.
### Conclusion
AI has the potential to significantly improve post-disaster analysis, leading to faster recovery and better preparedness for future disasters. By incorporating AI into the response and recovery processes, communities can enhance their resilience, minimize damage, and support more effective rebuilding efforts.
Leave a Reply