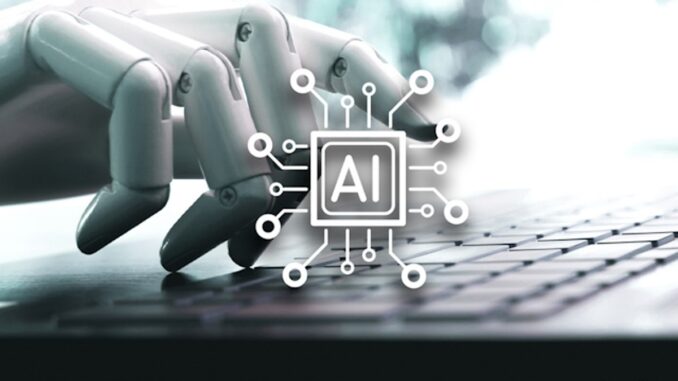
AI in volume analysis refers to the application of artificial intelligence techniques to understand and interpret the volume of data, whether in financial markets, big data analytics, or operational metrics. Here are some key areas where AI is utilized in volume analysis:
### 1. **Financial Markets** – **Trading Volume Analysis**: AI algorithms can analyze trading volumes to identify patterns that could indicate market trends. For instance, sudden increases in trading volume might signal a potential price movement, providing traders with insights for making buy or sell decisions.
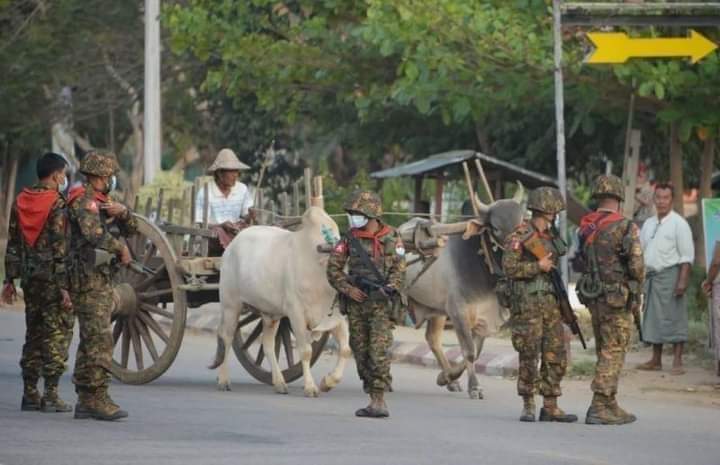
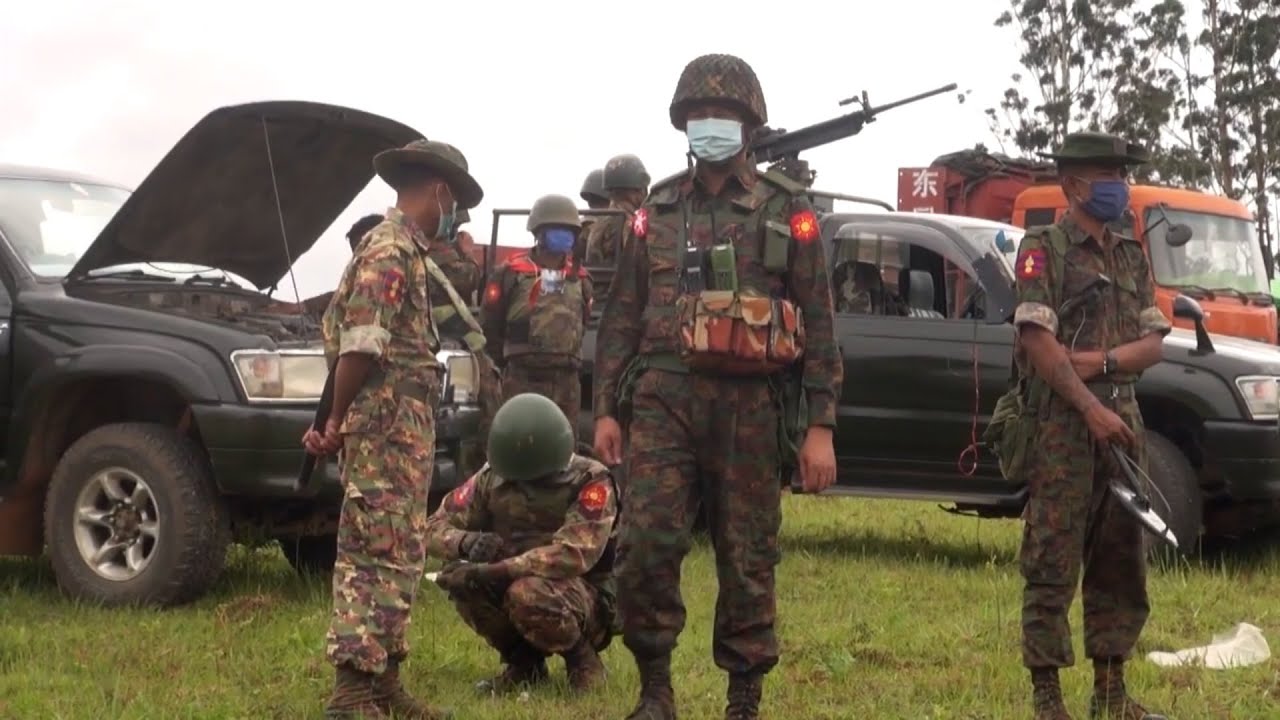
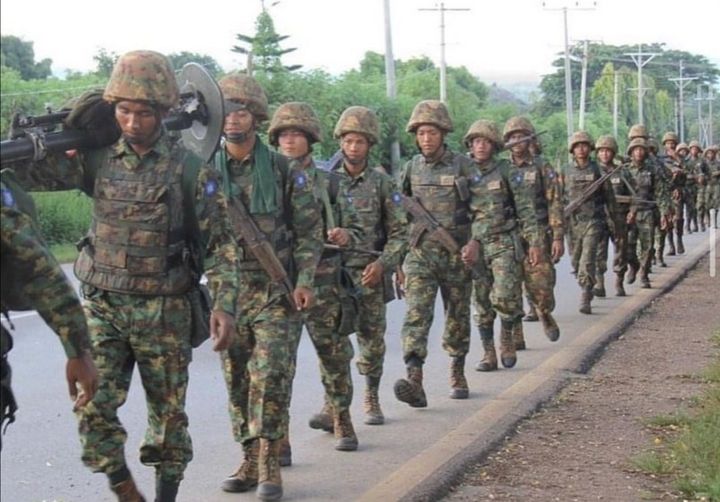
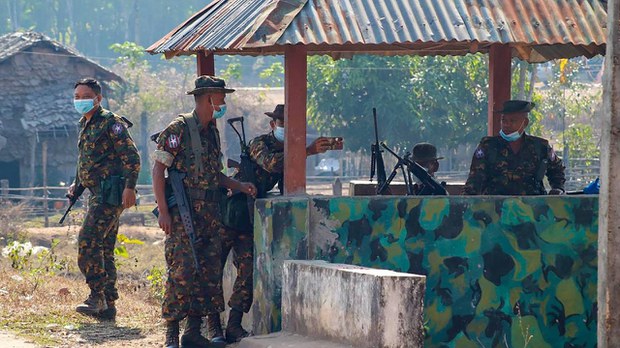
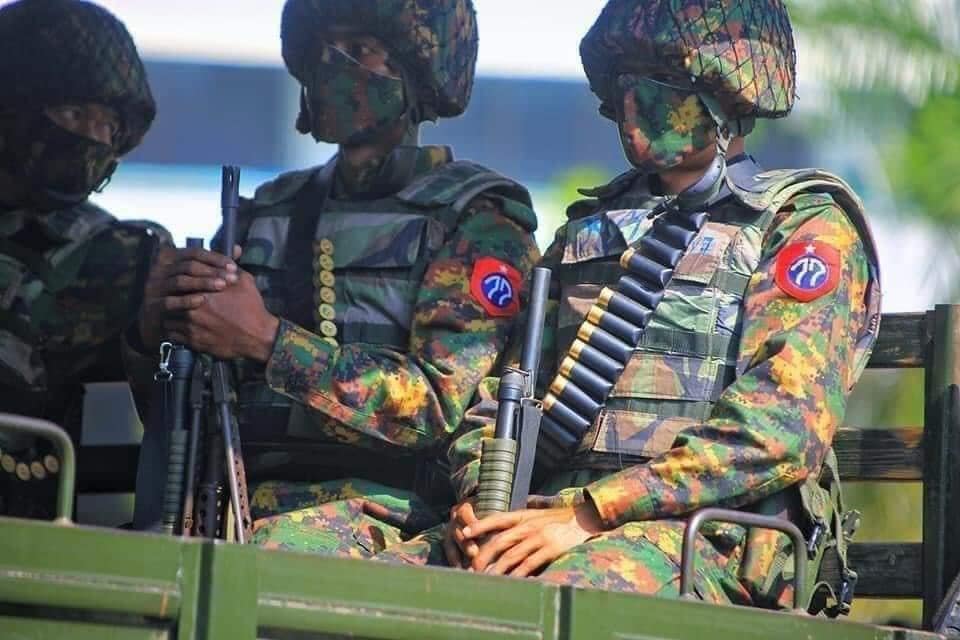
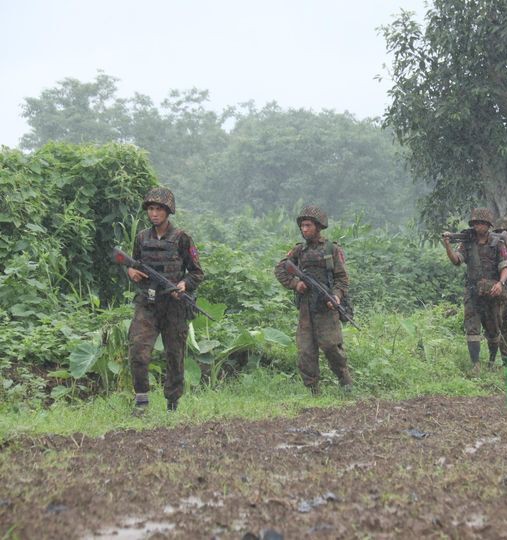
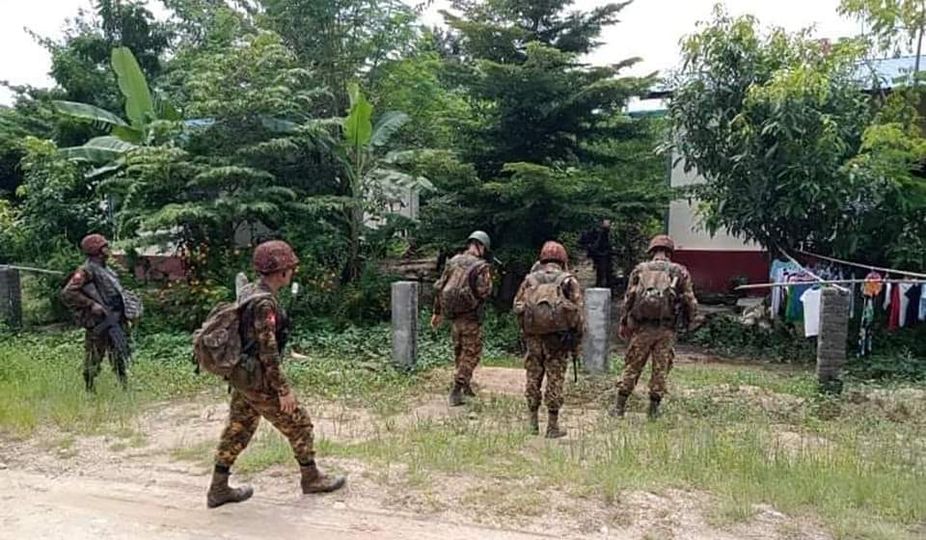
– **Predictive Analytics**: Machine learning models can be trained on historical trading volume data to forecast future volume trends and price changes, helping investors optimize their trading strategies.
### 2. **Big Data Analytics**
– **Data Processing**: AI can efficiently handle and analyze large volumes of data from various sources (e.g., social media, IoT devices, etc.), enabling organizations to extract actionable insights.
– **Pattern Recognition**: Algorithms can identify patterns or anomalies in large datasets, which could indicate opportunities or risks. For example, recognizing unusual spikes in customer behavior data can help businesses adjust their strategies.
### 3. **Customer Behavior Analysis**
– **Volume of Interactions**: Analyzing customer interaction volume across channels (e.g., website visits, social media engagement) can help businesses understand consumer preferences and trends.
– **Churn Prediction**: AI can analyze usage volumes to identify patterns associated with customer retention and attrition, enabling targeted interventions fo
r reducing churn rates.
### 4. **Supply Chain and Inventory Management**
– **Demand Forecasting**: AI models can analyze historical sales volume data to predict future demand, helping companies optimize inventory levels and reduce waste.
– **Optimization Algorithms**: By analyzing product volume data across the supply chain, AI can recommend adjustments in logistics and inventory management to enhance efficiency.
### 5. **Healthcare Analytics**
– **Patient Volume Analysis**: AI can analyze patient volume trends in hospitals and clinics to optimize staff allocation and resource management.
– **Predicting Health Trends**: Patterns in health data volumes can help in predicting outbreaks, patient admissions, and other trends crucial for public health planning.
### 6. **Manufacturing and Production**
– **Operational Efficiency**: AI can analyze production volume data to identify bottlenecks and inefficiencies in manufacturing processes, allowing for better resource allocation and production scheduling.
– **Quality Control**: By examining the volume of produced versus defective items, AI can help identify quality issues in manufacturing.
### Tools and Techniques
– **Machine Learning Algorithms**: Supervised and unsupervised learning methods (like regression, clustering, and neural networks) are commonly used to find patterns in volume data.
– **Natural Language Processing (NLP)**: Used for analyzing text data volume from customer feedback or social media to gauge sentiment or trends.
– **Time Series Analysis**: Techniques specifically designed for analyzing sequential data, useful in cases where volume changes over time, such as stock prices or sales figures.
### Conclusion
AI greatly enhances the capabilities of volume analysis by providing tools to process large datasets, uncover patterns, and generate predictive insights. By leveraging these advanced technologies, organizations across various sectors can improve decision-making, enhance operational efficiency, and drive strategic initiatives. If you have a specific application or industry in mind, I can provide more targeted insights or examples!
Leave a Reply