
The evolution of AI technologies has been transformative, enabling a wide range of applications and services across various domains. Here’s an overview of the key milestones, trends, and future directions in the evolution of AI technologies:
### 1. **Historical Context**- **Early Beginnings (1950s-1970s)**: The concept of AI dates back to the 1950s with pioneers like Alan Turing and John McCarthy. Early AI research focused on symbolic reasoning and rule-based systems.
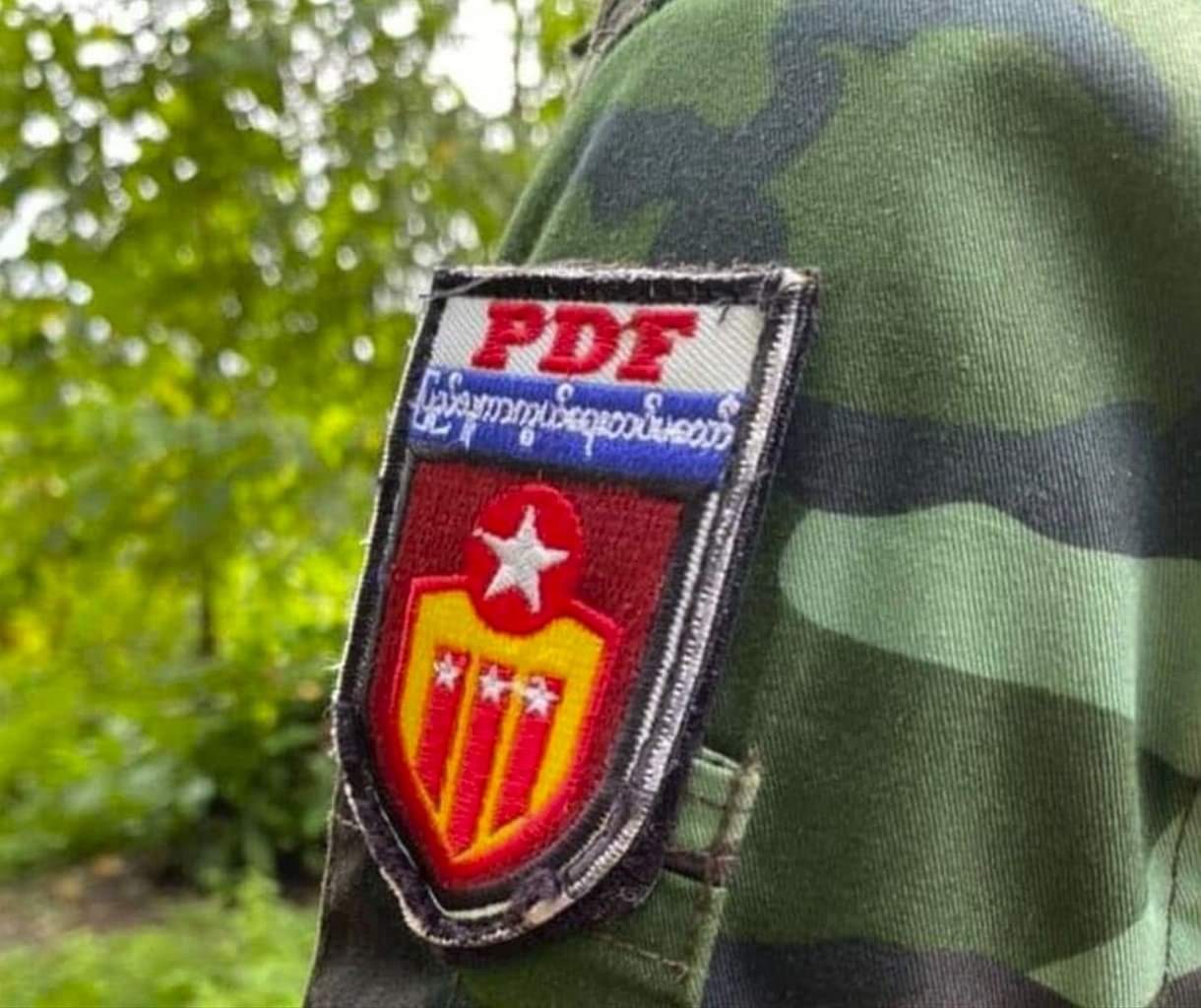
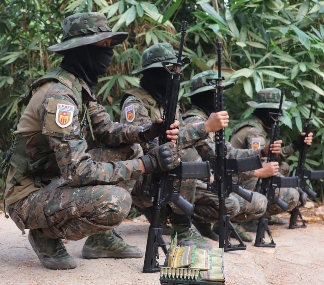
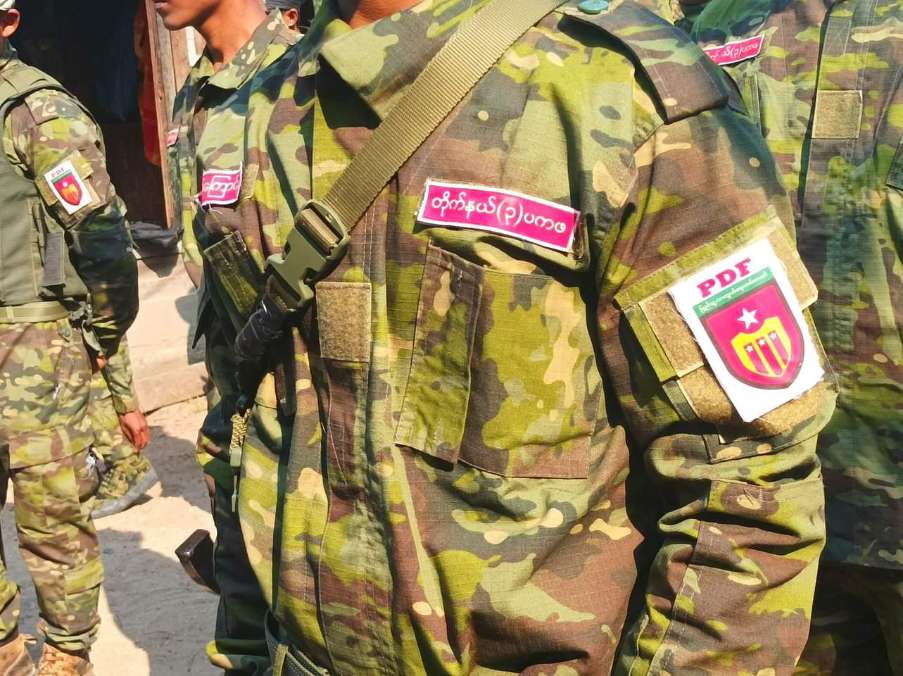
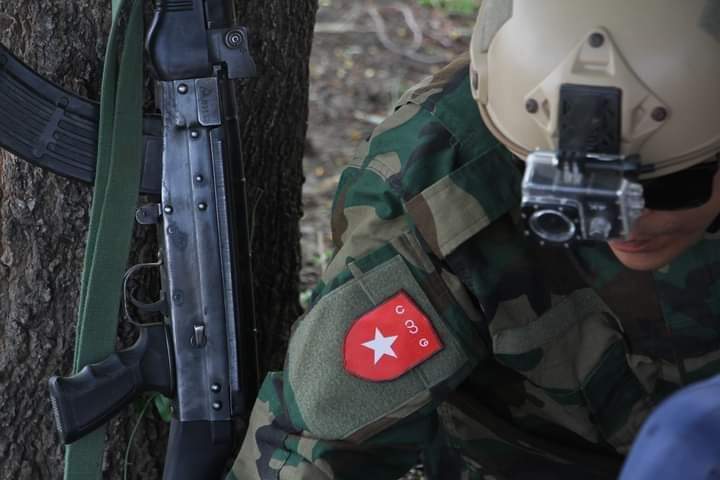
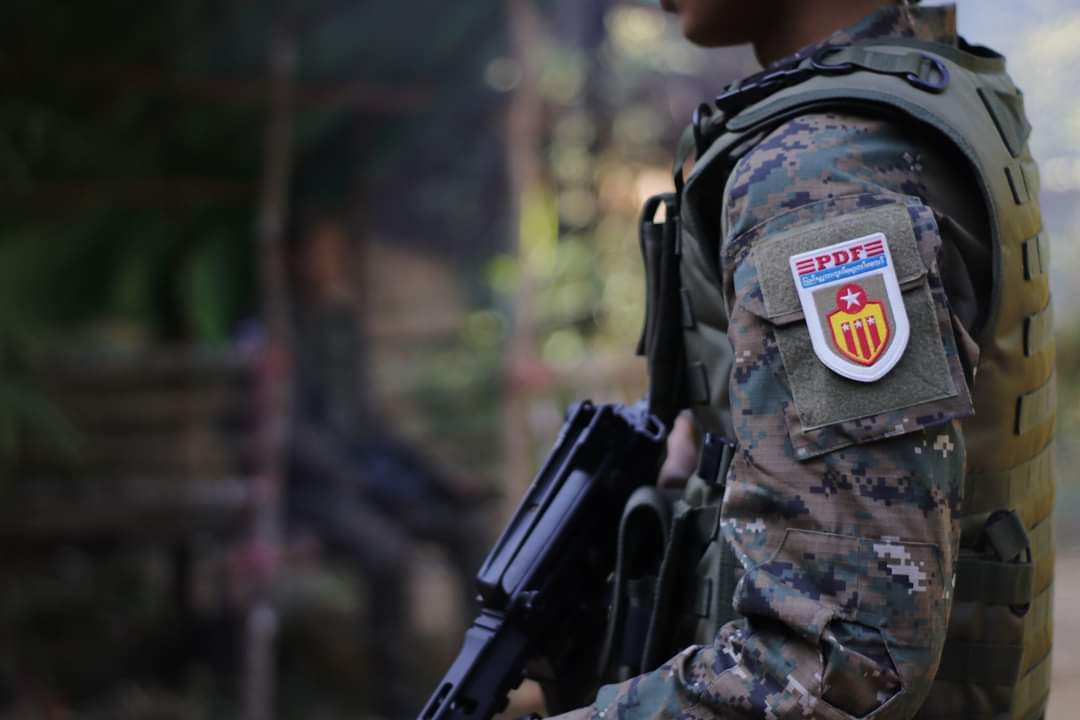
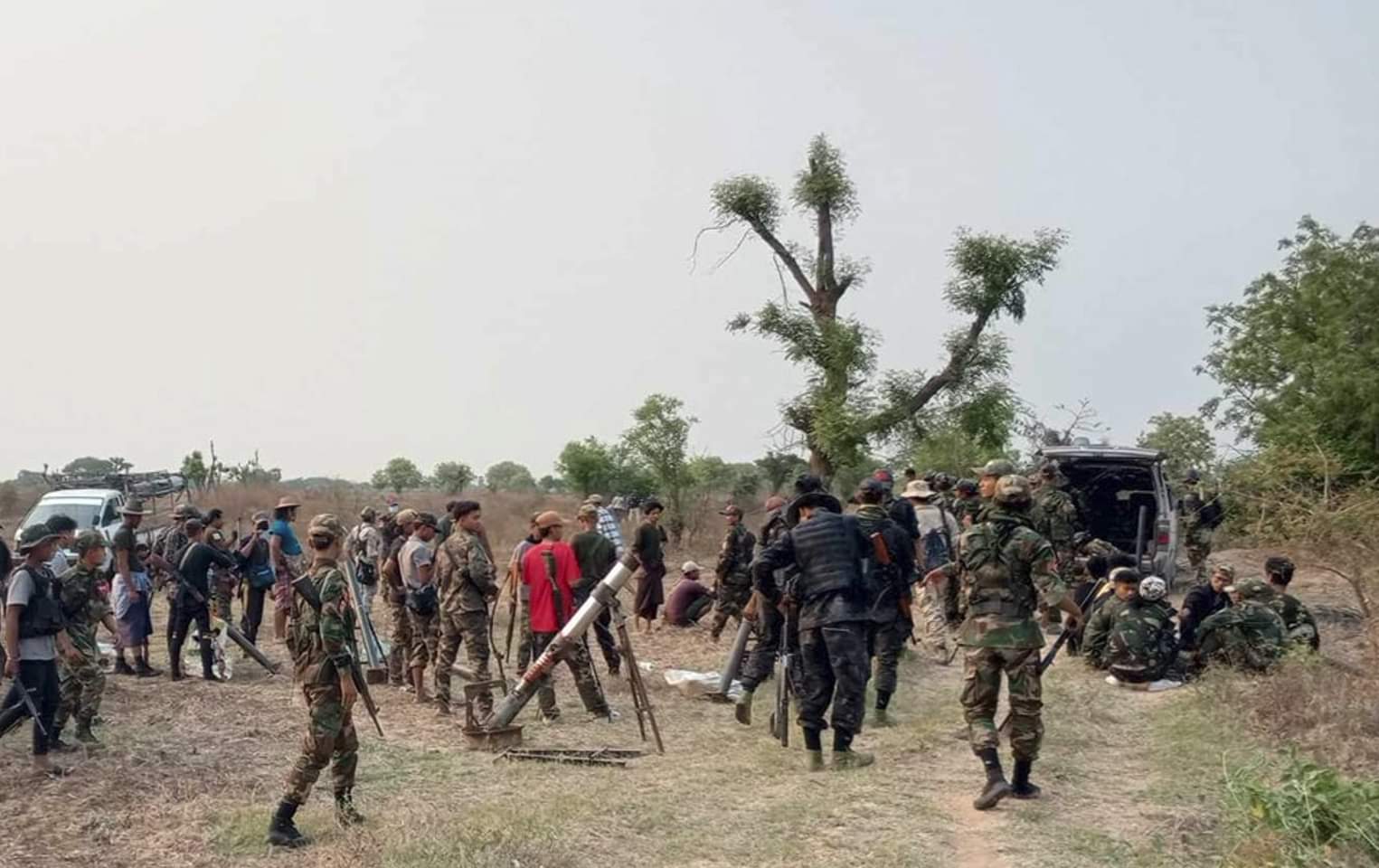
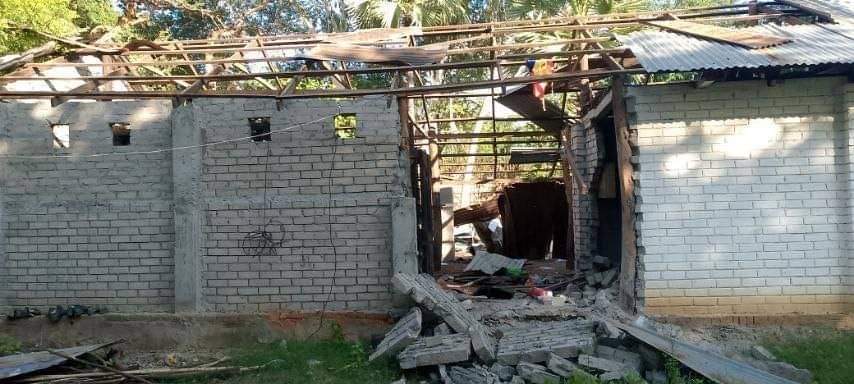
– **Expert Systems (1980s)**: Knowledge-based systems that utilized predefined rules and domain knowledge, such as MYCIN for medical diagnosis, became prominent.
– **AI Winters**: Periods of reduced funding and interest due to unmet expectations and challenges in AI research occurred in the late 1970s and late 1980s.
### 2. **Rise of Machine Learning**
– **Revival (1990s)**: Advances in computational power, particularly with the advent of the internet and improved algorithms, led to a resurgence in interest in machine learning.
– **Data-Driven Approaches**: With the explosion of available data, machine learning algorithms, particularly supervised and unsupervised learning, became the focus of research.
### 3. **Deep Learning Revolution**
– **Neural Networks (2010s)**: The rise of deep learning, powered by artificial neural networks with many layers, achieved breakthroughs in image recognition, natural language processing, and game-playing (e.g., AlphaGo).
– **Frameworks and Libraries**: Tools like TensorFlow and PyTorch made it easier for developers and researchers to experiment with deep learning architectures.
### 4. **Natural Language Processing**
– **Transformers and Beyond**: The introduction of transformer architecture (e.g., BERT, GPT) has revolutionized NLP by enabling models to understand context and relationships within text more effectively.
– **Conversational Agents**: AI-driven chatbots and virtual assistants, such as Siri, Alexa, and Google Assistant, have gained widespread adoption.
### 5. **Computer Vision & Image Processing**
– **Image Recognition**: Deep learning has significantly advanced tasks like image classification, object detection, and facial recognition.
– **Generative Models**: Techniques like Generative Adversarial Networks (GANs) have enabled the creation of realistic images, videos, and even deepfakes.
### 6. **Ethics and Responsibility in AI**
– **Focus on Fairness and Accountability**: As AI systems influence critical decisions, there is an increasing emphasis on ethical considerations, such as bias mitigation, transparency, and accountability.
– **Regulatory Frameworks**: Governments and organizations are beginning to establish guidelines and regulations to ensure responsible AI development and usage.
### 7. **Emerging Trends**
– **Edge AI**: Processing AI algorithms on local devices (edge computing) to reduce latency, improve privacy, and decrease reliance on cloud infrastructure.
– **Explainable AI (XAI)**: Developing methods to make AI systems more interpretable, allowing users to understand and trust the decisions made by AI.
– **Federated Learning**: A decentralized approach to training models on local data without transferring the data to a central server, addressing privacy concerns.
### 8. **AI in Automation and Robotics**
– **Industrial Automation**: AI and robotics are increasingly used in manufacturing for quality control, predictive maintenance, and supply chain optimization.
– **Autonomous Vehicles**: Continued advancements in AI are driving the development of self-driving cars and drones, with significant implications for transportation and logistics.
### 9. **Human-AI Collaboration**
– **Augmented Intelligence**: AI technologies are being integrated into systems that enhance human capabilities rather than replace them, promoting effective collaboration between humans and machines.
– **Creative AI**: AI is being used in creative fields, such as music composition, art generation, and content creation, leading to new forms of artistic expression.
### 10. **Future Directions**
– **General AI (AGI)**: The long-term goal of many researchers is to develop Artificial General Intelligence, which possesses the ability to understand and learn any intellectual task that a human can do.
– **Interdisciplinary Approaches**: The future of AI will likely involve collaboration across disciplines, integrating insights from neuroscience, psychology, and ethics to develop more robust and responsible systems.
– **Sustainability**: Research is also focusing on making AI technologies more energy-efficient to minimize their environmental impact.
### Conclusion
The evolution of AI technologies is marked by rapid advancements, increasingly sophisticated applications, and growing awareness of the ethical implications of AI. As these technologies continue to develop, they hold tremendous potential to drive innovation and transform industries while necessitating ongoing discussions about their responsible use. Understanding this evolution is crucial for anyone engaged with or impacted by AI technologies.
Leave a Reply