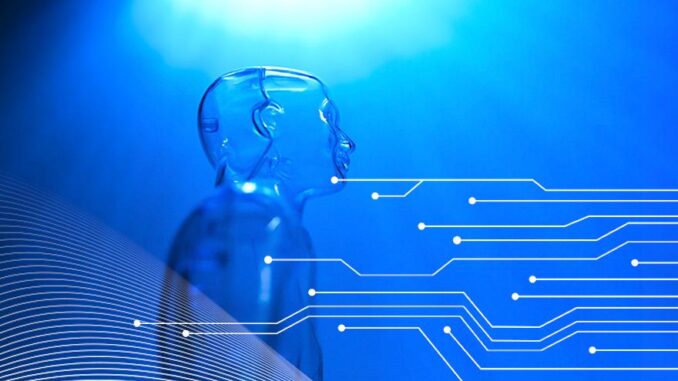
Understanding regulatory frameworks for AI (artificial intelligence) involves examining the guidelines, laws, and policies that govern the use, development, and deployment of AI technologies.
As AI applications become increasingly prevalent across various sectors, several key considerations and components emerge in the regulatory landscape:
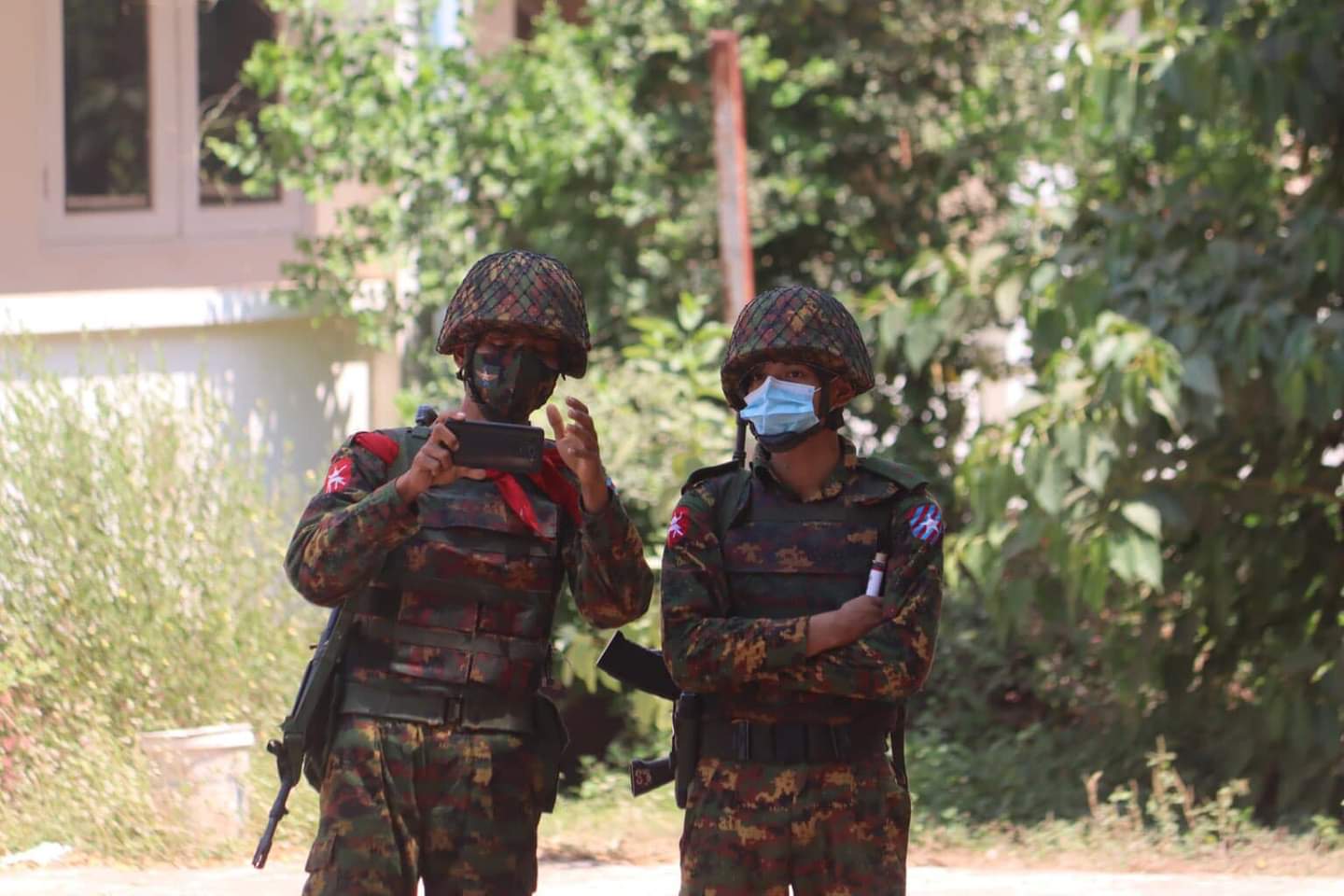

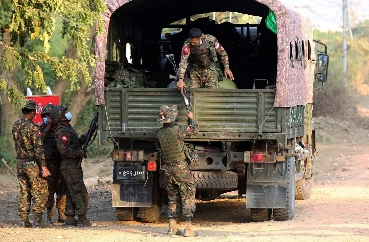
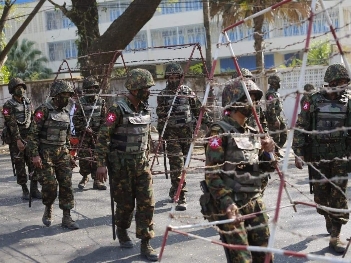
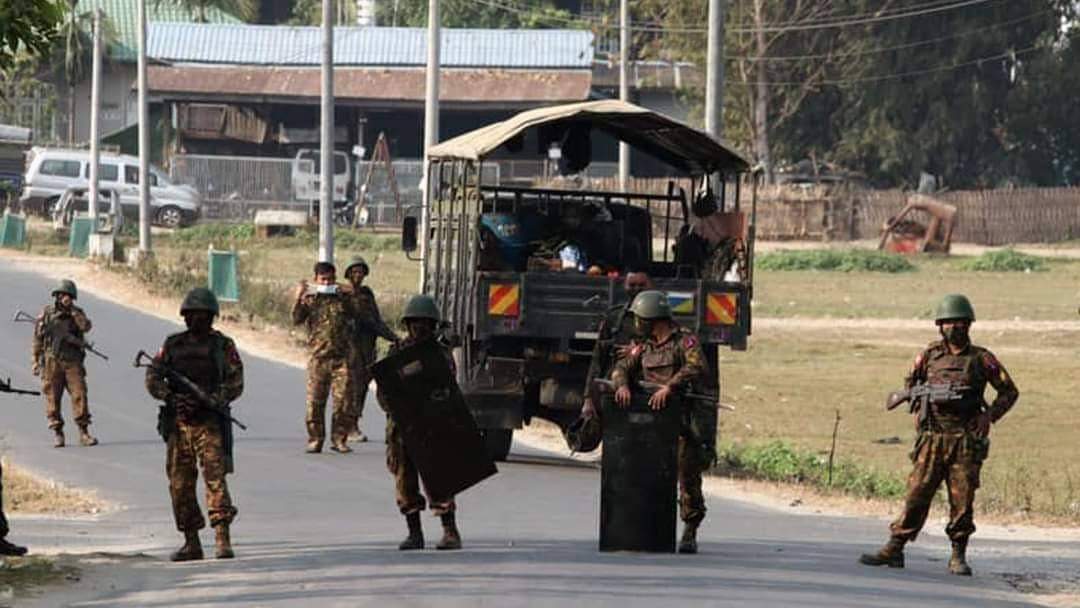
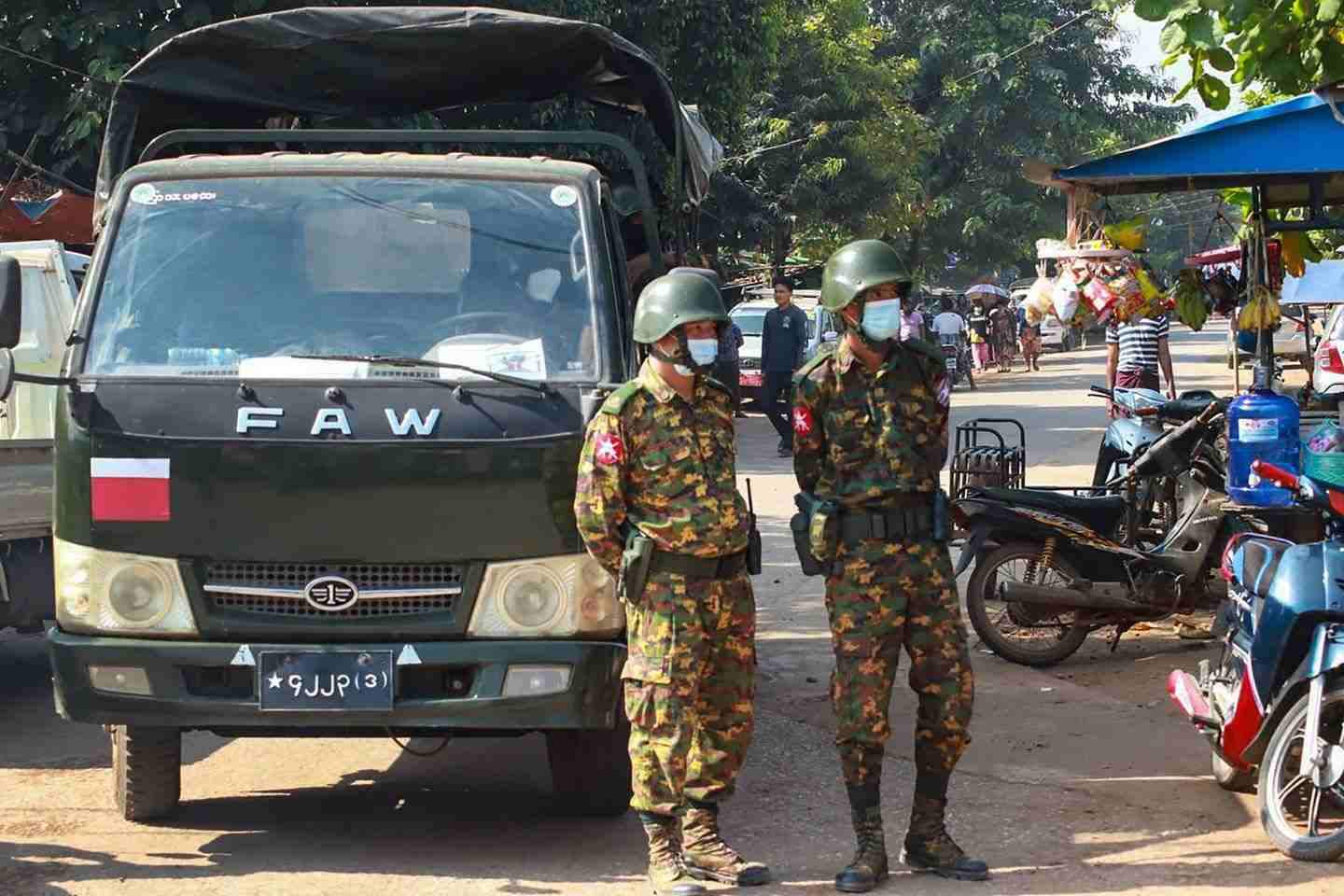
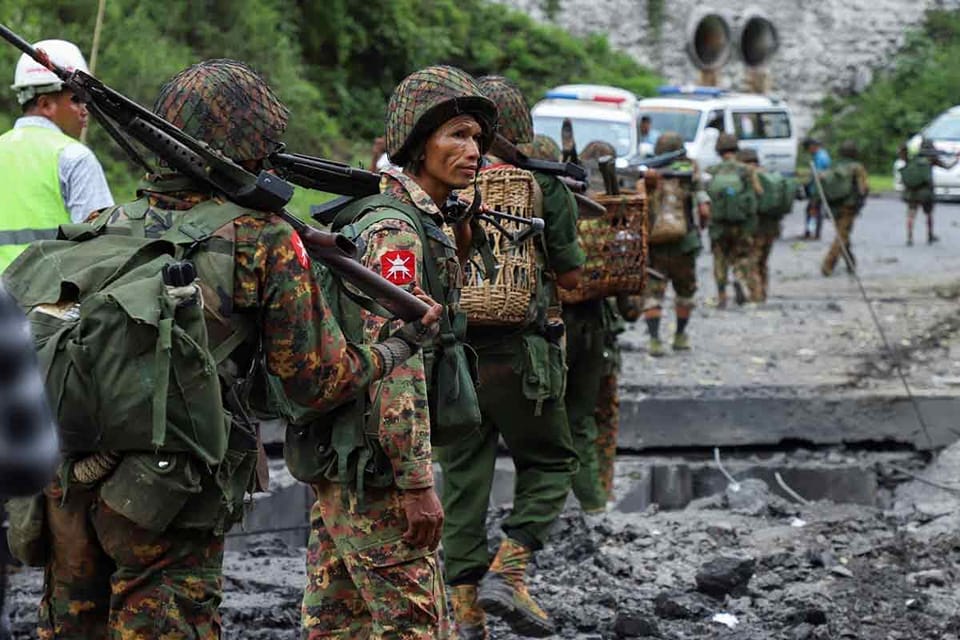
### 1. **Global Initiatives and Agreements**
– Various international bodies like the European Union, OECD, and UNESCO are working on establishing global norms and recommendations for AI governance.
– The **European Union AI Act** is one of the most comprehensive regulatory proposals, categorizing AI systems by risk (unacceptable, high risk, limited risk, and minimal risk) and establishing corresponding obligations.
### 2. **Risk Assessment**
– Regulatory frameworks often emphasize the importance of risk assessment for AI technologies. High-risk AI applications may be subject to more stringent controls, including:
– Transparency requirements
– Data governance rules
– Robustness and accuracy criteria
– Pre-market assessments
### 3. **Transparency and Explainability**
– Regulations are increasingly calling for transparency in AI algorithms, particularly in how decisions are made. This is particularly critical in high-stakes areas like healthcare, finance, and criminal justice.
– Explainability mandates require that AI systems provide understandable justifications for their outputs.
### 4. **Data Privacy and Protection**
– Compliance with data protection laws, such as the **General Data Protection Regulation (GDPR)** in the EU and the **California Consumer Privacy Act (CCPA)** in the U.S., is essential in AI regulation.
– These laws govern how data is collected, stored, and used, emphasizing user consent and data minimization principles.
### 5. **Bias and Fairness**
– Addressing bias in AI is a critical component of regulatory frameworks. Regulations may mandate:
– Regular audits of AI systems to detect and mitigate bias
– Guidelines for data collection to ensure representativeness
– Fairness in AI outcomes is necessary to avoid discrimination against specific groups.
### 6. **Accountability and Liability**
– Determining accountability for AI-driven decisions is an evolving area. Regulatory frameworks may establish:
– Liability regimes for harm caused by autonomous systems
– Designated accountability for AI developers and users
### 7. **Ethical Considerations**
– Many regulatory frameworks incorporate ethical guidelines for AI development and use, emphasizing principles such as:
– Human oversight
– Non-maleficence (avoiding harm)
– Beneficence (promoting well-being)
### 8. **Compliance and Enforcement**
– Regulations need to be enforceable, which may involve creating regulatory bodies to monitor compliance, investigate violations, and impose penalties.
– Businesses using AI will typically need to conduct impact assessments and maintain documentation to demonstrate compliance.
### 9. **Innovation and Economic Considerations**
– Balancing regulation with the need to encourage innovation is a critical challenge. Policymakers must ensure that regulations do not stifle technological advancement while still protecting public interest.
### 10. **Stakeholder Engagement**
– Involving a diverse range of stakeholders— including industry, academia, civil society, and the public—in the regulatory process is crucial. This can help ensure that regulations are well-informed and consider various perspectives and potential impacts.
### Conclusion
Understanding the regulatory frameworks for AI is essential for developers, organizations, and stakeholders involved in AI technologies. As regulations continue to evolve, keeping abreast of changes and best practices will be vital for compliance and responsible innovation in the AI landscape.
Leave a Reply