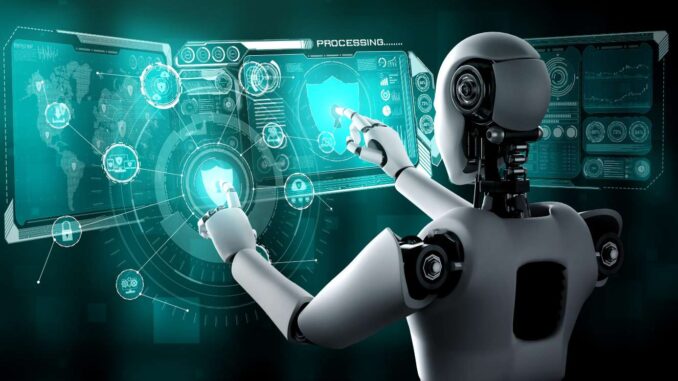
The development of technical AI standards is a critical endeavor aimed at ensuring that artificial intelligence systems are safe, reliable, ethical, and interoperable. Here are the key aspects and steps involved in developing these standards:
### 1. **Stakeholder Engagement** – **Involvement of Various Parties**: Include governments, industry leaders, researchers, and civil society organizations in the conversation to make sure diverse perspectives are represented.
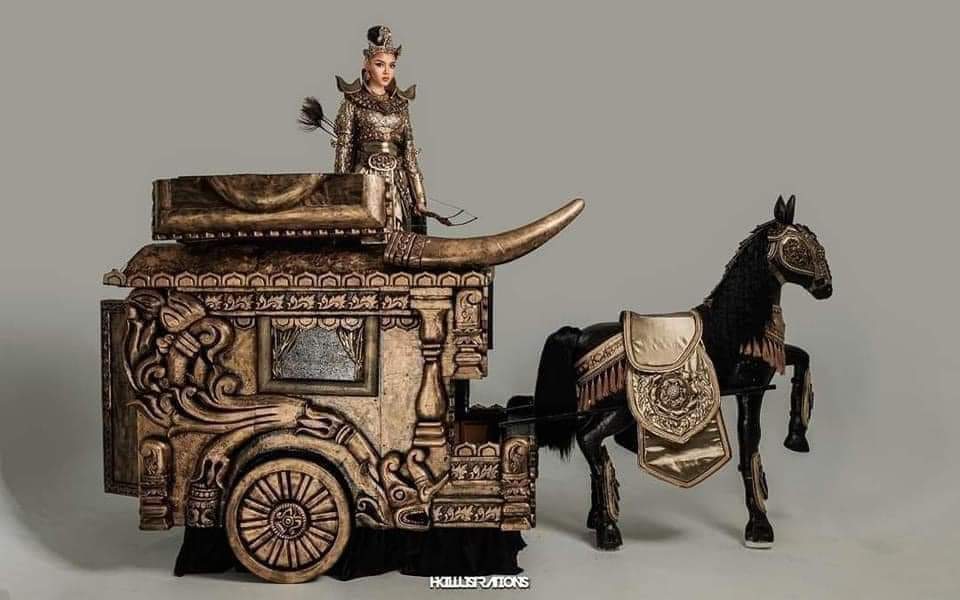
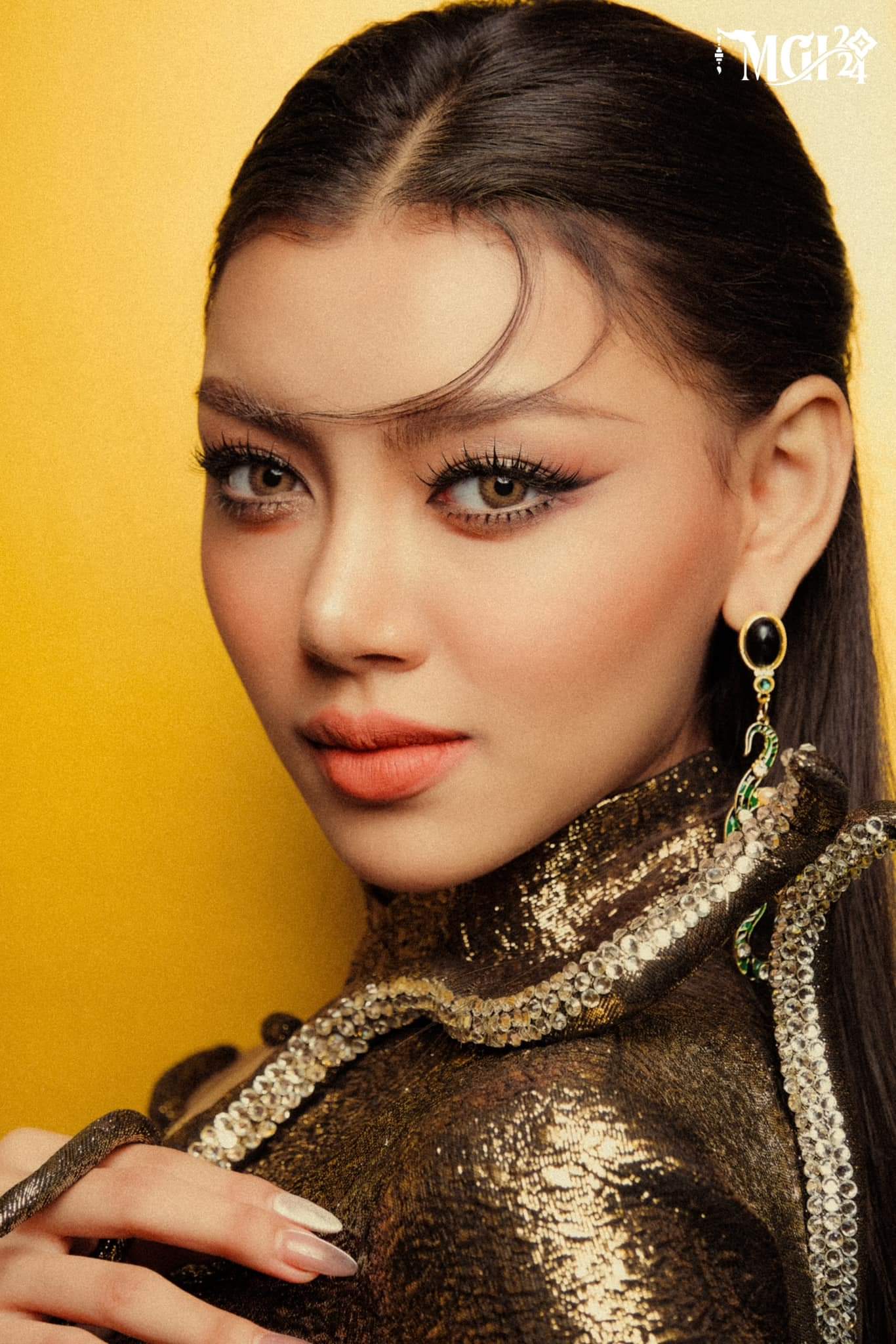
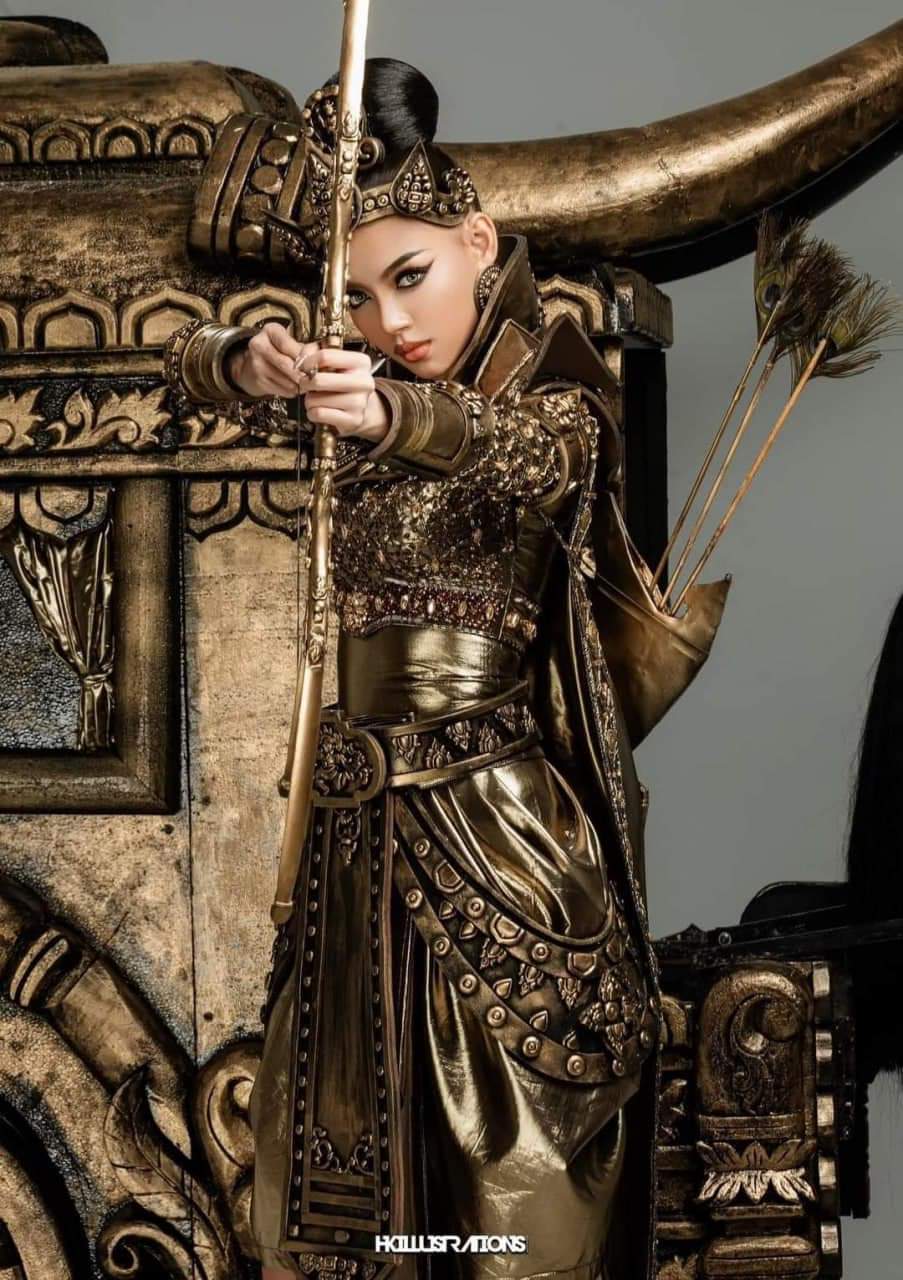
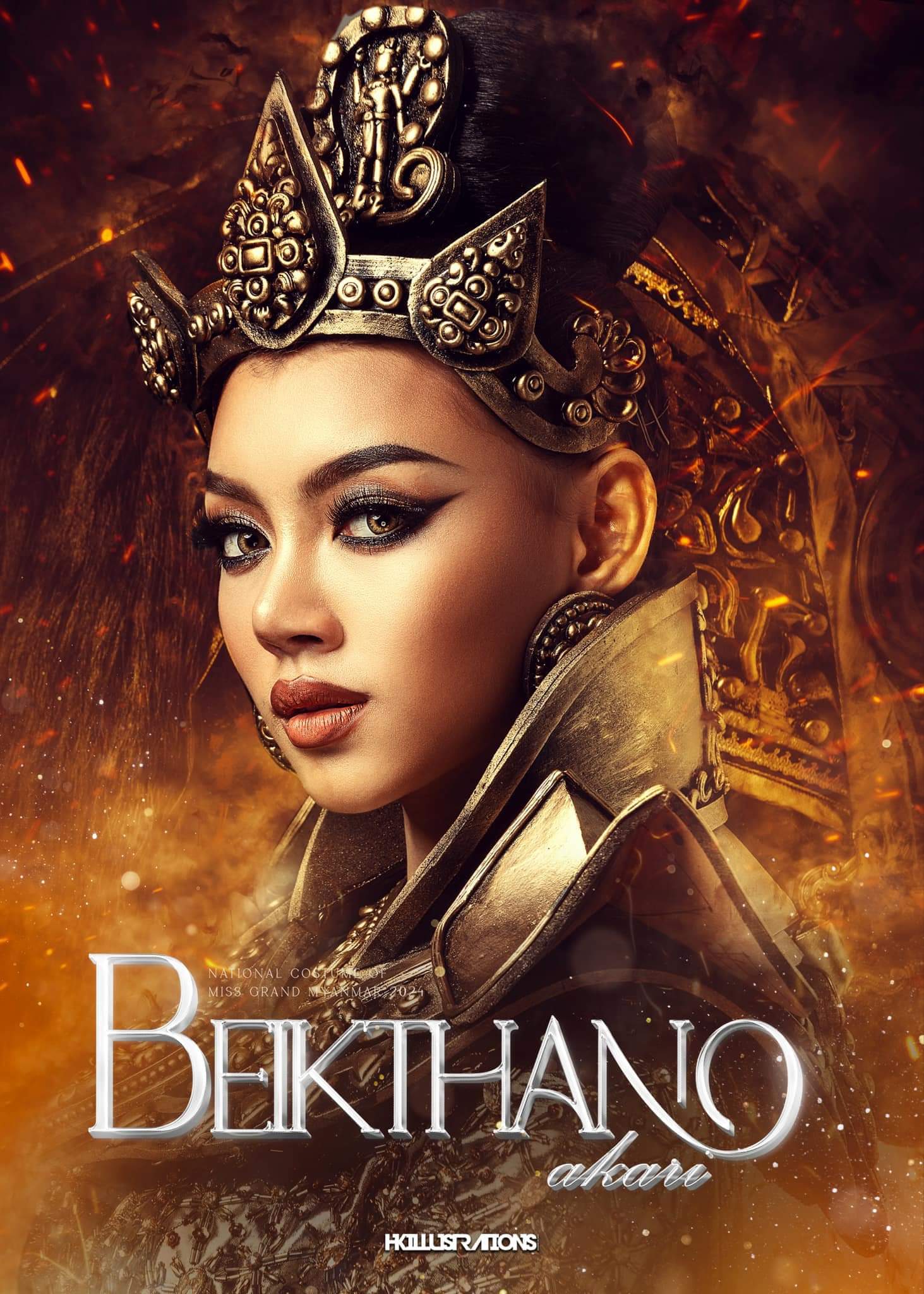
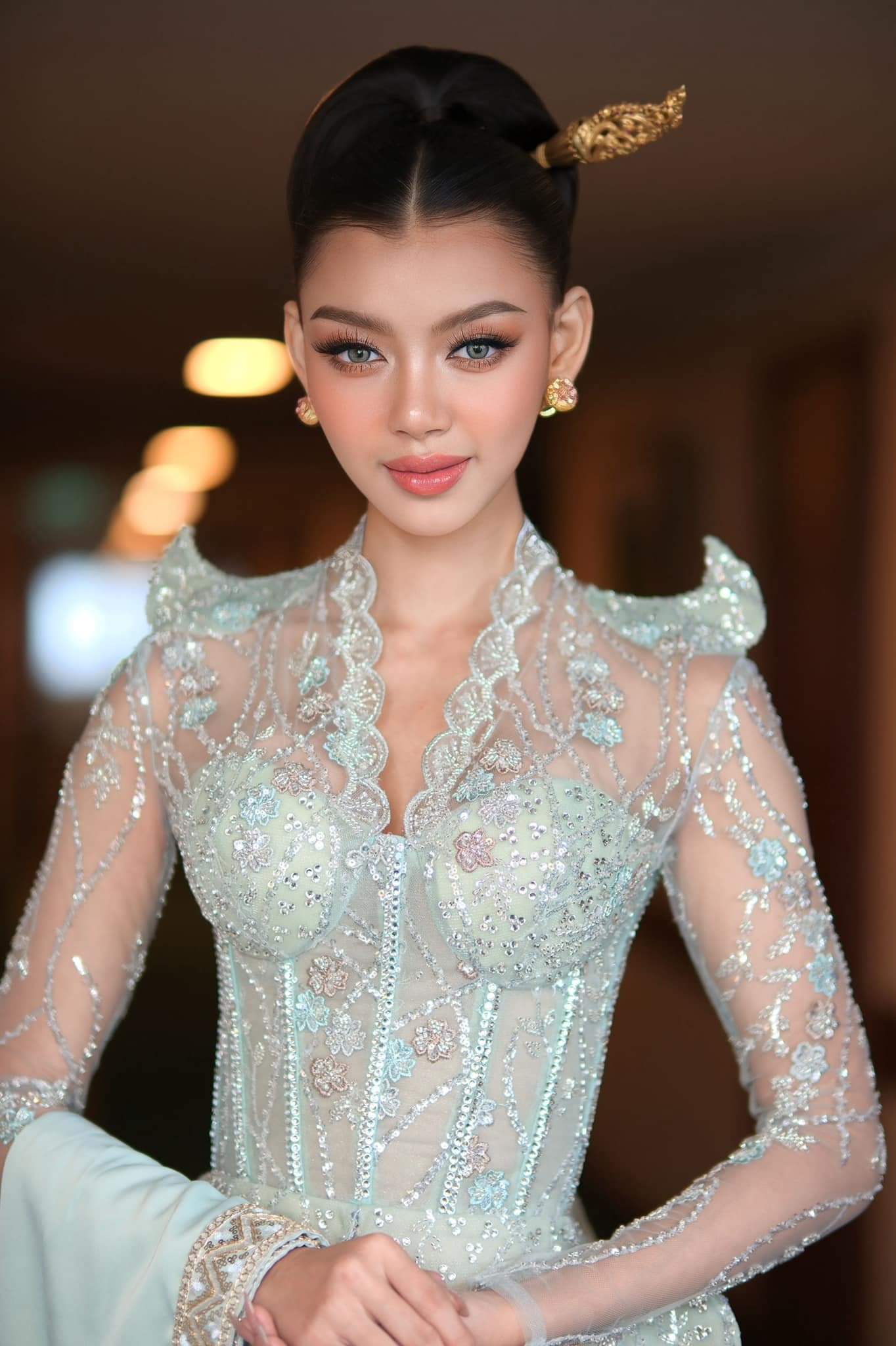
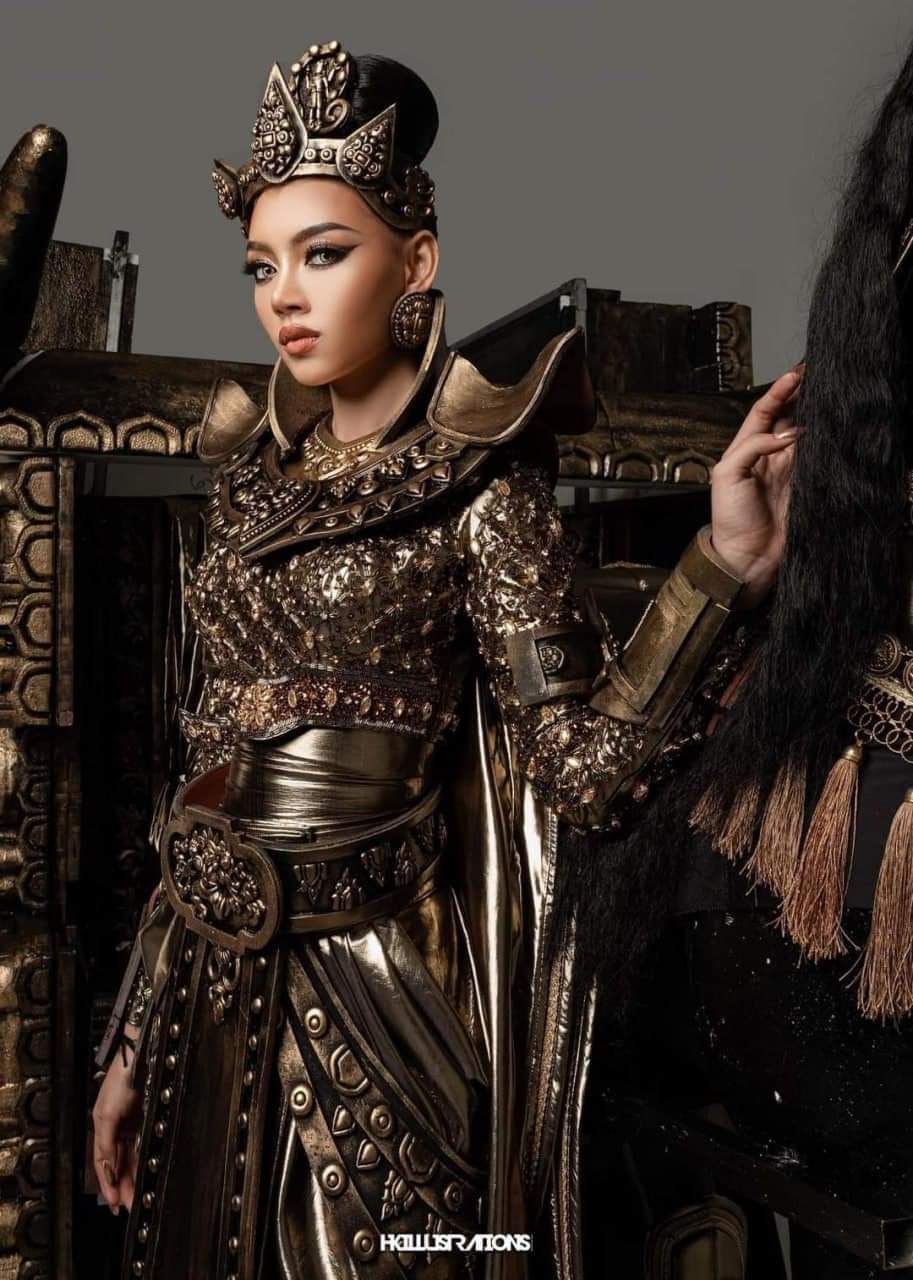
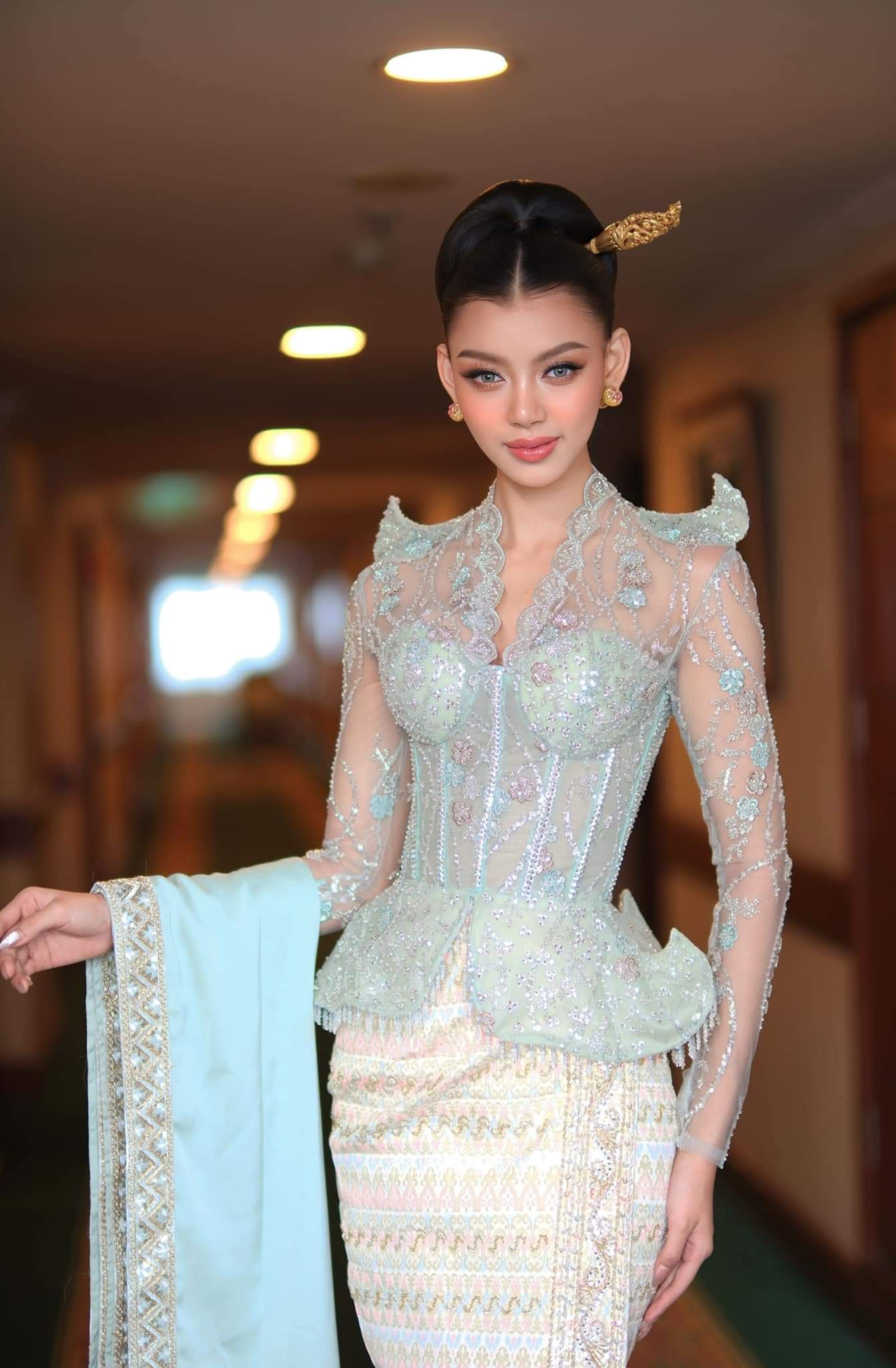
– **Public Consultations**: Foster dialogue with the public and user organizations to gather input and ensure the standards meet societal needs.
### 2. **Framework Establishment**
– **Defining Objectives**: Establish clear objectives for the standards, such as enhancing safety, promoting transparency, encouraging fairness, and ensuring accountability.
– **Scope and Applicability**: Decide the sectors and types of AI technologies that the standards will cover (e.g., machine learning, robotics, natural language processing).
### 3. **Technical Specifications**
– **Performance Metrics**: Develop concrete metrics for evaluating AI performance, including accuracy, efficiency, and robustness.
– **Interoperability Standards**: Create specifications that promote compatibility among different AI systems and data formats.
– **Security Standards**: Address cybersecurity concerns, focusing on data protection and secure handling of AI systems.
### 4. **Ethical Guidelines**
– **Bias Mitigation**: Establish procedures for detecting and mitigating bias in AI algorithms and datasets.
– **Fairness and Accountability**: Define guidelines to ensure equitable treatment of all users, and establish accountability mechanisms for AI decisions.
– **Transparency and Explainability**: Mandate features that allow users to understand how AI systems make decisions.
### 5. **Regulatory Alignment**
– **Global Collaboration**: Work with international organizations (like ISO, IEC, IEEE) to align standards globally, facilitating international cooperation and trade.
– **Compliance Framework**: Ensure that standards are compatible with existing and emerging regulations governing data protection, privacy, and AI.
### 6. **Implementation Guidelines**
– **Best Practices**: Develop detailed guidelines for the implementation of AI systems in various sectors.
– **Training and Certification**: Offer training programs and certification for developers and users to ensure they understand the standards and can apply them.
### 7. **Continuous Review and Update**
– **Feedback Mechanisms**: Establish channels for ongoing feedback from stakeholders to ensure standards remain relevant.
– **Periodic Reviews**: Commit to regular updates of the standards in response to rapid technological advancements and emerging challenges in the AI landscape.
### 8. **Awareness and Advocacy**
– **Education Initiatives**: Promote awareness and understanding of AI standards among developers, businesses, and consumers.
– **Industry Collaboration**: Encourage collaboration among industry players to build consensus and foster a culture of compliance with technical standards.
### Conclusion
Developing technical AI standards is an iterative process that requires continuous collaboration, research, and adaptation. The goal is to foster an environment where AI technologies can be trusted, understood, and beneficial to society, while minimizing risks and maximizing potential.
Leave a Reply