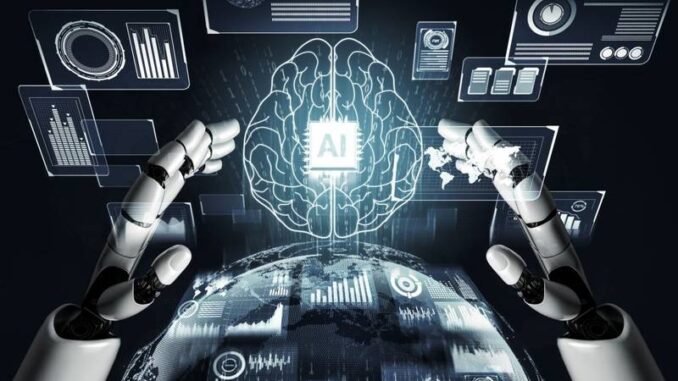
Advancing trustworthy AI technologies involves developing systems that are safe, reliable, transparent, and aligned with human values. Here are several key areas and strategies for achieving this:
### 1. **Robustness and Reliability** – **Adversarial Robustness**: Designing AI systems that are resilient to adversarial attacks and can maintain performance in the face of unexpected inputs.
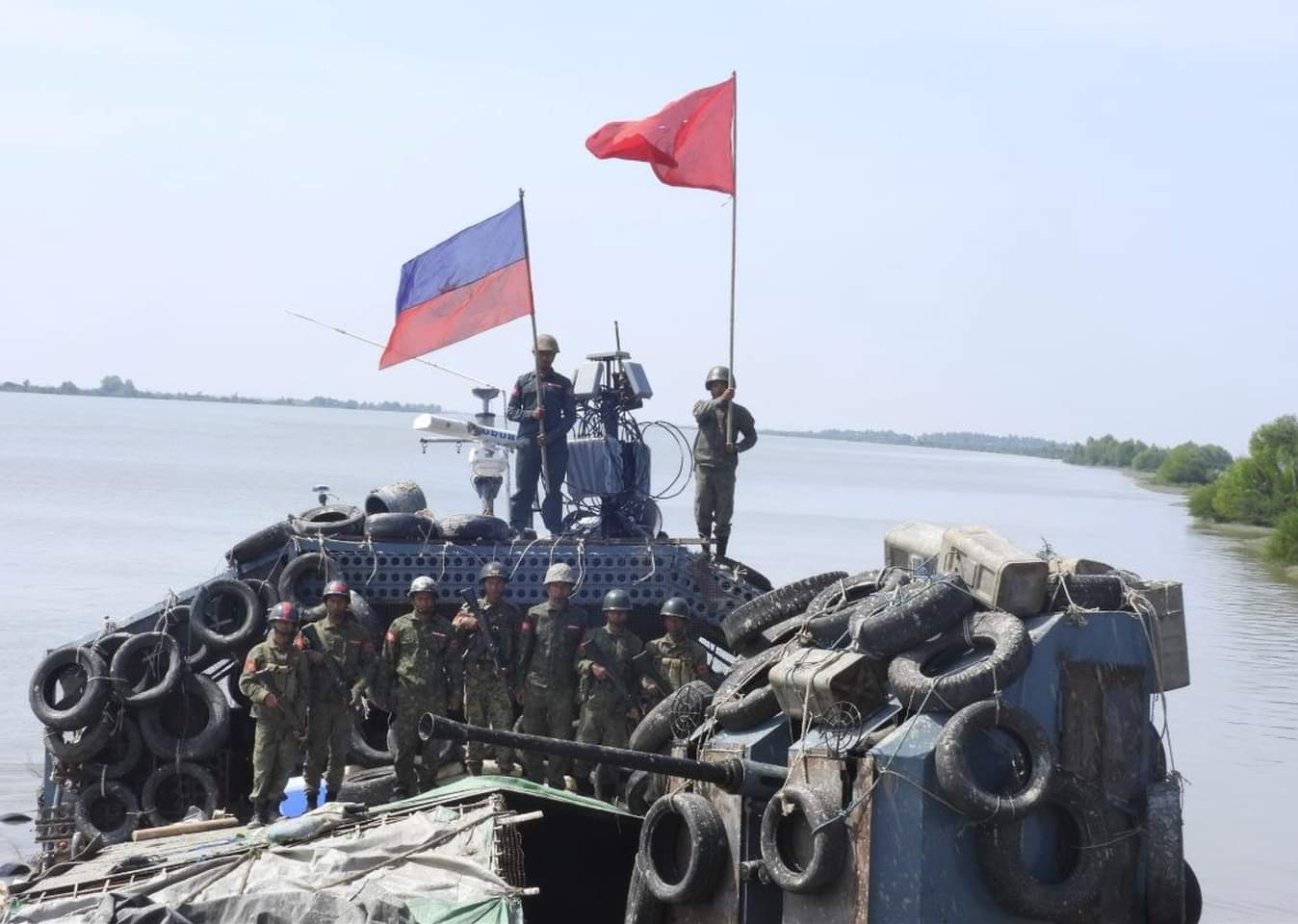
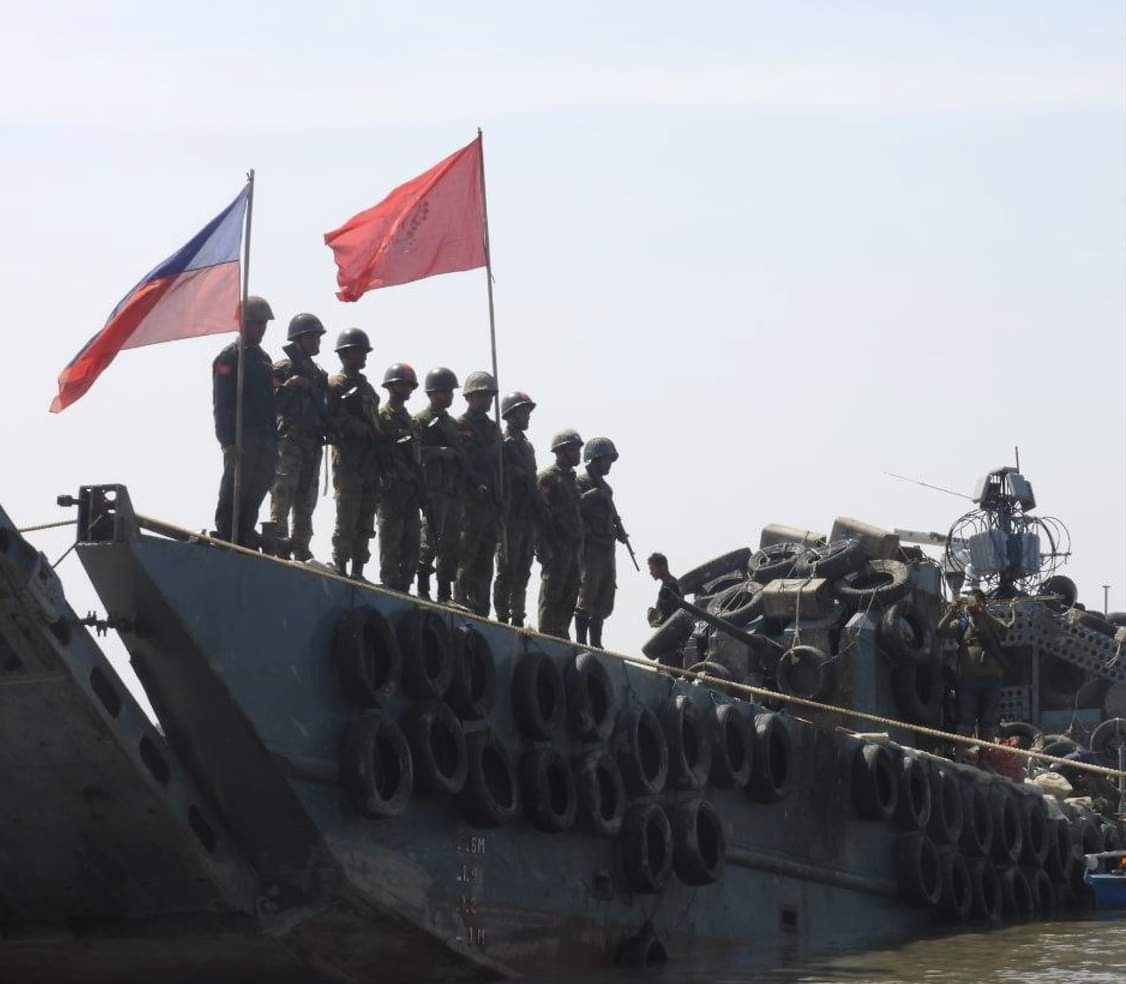
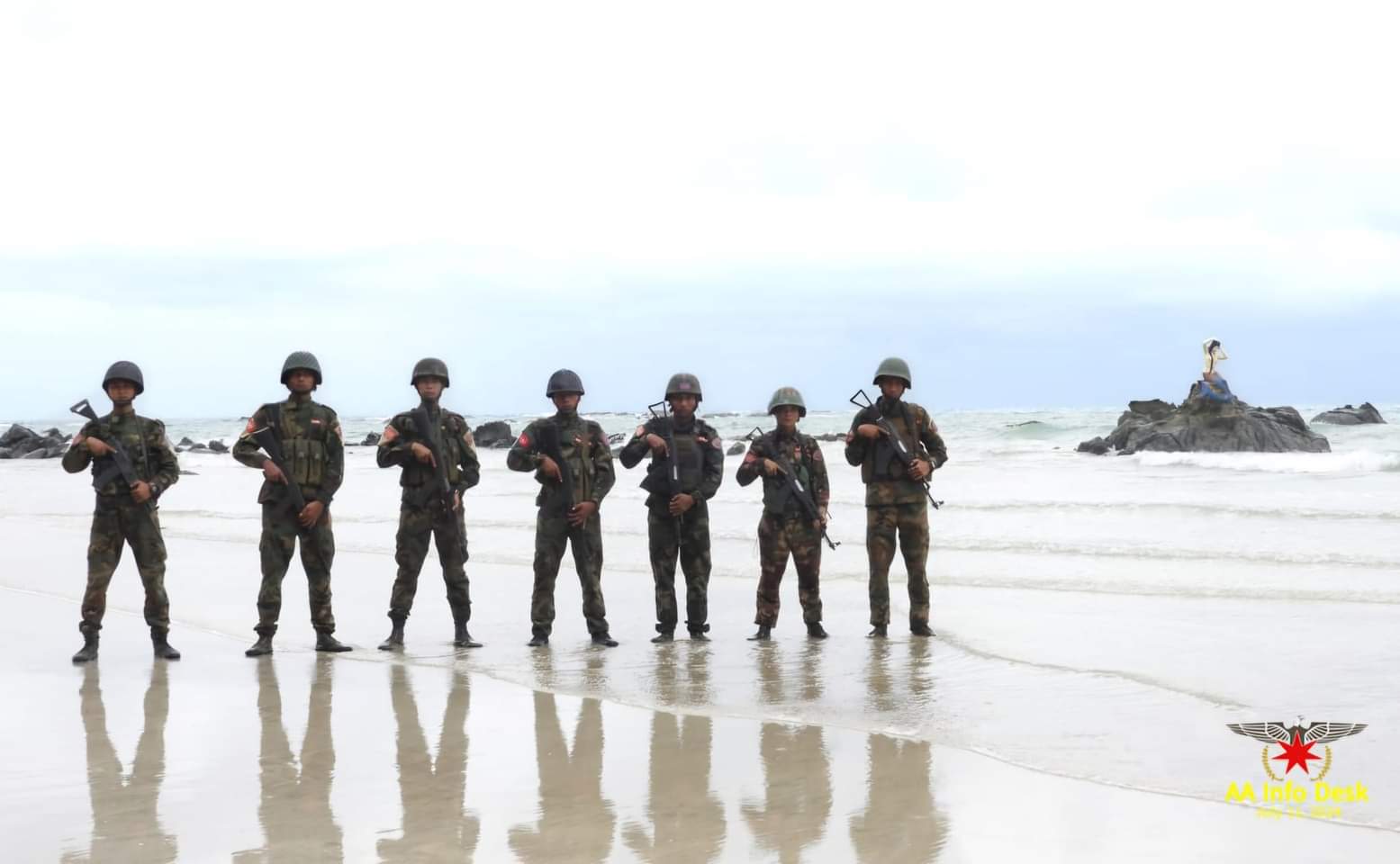
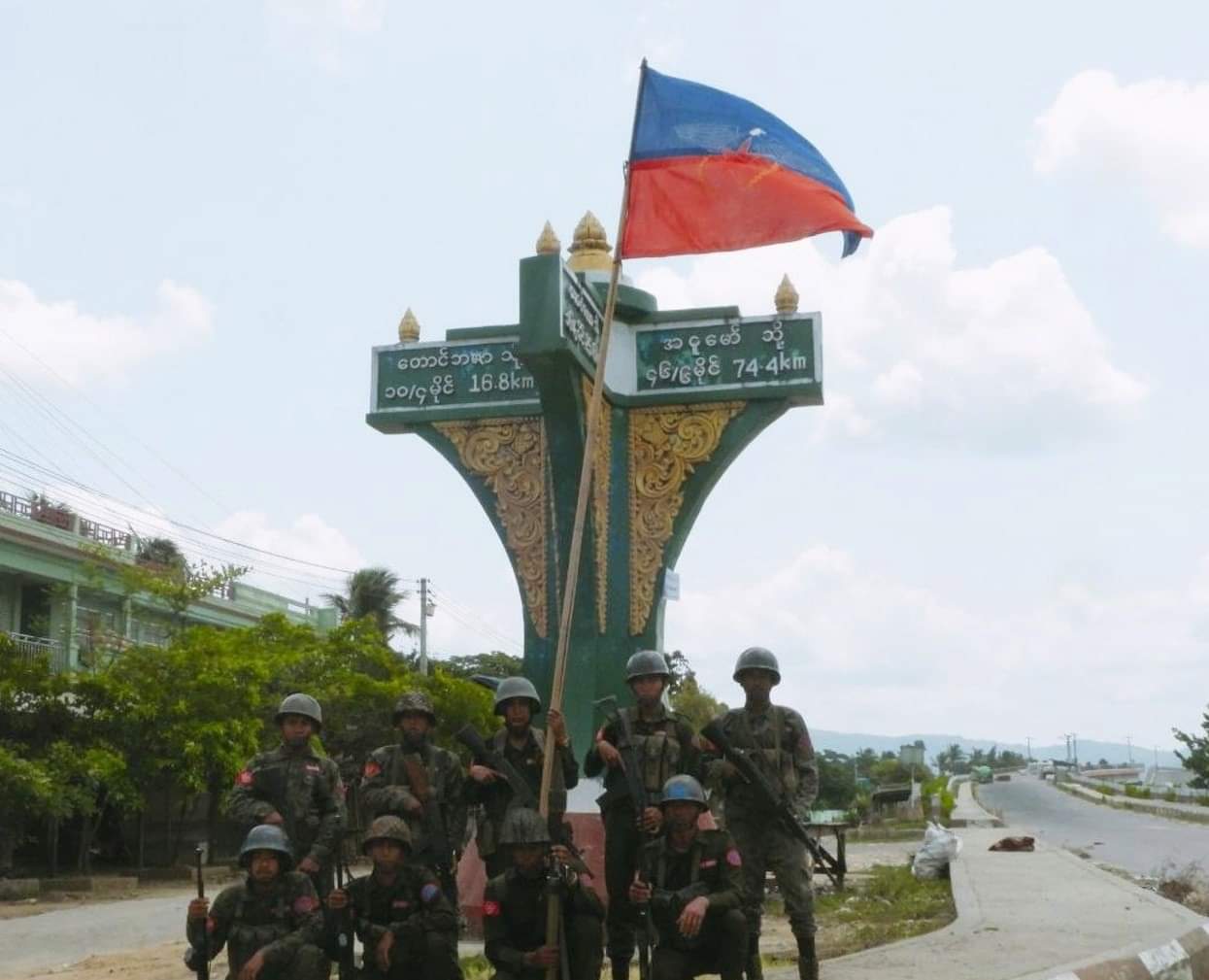
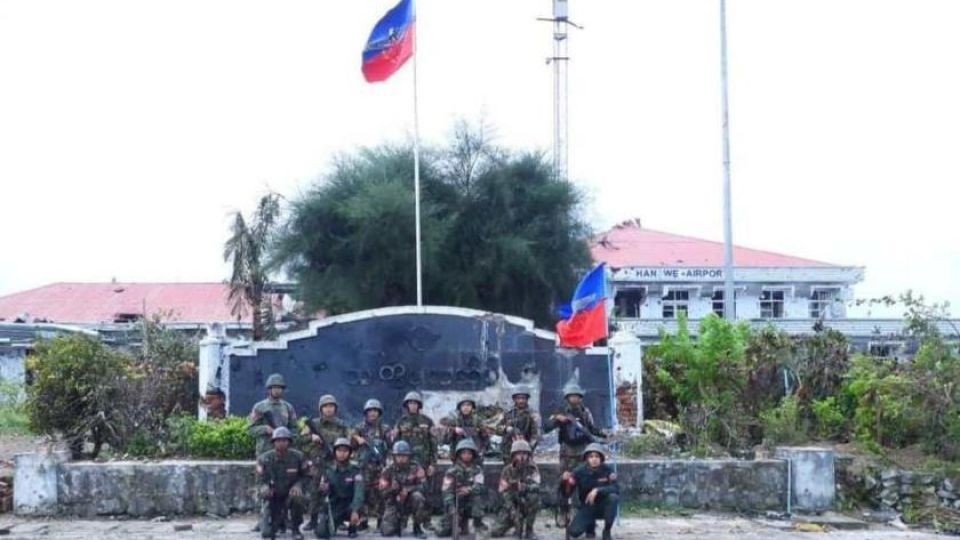
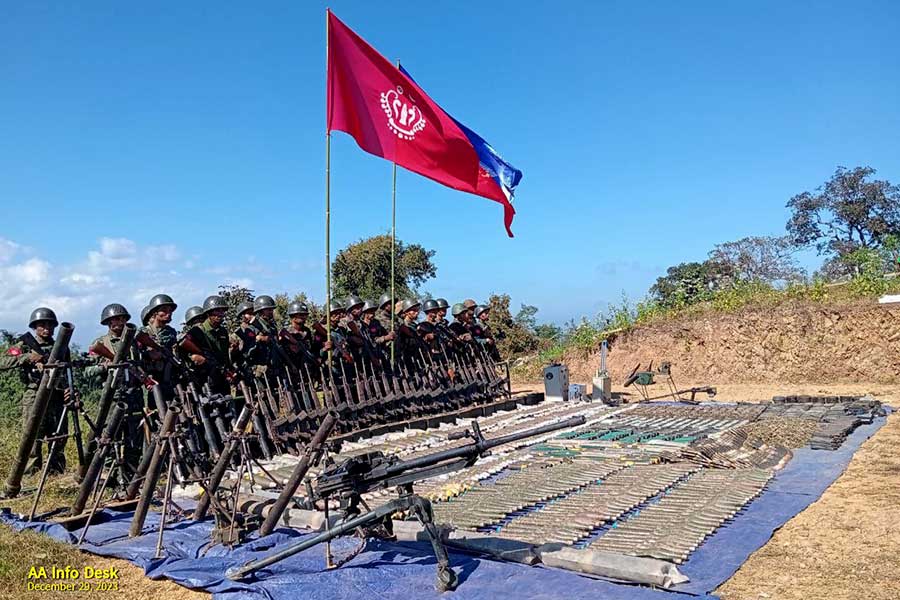
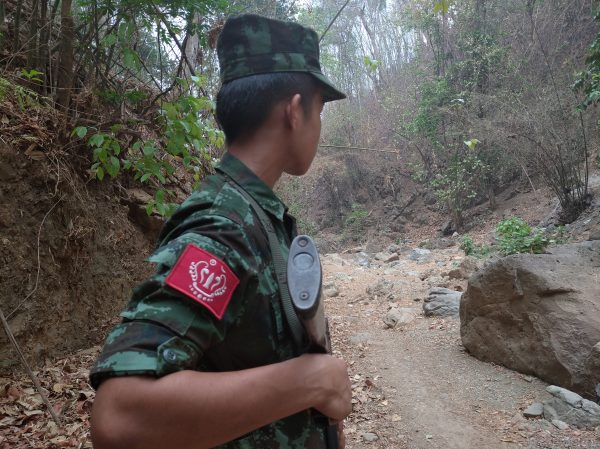
– **Testing and Validation**: Implementing rigorous testing protocols to ensure that AI systems behave as intended in various scenarios and environments.
### 2. **Transparency and Explainability**
– **Interpretability**: Developing models that provide insights into their decision-making processes, enabling users to understand how conclusions are reached.
– **Documentation**: Creating comprehensive documentation for AI models that detail their training data, algorithms, limitations, and intended use cases.
### 3. **Ethical Considerations**
– **Fairness**: Ensuring that AI systems are trained on diverse datasets to minimize bias and promote equitable outcomes across different demographic groups.
– **Accountability**: Establishing clear accountability frameworks for AI deployment, detailing the responsibilities of developers and organizations.
### 4. **Human-Centric Design**
– **User Involvement**: Engaging end-users in the design process to ensure that AI tools address real needs and concerns.
– **User-Friendly Interfaces**: Creating intuitive interfaces that help users understand AI capabilities and limitations, fostering informed decision-making.
### 5. **Regulations and Standards**
– **Compliance with Regulations**: Adhering to existing legal frameworks and guidelines regarding AI use, privacy, and data protection.
– **Establishing Standards**: Collaborating with industry and governmental organizations to create standards for trustworthy AI development and deployment.
### 6. **Data Integrity and Privacy**
– **Responsible Data Use**: Using high-quality, ethically sourced datasets that respect privacy and consent.
– **Data Protection Technologies**: Implementing techniques such as differential privacy and federated learning to protect individual data while still allowing for effective model training.
### 7. **Continuous Monitoring and Feedback**
– **Performance Monitoring**: Continuously monitoring AI systems post-deployment to ensure they continue to operate safely and effectively.
– **Feedback Loops**: Establishing mechanisms for users and stakeholders to provide feedback, allowing for iterative improvements in AI systems.
### 8. **Collaboration and Research Partnerships**
– **Interdisciplinary Collaboration**: Partnering with researchers from various fields (e.g., ethics, social science, law) to address the multifaceted challenges of AI.
– **Industry Cooperation**: Sharing best practices, resources, and findings within the AI community to promote widespread adoption of trustworthy technologies.
### 9. **Education and Awareness**
– **Public Engagement**: Raising awareness about AI technologies and their implications through education and outreach programs.
– **Training for Practitioners**: Providing training for developers and practitioners on ethical AI development and best practices for trustworthiness.
By focusing on these areas, researchers, developers, and organizations can contribute to the advancement of trustworthy AI technologies that benefit society while minimizing risks and negative consequences.
Leave a Reply