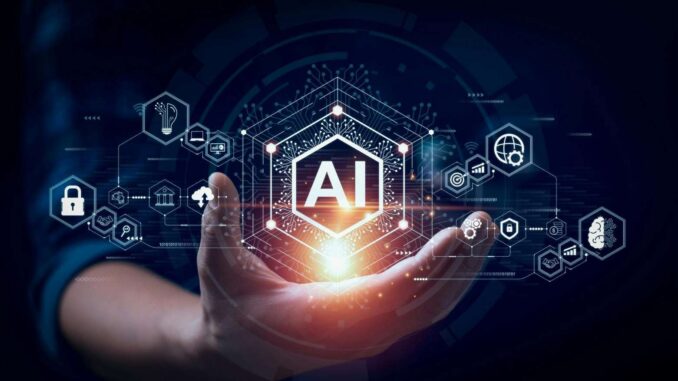
The development of AI has unfolded through several key phases, each marked by significant technological breakthroughs, research advancements, and shifts in societal perceptions. Here’s an overview of the major milestones in AI development:
### 1. **Early Foundations (1950s-1960s)** – **Turing Test:** In 1950, Alan Turing proposed the Turing Test as a criterion of intelligence, raising questions about machine capability and consciousness.
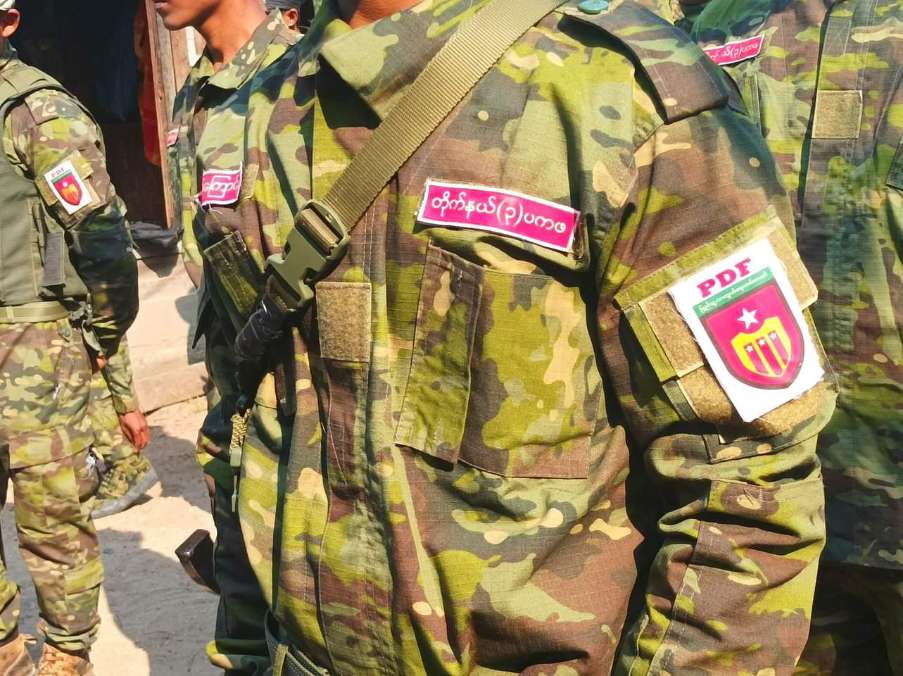
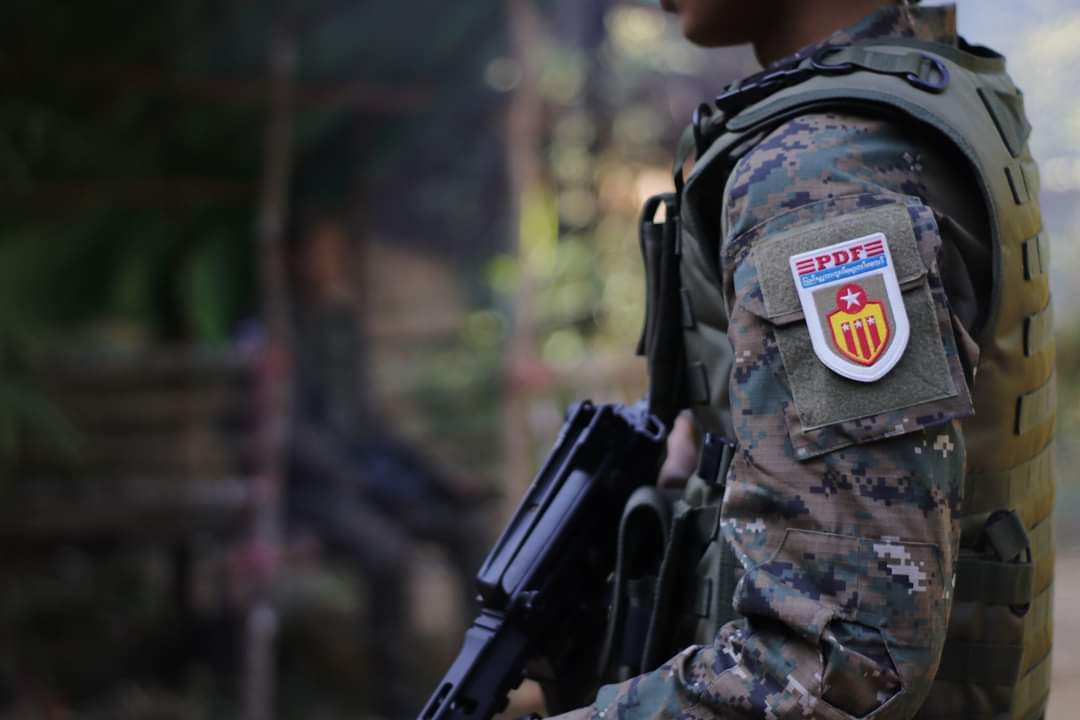
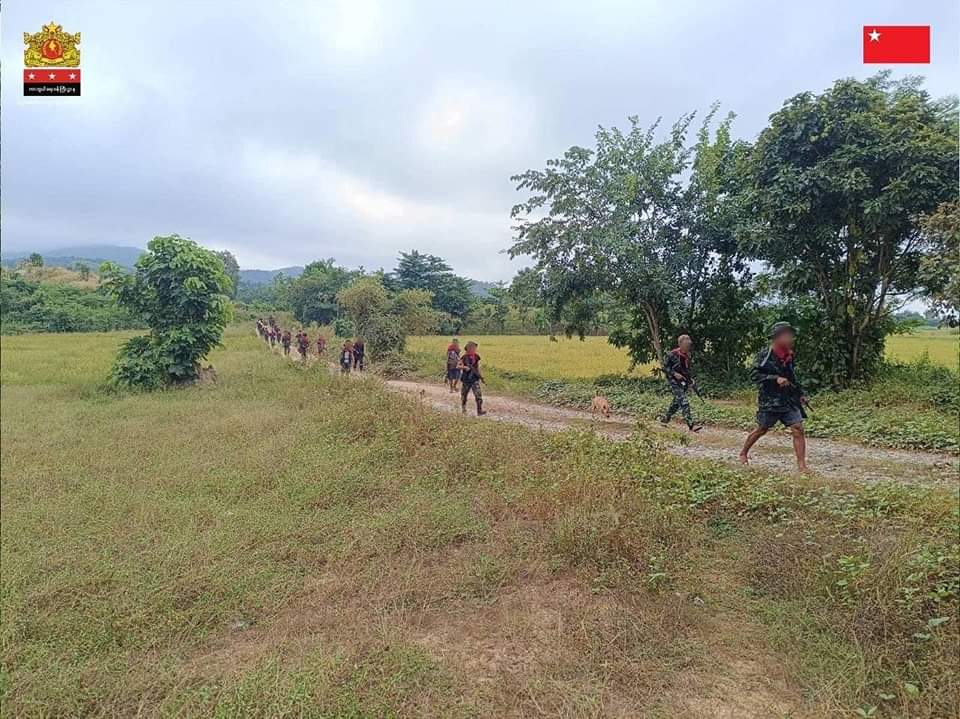
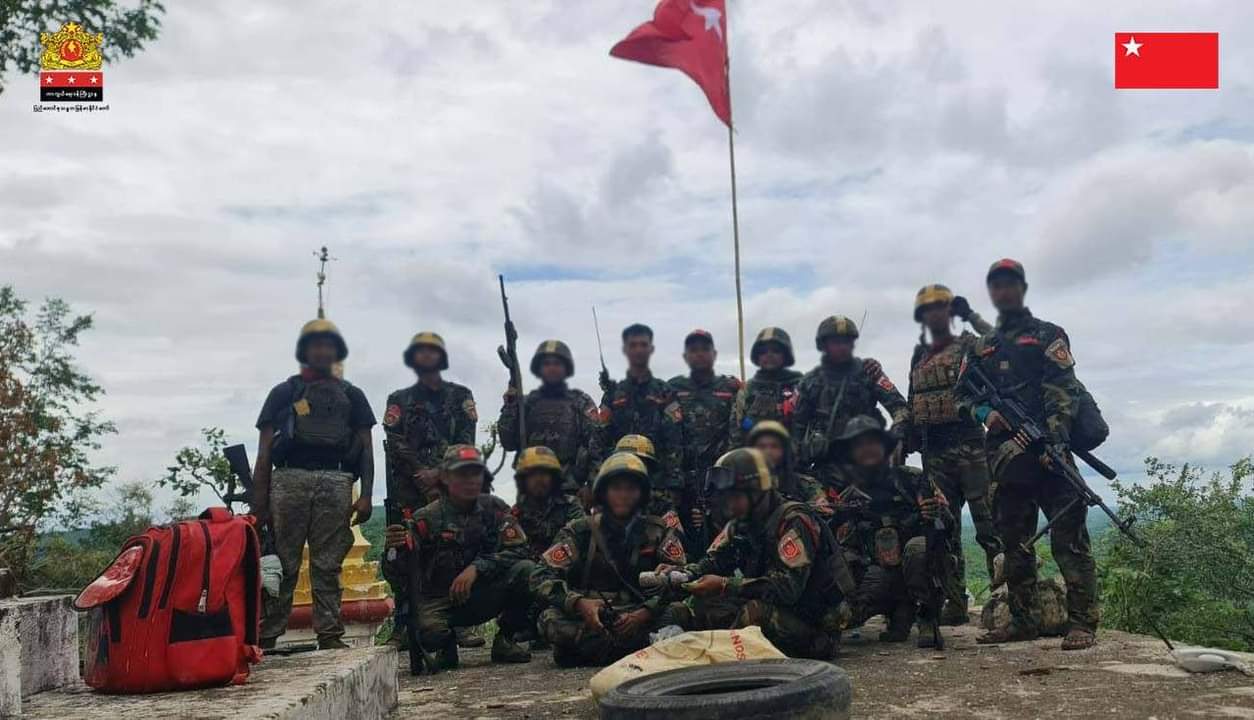
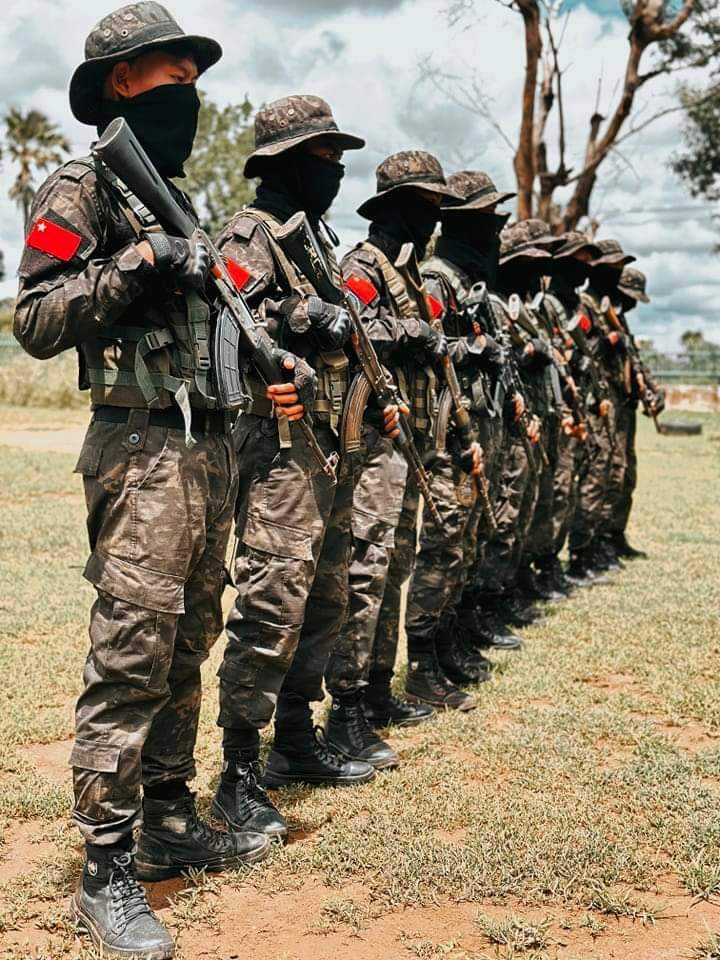
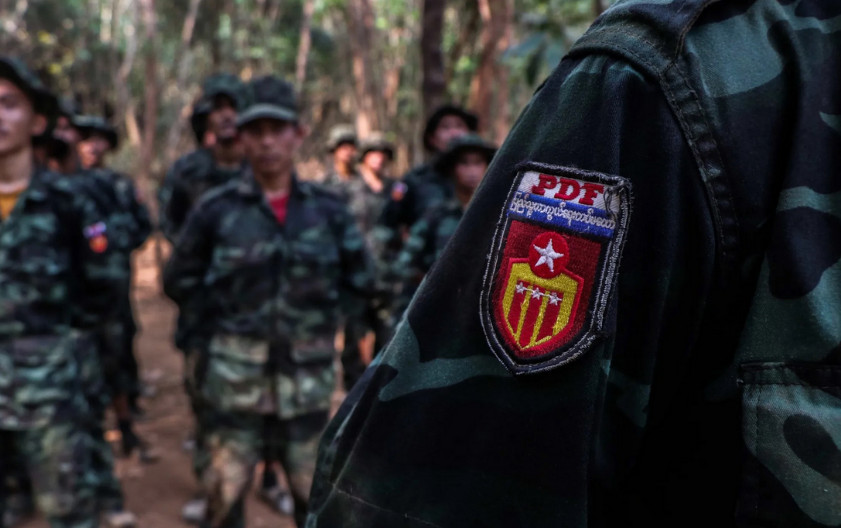
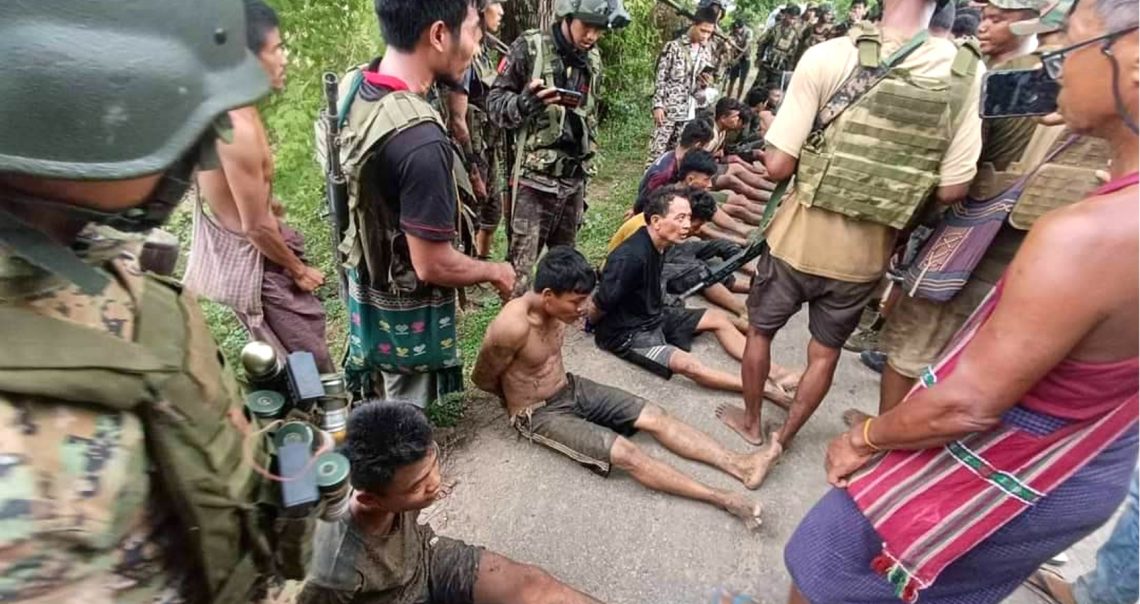
– **First AI Programs:** Early experiments included programs like the Logic Theorist (1955) and General Problem Solver (1957), which aimed to solve mathematical problems.
### 2. **The Golden Years (1960s-1970s)**
– **Natural Language Processing:** The development of programs like ELIZA, which simulated conversation, marked significant strides in NLP.
– **Expert Systems:** In the 1970s, expert systems like MYCIN were designed to mimic human decision-making in specialized areas, such as diagnosing diseases.
### 3. **AI Winter (1970s-1990s)**
– **Funding Cuts and Disillusionment:** Due to high expectations and the limitations of existing technologies, interest and funding for AI research dropped, leading to what is known as the “AI Winter.”
### 4. **Resurgence and Machine Learning (1980s-1990s)**
– **Reinvention of Neural Networks:** Researchers revitalized interest in neural networks, leading to the development of backpropagation algorithms.
– **Increased Computing Power:** The rise of personal computing and improved hardware facilitated computationally intensive AI research.
### 5. **Data-Driven Approaches (2000s-2010s)**
– **Big Data:** The advent of big data allowed machine learning models to be trained on vast datasets, leading to remarkable advancements in performance and accuracy.
– **Deep Learning:** Breakthroughs in deep learning architectures (e.g., convolutional neural networks) enabled significant progress in image and speech recognition, with models like AlexNet (2012) winning major competitions.
### 6. **AI for Everyone (2010s-Present)**
– **Mainstream Adoption:** AI technologies began to be integrated into consumer products (e.g., virtual assistants like Siri and Alexa, recommendation algorithms on Netflix and Amazon).
– **OpenAI and Collaboration:** Organizations like DeepAI, alongside advancements in research collaboration, democratized access to AI tools and frameworks, fostering innovation across various sectors.
### 7. **Current Developments and Future Directions**
– **Generative AI:** The emergence of generative models (like GPT-3 and DALL-E) showcases AI’s ability to create human-like text and images, expanding creative and business applications.
– **Ethics and Regulation:** Growing concerns about bias, privacy, and the implications of AI on jobs and society have led to calls for ethical guidelines and regulatory frameworks to ensure responsible AI deployment.
– **Interdisciplinary Applications:** AI is increasingly being applied in diverse fields—from drug discovery and climate modeling to autonomous vehicles and personalized medicine—highlighting its transformative potential.
### Challenges and Considerations
As AI continues to evolve, several challenges remain:
– **Bias and Fairness:** Ensuring AI systems are fair and do not perpetuate existing biases.
– **Transparency and Explainability:** Developing systems that can explain their decision-making processes to users.
– **Job Displacement:** Addressing the potential for AI to disrupt labor markets and the economy.
– **Security and Privacy:** Safeguarding user data and protecting AI systems from malicious attacks.
The development of AI is an ongoing journey, with continuous advancements shaping the future of technology and society. As we move forward, collaboration among technologists, policymakers, and the public is crucial to navigate the complexities and harness the benefits of AI responsibly.
Leave a Reply