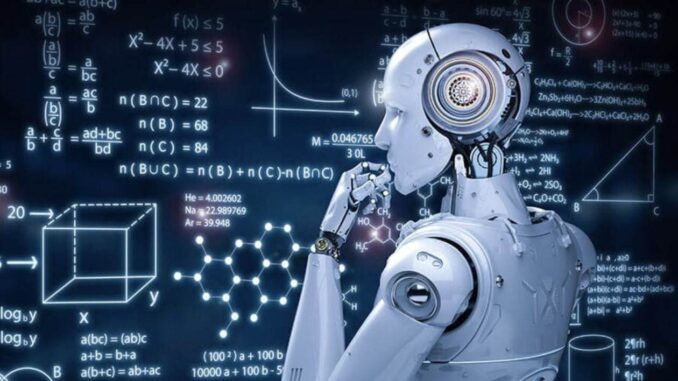
AI technologies are indeed evolving rapidly, leading to significant advancements in capabilities, applications, and integration into various sectors.
Here’s a detailed look at how AI technologies are evolving, including trends, emerging technologies, and their implications for businesses and society:
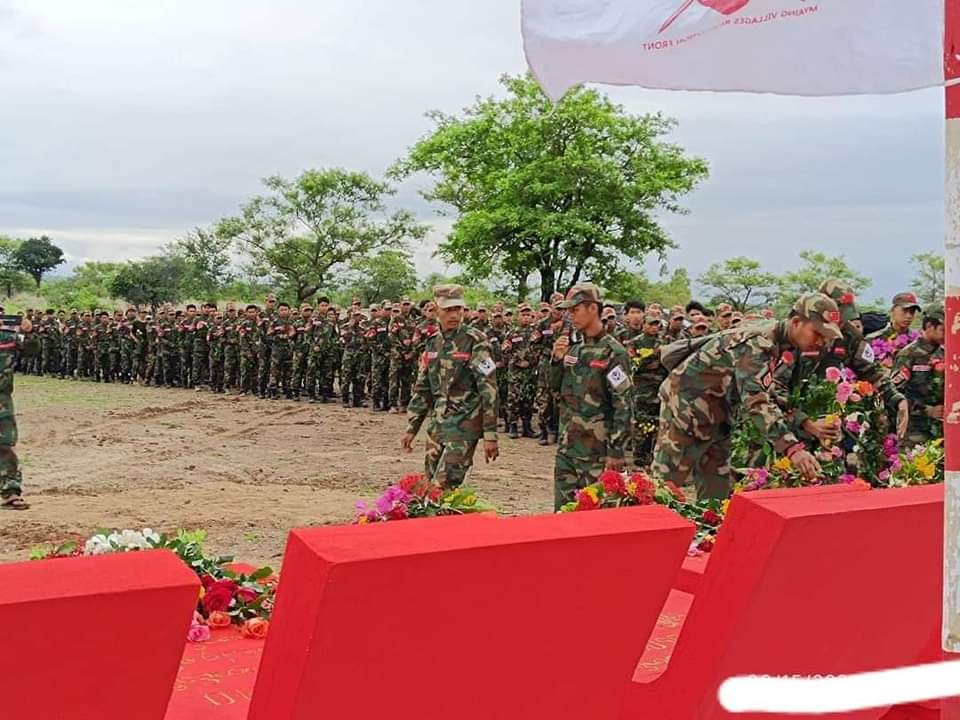
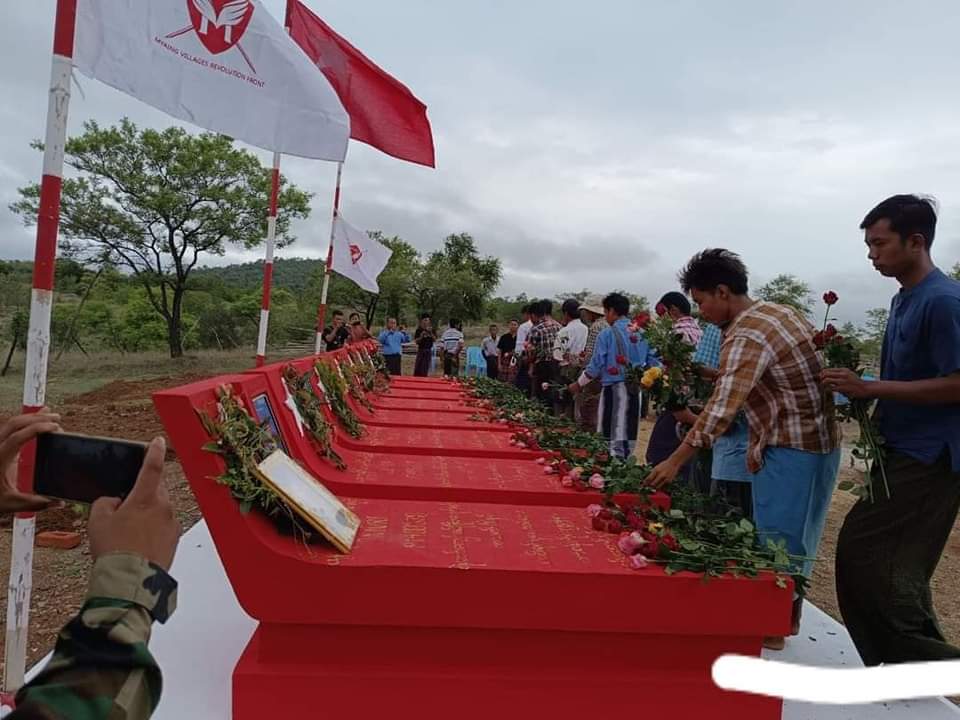
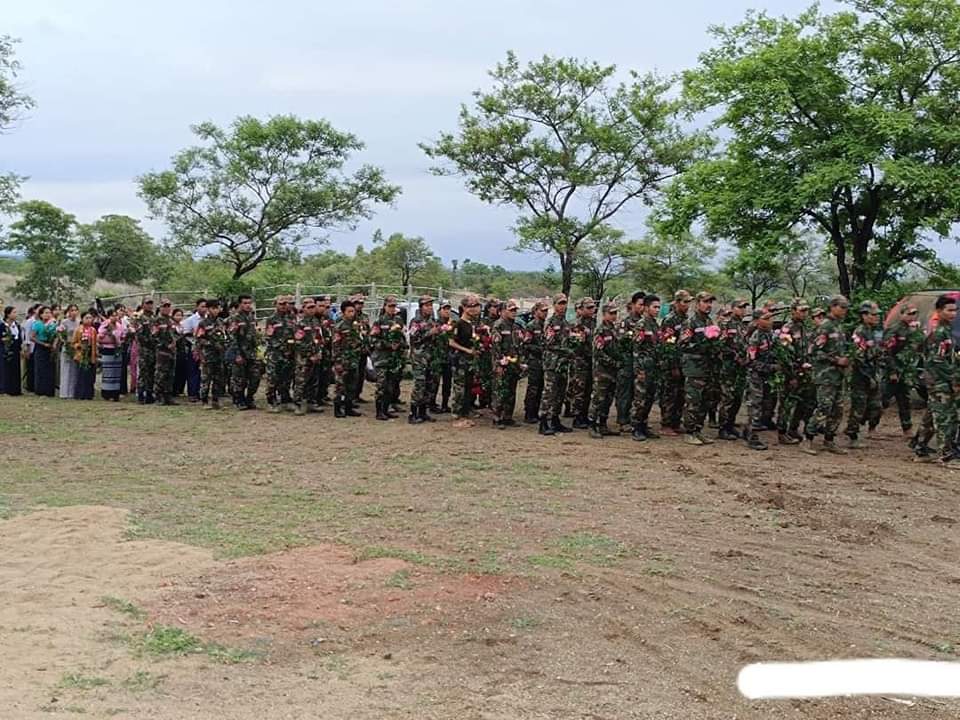
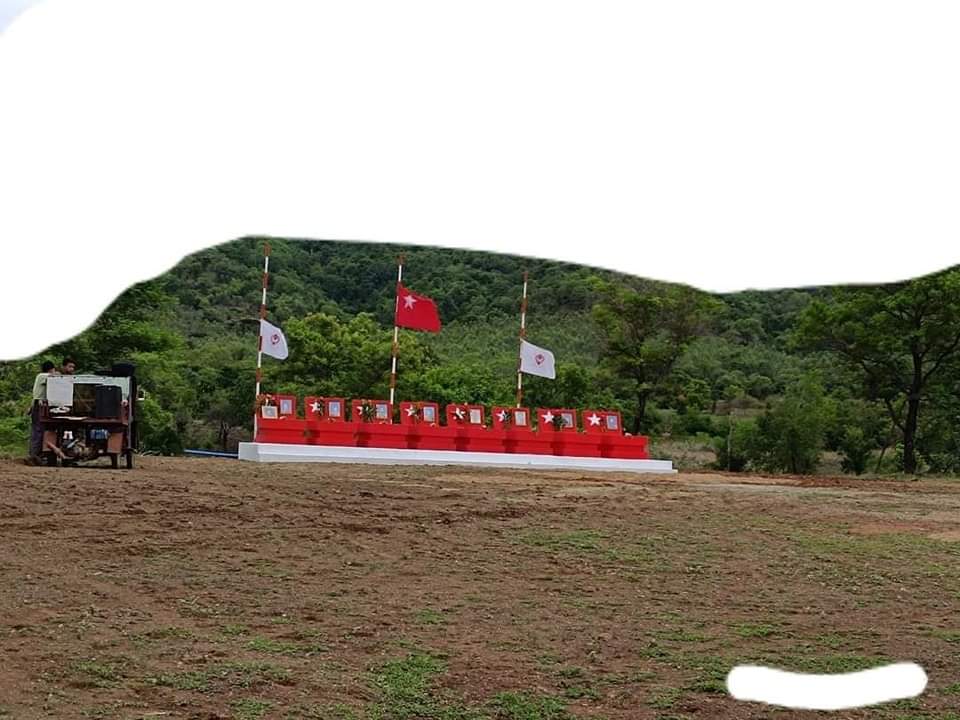
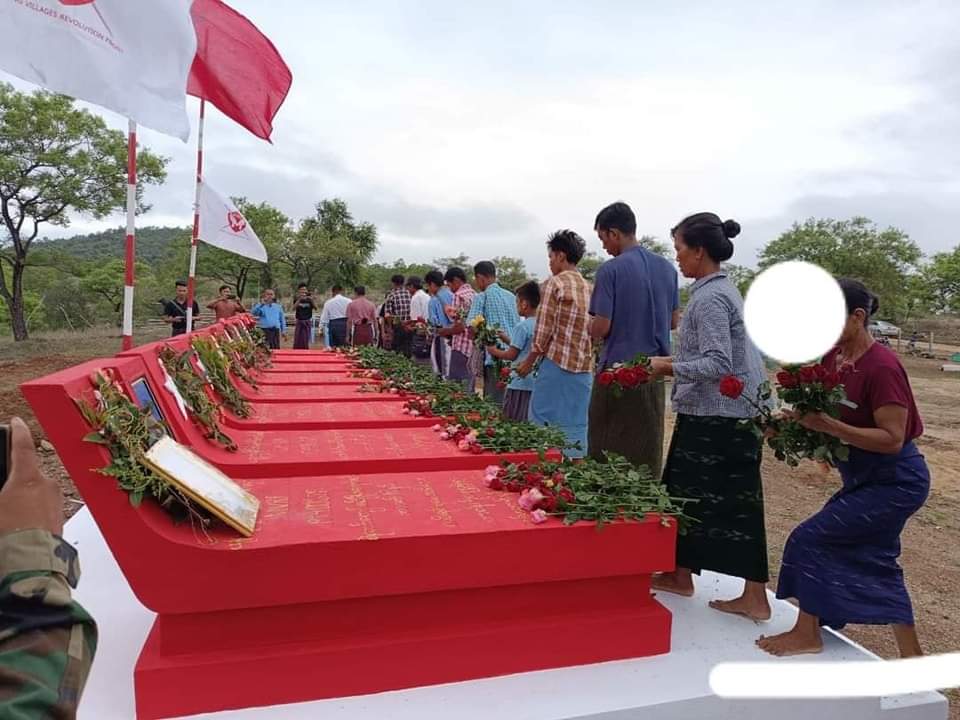
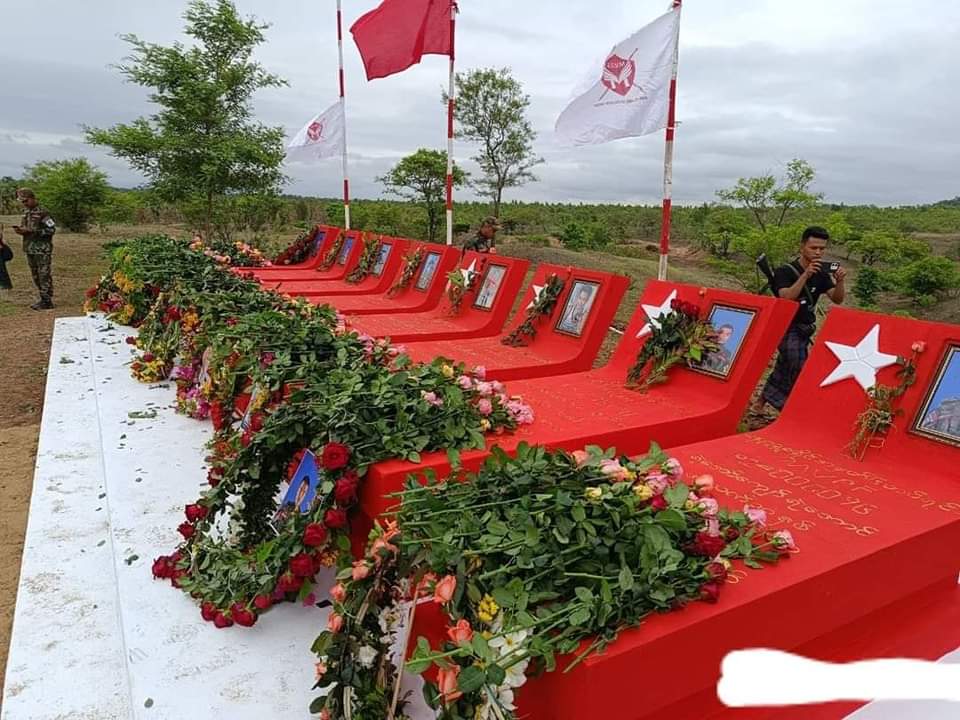
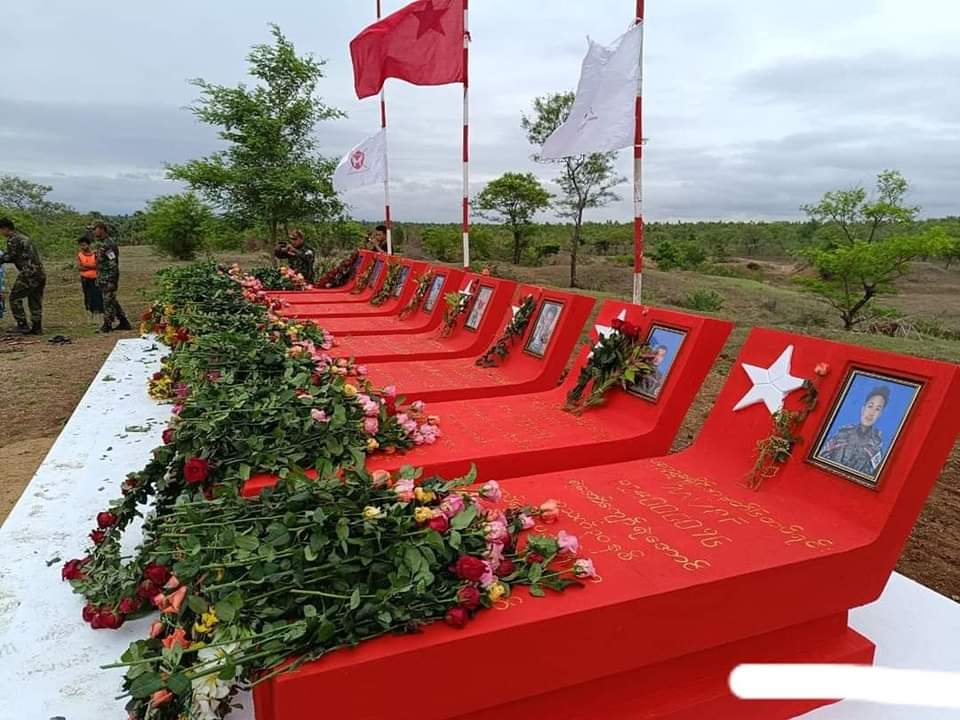
### 1. **Advancements in Machine Learning (ML)**
– **Deep Learning**: Advances in neural networks, particularly deep learning, are enabling AI systems to handle complex tasks such as image and speech recognition with improved accuracy. Innovations like transformer architectures drive breakthroughs in natural language processing (NLP) applications.
– **Reinforcement Learning**: This approach allows AI systems to learn by interacting with their environment and receiving feedback. Applications include gaming, robotics, and optimizing complex systems.
– **AutoML (Automated Machine Learning)**: Tools that automate the model selection and tuning processes are making it easier for organizations to implement machine learning without requiring deep expertise.
### 2. **Natural Language Processing (NLP)**
– **Conversational AI**: The development of sophisticated natural language understanding (NLU) models has improved chatbots and virtual assistants, enabling them to engage in nuanced conversations and handle complex queries.
– **Sentiment Analysis**: Businesses are increasingly using NLP to gauge customer sentiment from reviews and social media, helping to inform marketing and product decisions.
– **Machine Translation**: AI-driven translators are becoming more accurate and context-aware, facilitating global communication.
### 3. **Computer Vision**
– **Image and Video Analysis**: AI technologies are being harnessed for applications in surveillance, retail (like cashier-less stores), healthcare (medical imaging), and autonomous vehicles.
– **Augmented Reality (AR) and Virtual Reality (VR)**: Computer vision advancements are enhancing AR/VR experiences, making them more immersive and interactive.
### 4. **Ethics and Explainability**
– **AI Ethics Frameworks**: As AI infiltrates high-stakes areas such as healthcare, finance, and law enforcement, there is an increasing focus on ethical AI. Organizations are developing frameworks to ensure fairness, accountability, and transparency in their AI systems.
– **Explainable AI (XAI)**: Efforts are underway to develop AI systems that can explain their decision-making processes. This is essential for trust and compliance, particularly in regulated industries.
### 5. **Edge AI**
– **Computing at the Edge**: The rise of IoT (Internet of Things) devices has fueled the adoption of edge AI, where data processing occurs locally on devices rather than relying on centralized cloud-based systems. This improves response times and reduces bandwidth usage.
– **Real-Time Decision Making**: Edge AI enables applications that require immediate action, such as autonomous vehicles, smart grids, and real-time video analytics.
### 6. **Generative AI**
– **Content Creation**: Advances in models like OpenAI’s GPT-3 and DALL-E are allowing for the generation of human-like text, images, music, and more, fostering creativity in fields such as marketing, design, and entertainment.
– **Synthetic Data Generation**: Generative models are being used to create synthetic data for training other models, helping to overcome data limitations and privacy concerns.
### 7. **AI in Robotics**
– **Collaborative Robots (Cobots)**: AI-powered robots are increasingly being designed to work alongside humans in manufacturing, logistics, and healthcare settings, enhancing productivity and safety.
– **Autonomous Vehicles**: Significant advancements in AI algorithms are facilitating the development of fully autonomous vehicles, with improved navigation, obstacle detection, and decision-making.
### 8. **Integration and Interoperability**
– **AI as a Service (AIaaS)**: Cloud providers are offering AI capabilities as services, allowing businesses to leverage AI tools without significant upfront investments. This makes advanced AI technologies accessible to smaller organizations.
– **Cross-Platform Integration**: Enhanced integration between AI platforms and traditional business systems (like CRM and ERP) is enabling organizations to leverage AI-driven insights seamlessly within their operations.
### 9. **Future Directions**
– **Human-AI Collaboration**: Future AI systems will focus on augmenting human capabilities, emphasizing collaboration rather than replacement. This leads to a new paradigm of work where human judgment is complemented by AI-generated insights.
– **Personalization and User Experience**: As AI continues to evolve, it will enable hyper-personalized experiences across customer interactions, driving engagement and loyalty.
– **Sustainability**: There is a growing movement to use AI to address environmental challenges, optimizing resource use, reducing waste, and improving efficiency in industries such as agriculture and energy.
### Conclusion
The evolution of AI technologies is reshaping industries, enhancing productivity, and driving innovation. As these technologies continue to mature, their integration into business processes and everyday life will become increasingly impactful. However, organizations must prioritize ethical practices, transparency, and collaboration to harness the full potential of AI while addressing associated challenges and societal implications.
Leave a Reply