
The integration of artificial intelligence (AI) refers to the incorporation of AI technologies and methodologies into various systems, processes, and applications to enhance functionality, improve efficiency, and enable smarter decision-making.
This integration can occur across a wide range of industries and domains, often resulting in significant advancements in productivity, innovation, and user experience.
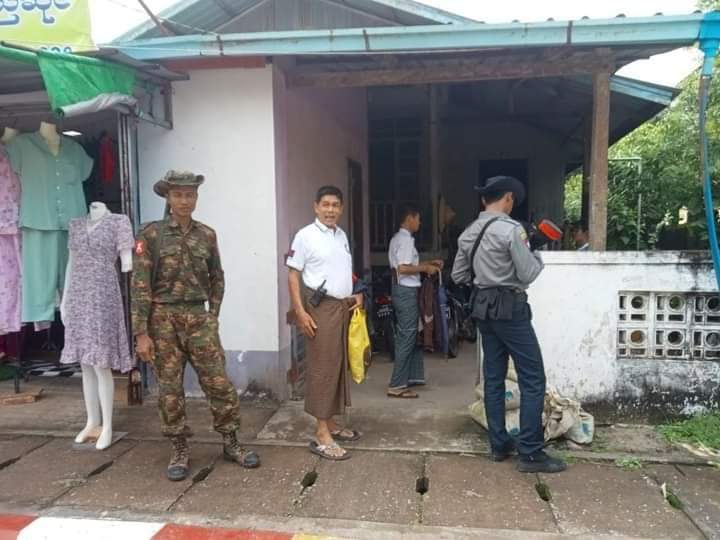
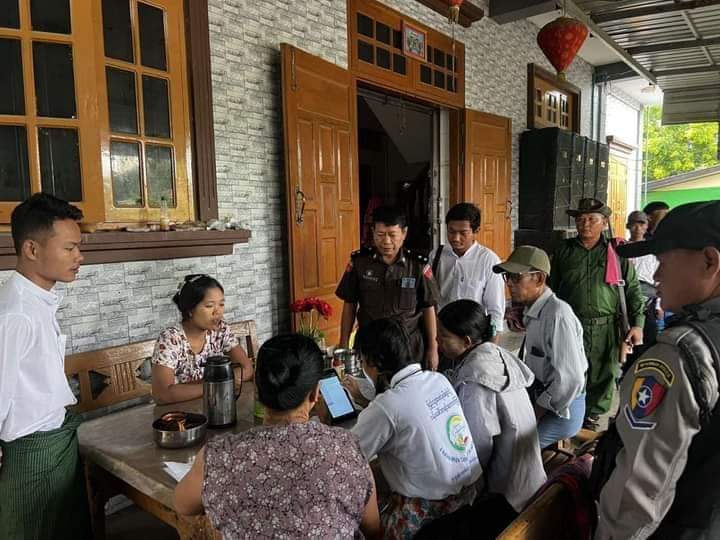
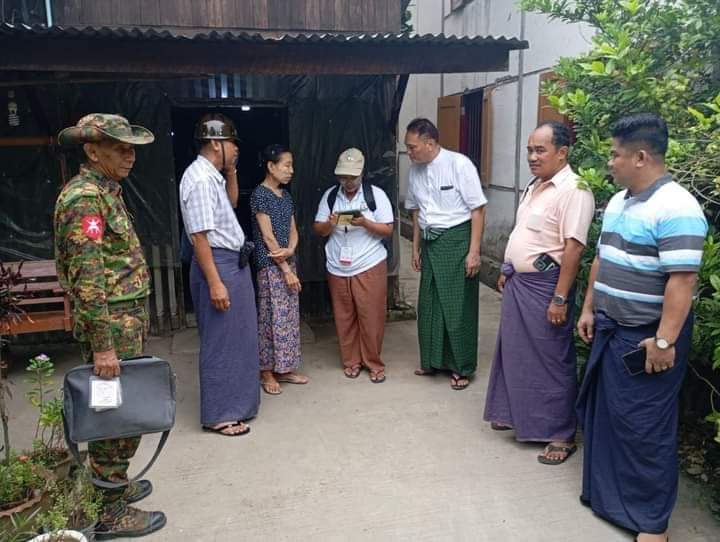
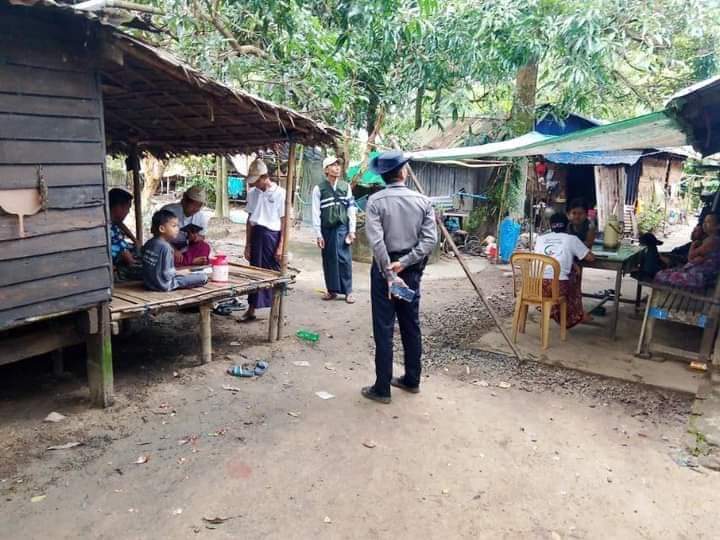
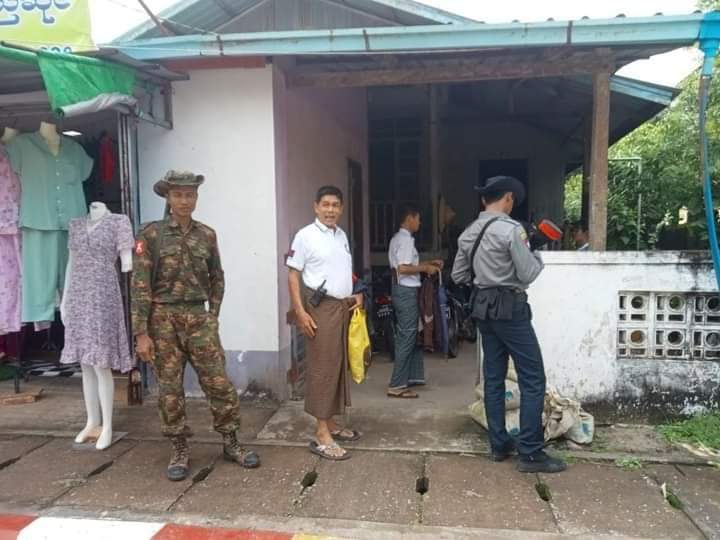
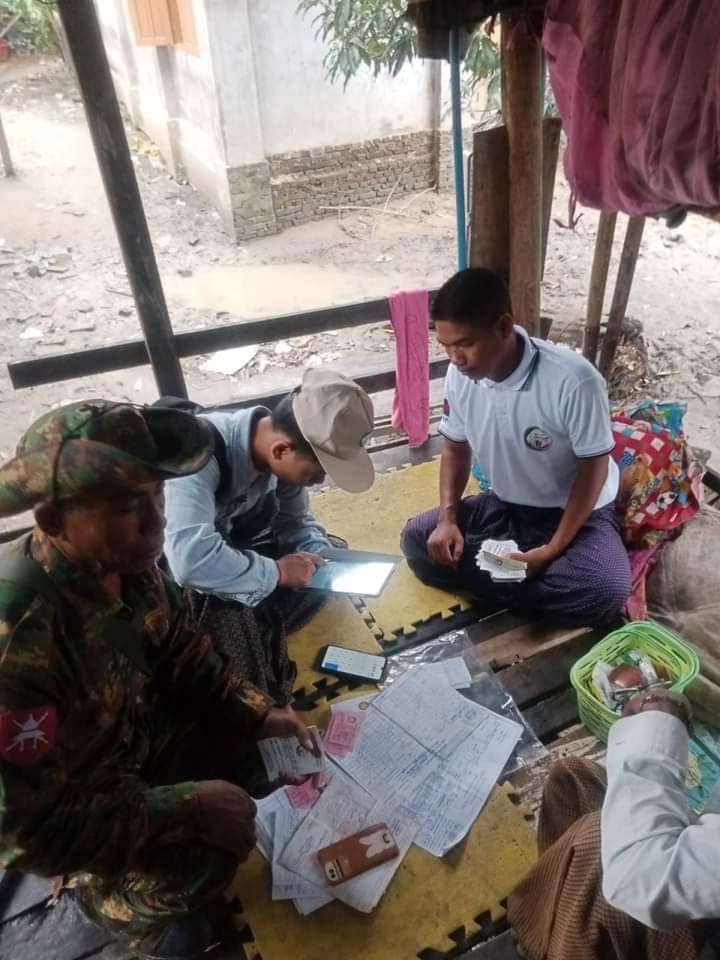
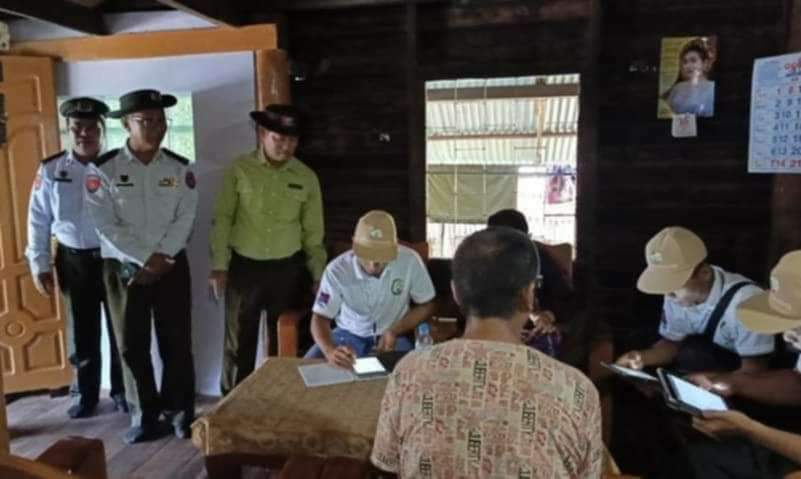
Here are some key aspects, benefits, challenges, and trends associated with the integration of AI:
### Key Aspects of AI Integration
1. **Data Utilization**:
– AI requires vast amounts of data for training machine learning models. Organizations must have robust data collection, storage, and management systems in place to leverage AI effectively.
– Data can come from various sources, including sensors, IoT devices, customer interactions, and enterprise systems.
2. **Machine Learning and Deep Learning**:
– Machine learning (ML) and deep learning (DL) are essential components of AI integration. These techniques allow systems to learn from data, adapt to new inputs, and make predictions or recommendations.
– Training models require specialized knowledge in algorithms and data science.
3. **API and Framework Integration**:
– Many organizations utilize existing AI frameworks (like TensorFlow, PyTorch, or scikit-learn) and APIs (like DeepAI’s GPT or Google Cloud AI) to streamline the integration of AI capabilities into their services or products.
4. **Infrastructure**:
– Effective integration requires a robust technological infrastructure, including cloud computing resources, edge devices, or specialized hardware (e.g., GPUs, TPUs).
– Scalability, security, and performance are essential considerations.
5. **Human-Machine Collaboration**:
– AI integration often involves redefining workflows, featuring collaboration between humans and machines. Human oversight is crucial in many applications, especially those involving ethical considerations or complex decision-making.
### Benefits of AI Integration
1. **Increased Efficiency**:
– AI can automate repetitive tasks, streamline processes, and optimize resource allocation, leading to significant efficiency gains.
2. **Enhanced Decision-Making**:
– AI can analyze vast datasets to provide insights, predictions, and recommendations, aiding better strategic and operational decision-making.
3. **Personalization**:
– AI enables personalized experiences for users, such as tailored content recommendations in streaming services or customized marketing strategies in e-commerce.
4. **Cost Savings**:
– By automating tasks and optimizing processes, organizations can reduce operational costs and improve profit margins.
5. **Innovation**:
– The integration of AI can lead to the development of innovative products and services that improve customer experiences and enable new business models.
### Challenges of AI Integration
1. **Data Quality and Management**:
– The success of AI largely depends on the quality and relevance of the data used. Organizations must invest in data governance strategies.
2. **Skill Gaps**:
– There is a significant demand for data scientists, machine learning engineers, and AI specialists, leading to a skills gap in many sectors.
3. **Integration Costs**:
– Implementing AI can involve substantial initial investment in technology, talent, and infrastructure.
4. **Ethical and Regulatory Concerns**:
– Issues such as bias in AI models, data privacy, and the implications of automated decision-making must be addressed proactively. Adhering to regulations and ethical principles is crucial.
5. **Change Management**:
– Organizations must manage the cultural and operational changes that come with AI integration, ensuring that teams are aligned and engaged with new technologies.
### Trends in AI Integration
1. **Edge AI**:
– The movement of AI computation closer to data sources (edge devices) to enable real-time processing and decision-making while reducing latency.
2. **Natural Language Processing (NLP)**:
– Ongoing advancements in NLP are enabling more intuitive human-computer interactions, from chatbots to voice assistants.
3. **AI in Cybersecurity**:
– Increasing use of AI to detect and respond to cybersecurity threats in real-time, leveraging behavioral analytics and anomaly detection.
4. **Robotics and Automation**:
– AI integration in robotics is driving advancements in manufacturing, logistics, and beyond, facilitating more autonomous and intelligent systems.
5. **AI and Sustainability**:
– Growing focus on using AI to tackle environmental challenges, optimize resource usage, and enhance sustainability across industries.
### Conclusion
The integration of artificial intelligence represents a transformative force across sectors, enabling organizations to enhance their operations, innovate their offerings, and drive strategic advantages. While challenges remain—particularly in terms of data management, ethics, and workforce readiness—proactive approaches toward AI integration can lead to significant benefits and help organizations navigate the complexities of the modern digital landscape. As AI continues to evolve, its integration will likely deepen, making it a fundamental component of future business strategies.
Leave a Reply