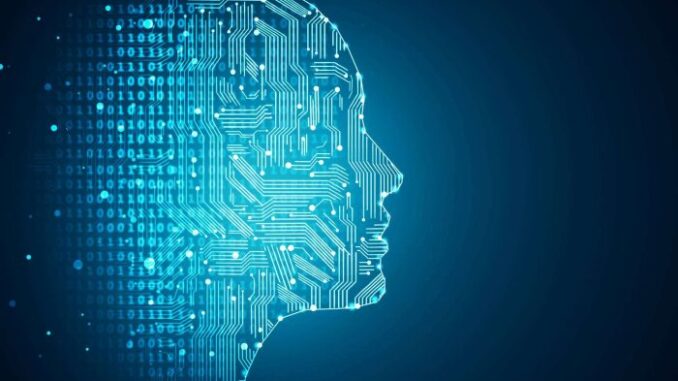
Leveraging domain-specific models in artificial intelligence can significantly enhance the effectiveness and accuracy of systems across various industries.
These models are tailored to understand and process data relevant to a specific domain, making them more adept at handling specialized tasks compared to general-purpose AI models.
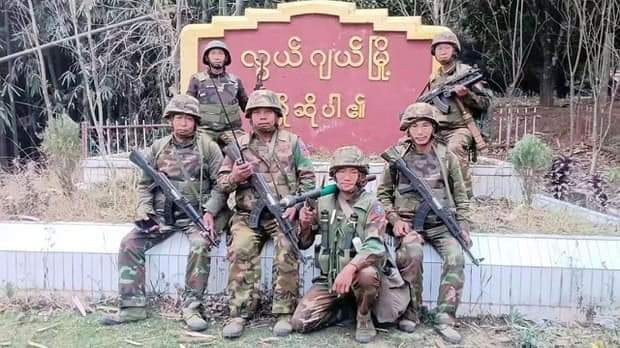
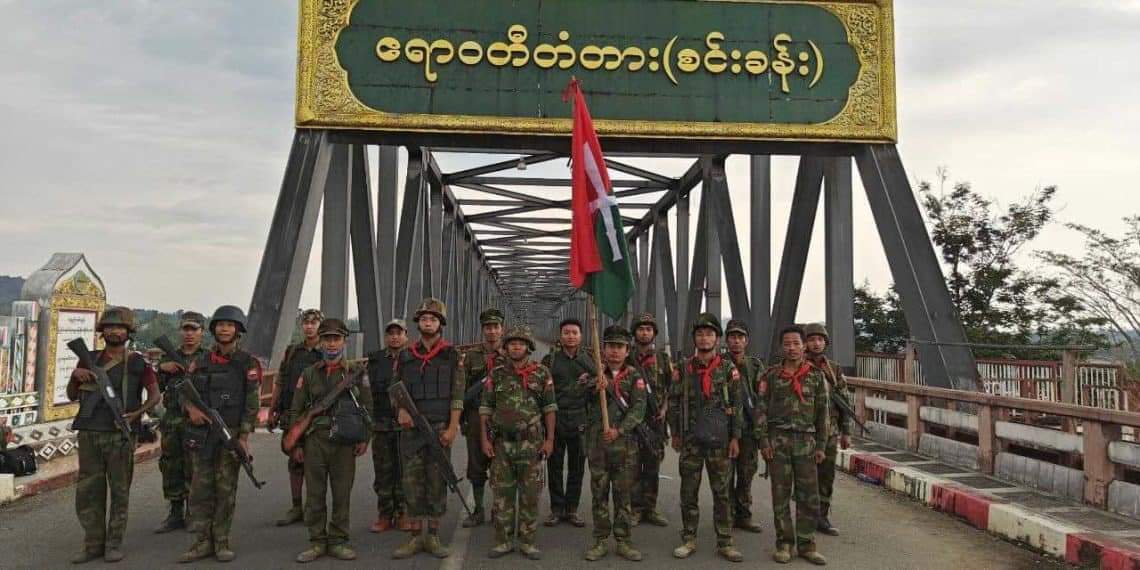


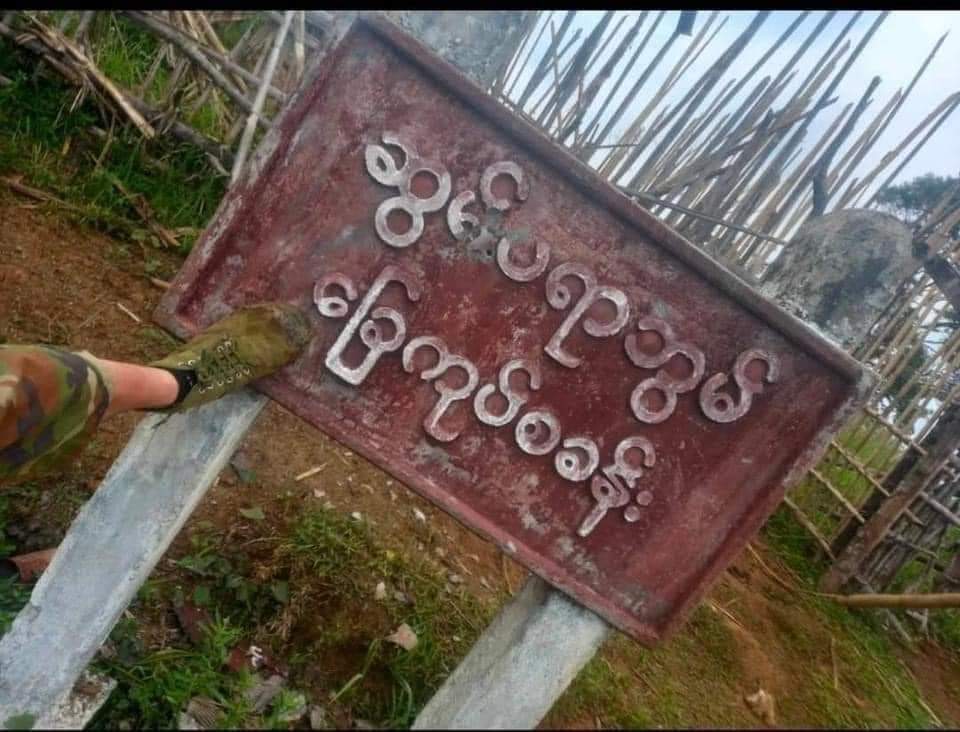

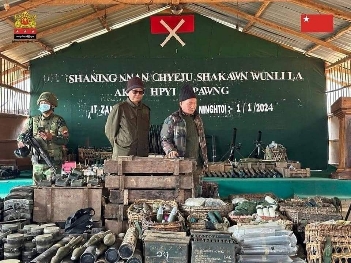
Here are several key aspects and applications of domain-specific models:
### Key Aspects:
1. **Custom Data Training:**
– Domain-specific models are trained on datasets that are relevant to a particular field (e.g., medical records for healthcare models, legal documents for law-related models).
– This training allows the model to learn domain-specific terminology, nuances, and intricacies.
2. **Improved Accuracy:**
– By focusing on a narrow domain, these models can achieve higher accuracy in predictions and insights than general models, which may lack contextual knowledge.
3. **Regulatory Compliance:**
– In regulated industries (like finance or healthcare), domain-specific models can help ensure compliance with industry standards and regulations by incorporating the necessary guidelines into their processing.
4. **Enhanced Interpretability:**
– Tailored models can be designed to provide insights and explanations that are easier for domain experts to understand, fostering trust and usability.
5. **Reduced Data Requirements:**
– While general models may require vast amounts of data to perform adequately, domain-specific models can often function well with less data because they are designed to leverage domain expertise.
### Applications:
1. **Healthcare:**
– **Medical Imaging:** Models trained on medical images (MRIs, CT scans) can assist in diagnosing diseases with higher accuracy.
– **Clinical Decision Support:** AI tools can analyze patient data to support clinical decisions, predicting outcomes based on historical treatment effectiveness.
2. **Finance:**
– **Fraud Detection:** Models trained on transaction data can identify patterns indicative of fraudulent activity.
– **Risk Management:** Domain-specific models can assess loan applications by predicting default risks based on historical lending data.
3. **Legal:**
– **Contract Analysis:** AI models can analyze contracts, highlighting critical clauses and potential risks, and providing insights into compliance issues.
– **Legal Research:** Natural language processing (NLP) models can help lawyers quickly find relevant case law and precedents.
4. **Retail:**
– **Customer Behavior Analysis:** Models can analyze purchasing patterns to optimize inventory and personalize marketing strategies.
– **Recommendation Engines:** Tailored models can suggest products based on intricate understanding of customer preferences.
5. **Manufacturing:**
– **Predictive Maintenance:** AI can be used to predict equipment failures by analyzing sensor data specific to machinery used in the manufacturing process.
– **Quality Control:** Models can detect defects in products by analyzing images or data collected during the production process.
6. **Energy:**
– **Demand Forecasting:** AI can predict energy consumption patterns, allowing for better resource allocation.
– **Grid Management:** Models can optimize the distribution of renewable energy based on weather patterns and energy consumption forecasts.
### Conclusion:
Incorporating domain-specific models into AI applications can lead to more precise, effective, and relevant solutions. By focusing on the unique characteristics of a field, organizations can harness the power of AI to drive innovation, improve efficiencies, and achieve better outcomes. When developing or selecting these models, it’s crucial to work closely with domain experts to ensure that the models align with industry needs and standards.
Leave a Reply