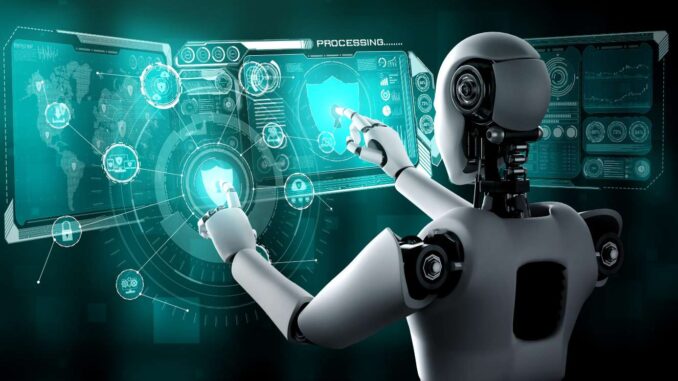
AI has garnered significant attention for its potential environmental impacts, both positive and negative. Here’s an overview of how AI intersects with environmental issues:
### Positive Impacts of AI on the Environment 1. **Energy Efficiency**: AI can optimize energy consumption in various sectors, including manufacturing, transportation, and buildings.
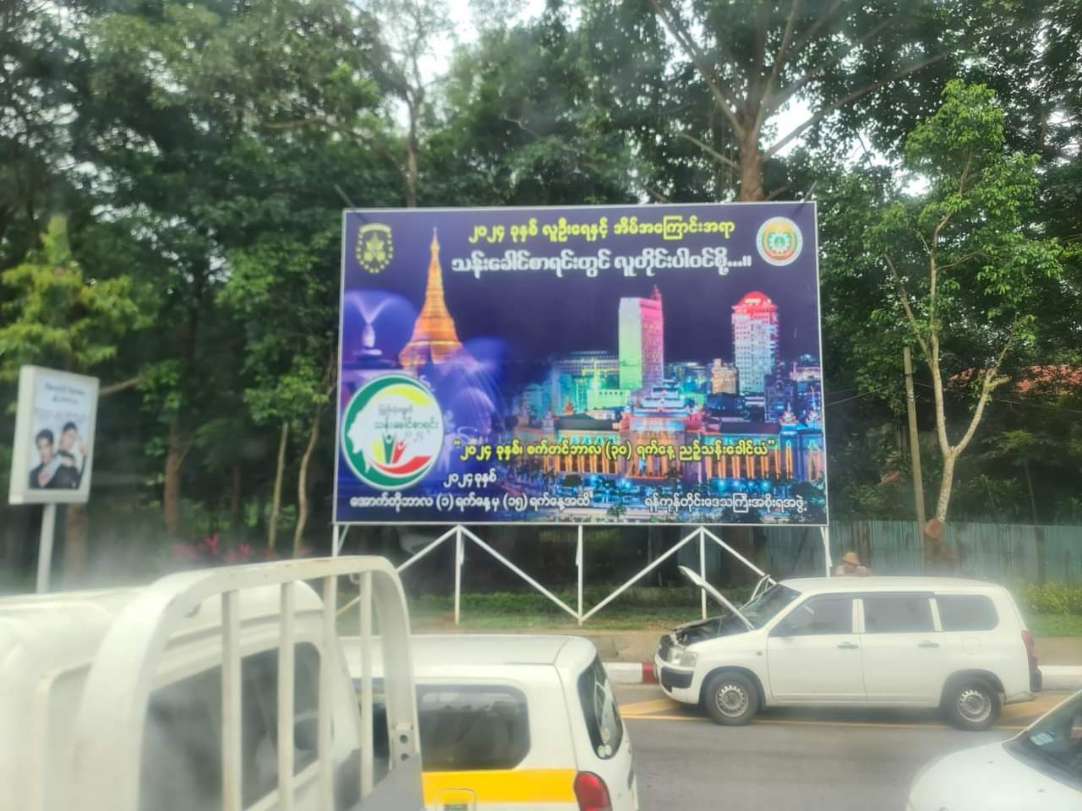
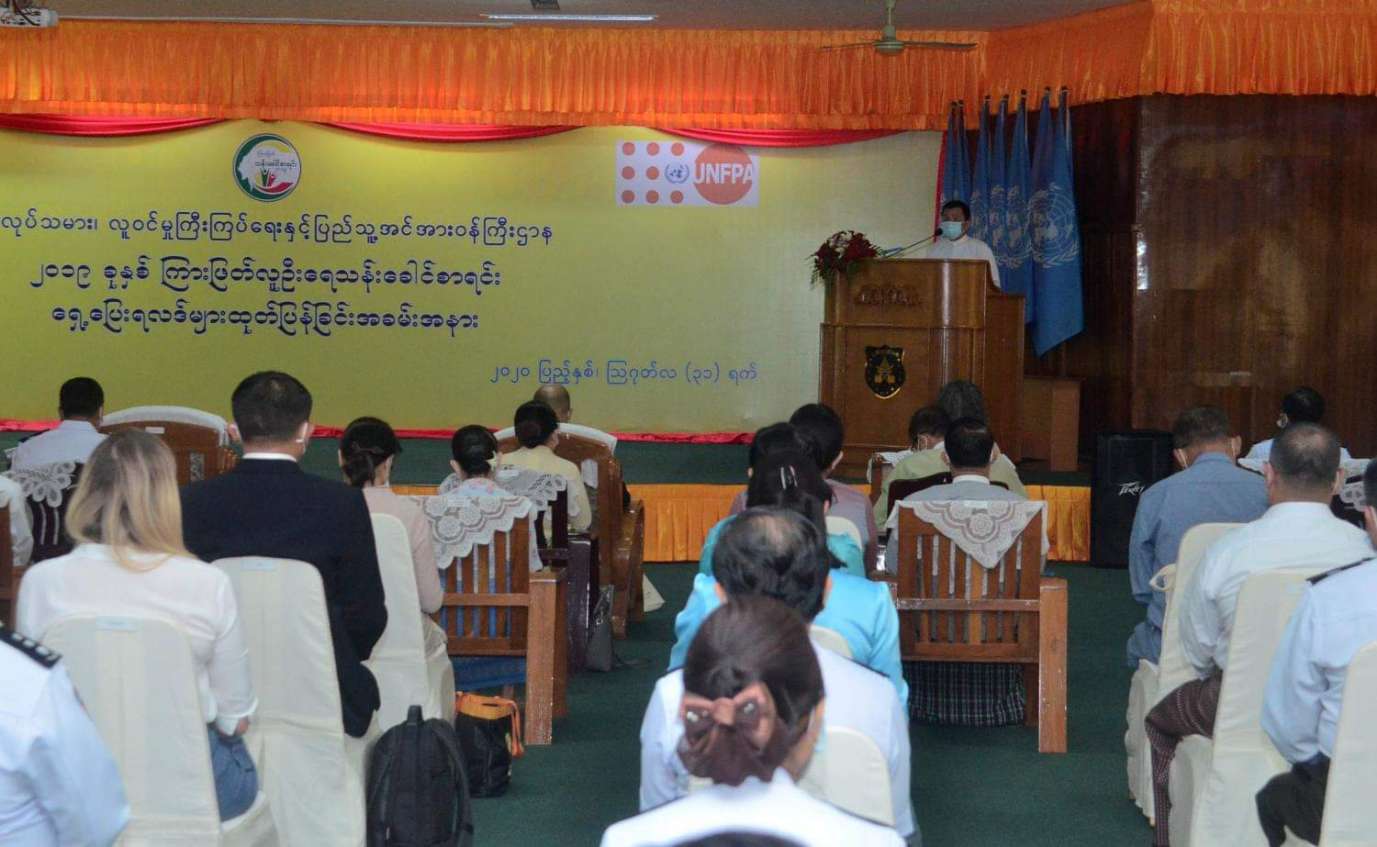
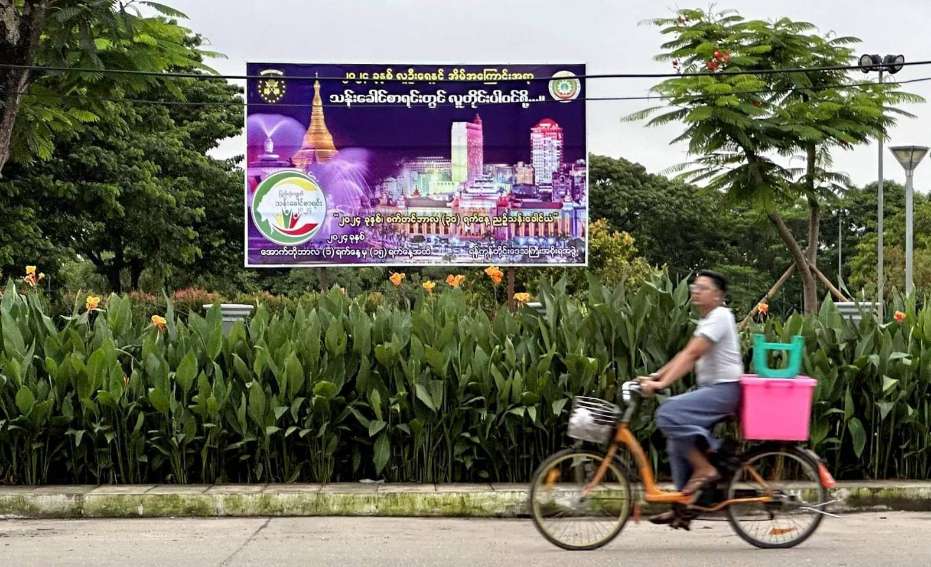
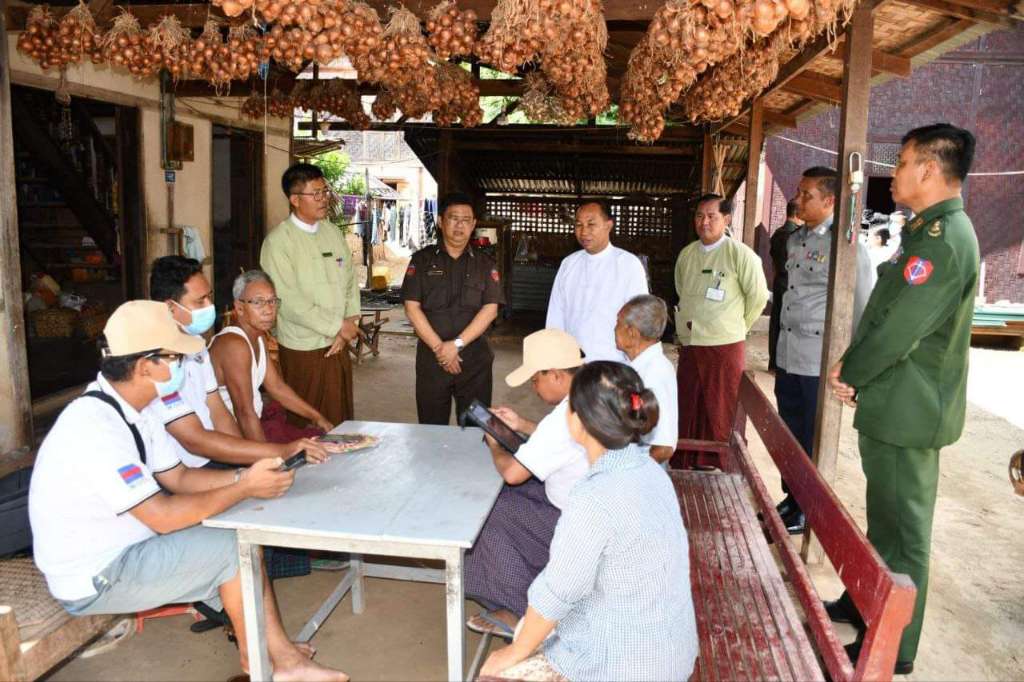
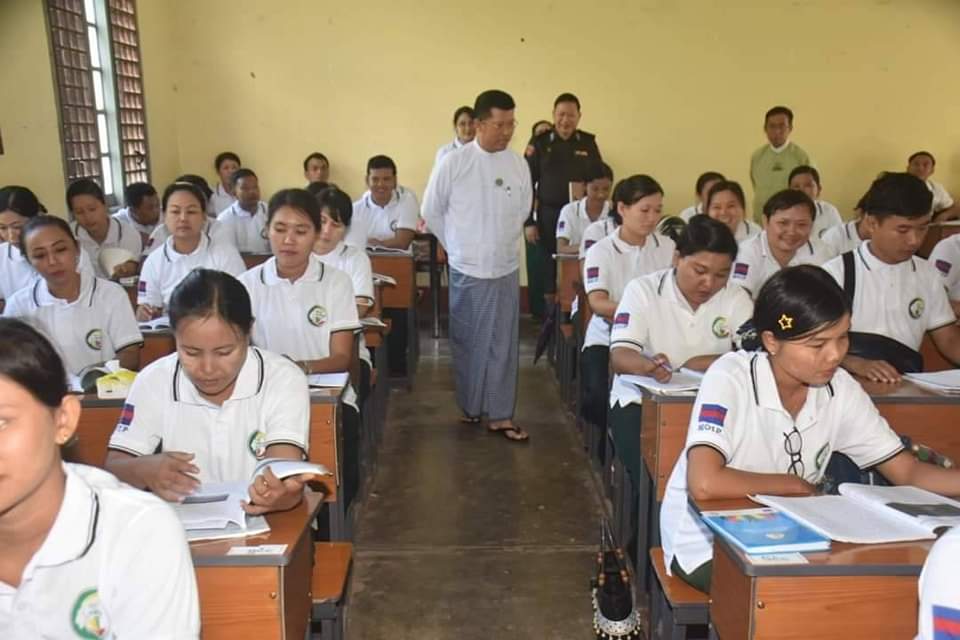
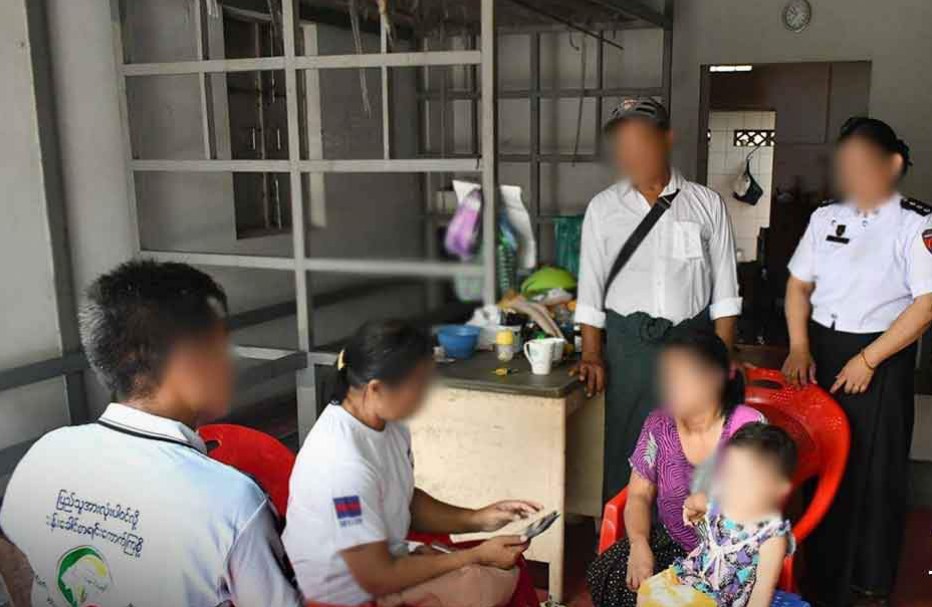
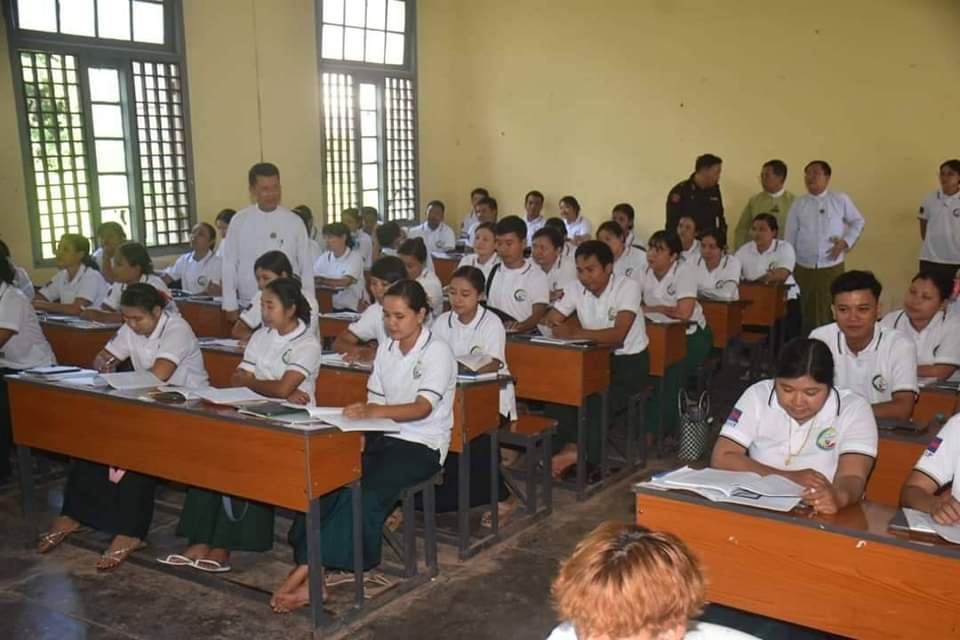
Smart grids, powered by AI algorithms, can balance supply and demand more efficiently, integrating renewable energy sources and minimizing waste.
2. **Natural Resource Management**: AI technologies help monitor and manage natural resources more efficiently. For example, AI can analyze satellite imagery to assess water usage, track deforestation, or monitor wildlife populations, aiding in conservation efforts.
3. **Climate Modeling and Prediction**: AI can improve climate models by analyzing vast datasets and identifying complex patterns. This capability enhances our understanding of climate change and helps predict its impacts more accurately.
4. **Sustainable Agriculture**: AI-driven tools, such as precision agriculture, use data analytics to optimize crop yields while minimizing environmental impact. Drones and satellite imagery coupled with AI can monitor crop health, manage pests, and reduce chemical usage.
5. **Waste Management**: AI can help improve recycling processes by identifying and sorting materials more effectively, thereby reducing landfill waste. Smart waste management systems can optimize collection routes, reducing emissions from vehicles.
### Negative Impacts of AI on the Environment
1. **Energy Consumption**: Training large AI models requires significant computational power, which can lead to a high carbon footprint. Data centers housing AI systems often consume substantial amounts of electricity, contributing to greenhouse gas emissions, especially if the energy comes from fossil fuels.
2. **E-waste Generation**: The rapid pace of AI development encourages frequent updates and hardware upgrades, leading to increased electronic waste. Proper recycling and disposal methods are often insufficient to mitigate environmental harm.
3. **Resource Use**: The extraction of minerals needed for advanced computing hardware (e.g., lithium, cobalt) can lead to environmental degradation, habitat destruction, and pollution in mining regions.
4. **Bias and Inequality in Deployment**: While this may not be a direct environmental impact, the deployment of AI algorithms can exacerbate existing inequalities in accessing environmental resources and services, affecting marginalized communities disproportionately.
### Mitigation Strategies
– **Energy Efficiency Improvements**: Developing more energy-efficient AI models and hardware can reduce the carbon footprint associated with AI training and deployment.
– **Renewable Energy**: Transitioning data centers to renewable energy sources can help offset the energy consumption of AI systems.
– **Regulations and Standards**: Governments and organizations can create regulations that promote sustainable practices in AI development and deployment.
– **Sustainable AI Research**: Encouraging research into low-energy AI models, such as federated learning and model distillation, can lead to more environmentally friendly AI solutions.
### Conclusion
AI holds significant potential to address many environmental challenges, but it also poses risks that need to be carefully managed. Balancing the innovative capabilities of AI with its environmental impacts is essential for creating sustainable solutions. As the technology continues to evolve, a multi-stakeholder approach involving researchers, policymakers, and industries will be crucial for maximizing benefits while minimizing harm to the environment.
Leave a Reply