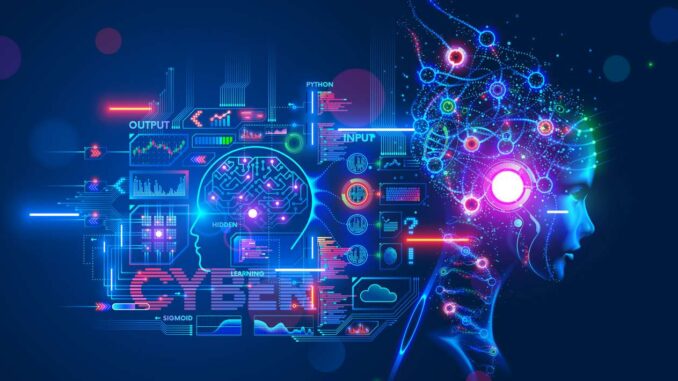
AI indeed provides evidence-based responses by leveraging data and algorithms to generate insights, recommendations, and actions based on
factual information and patterns derived from past experiences. Here are some key aspects of how AI delivers evidence-based responses:
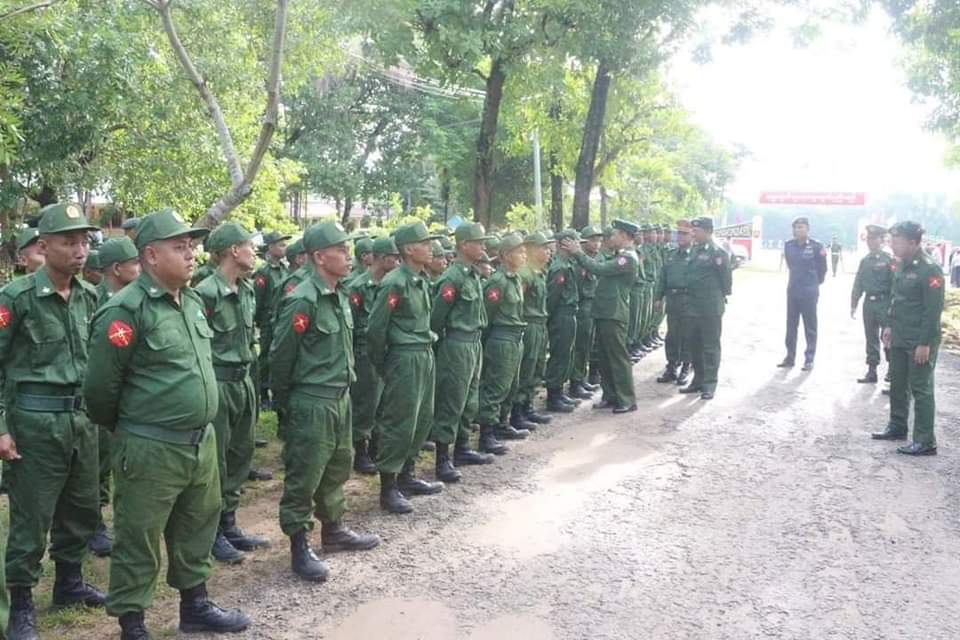
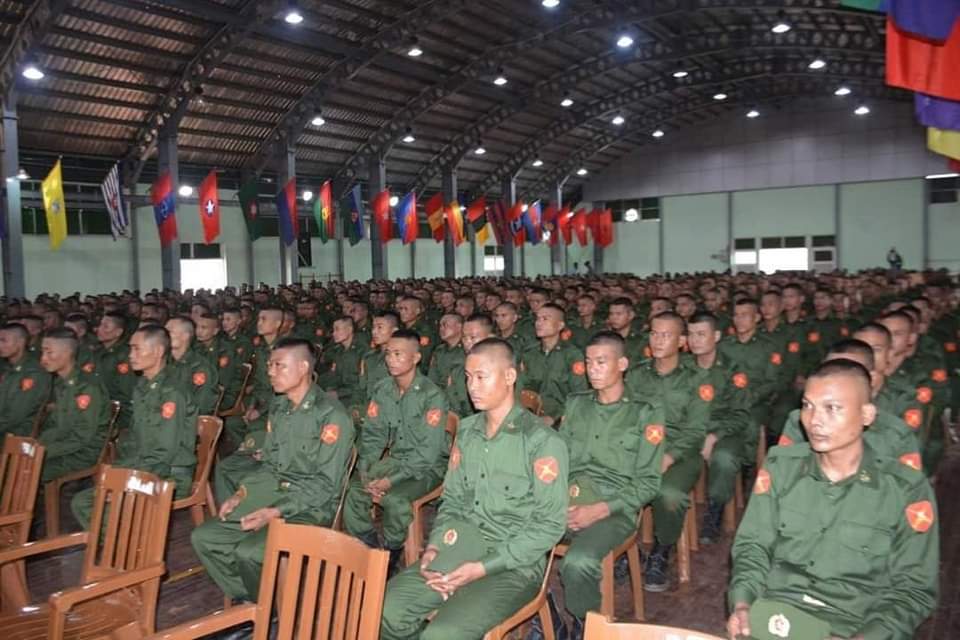
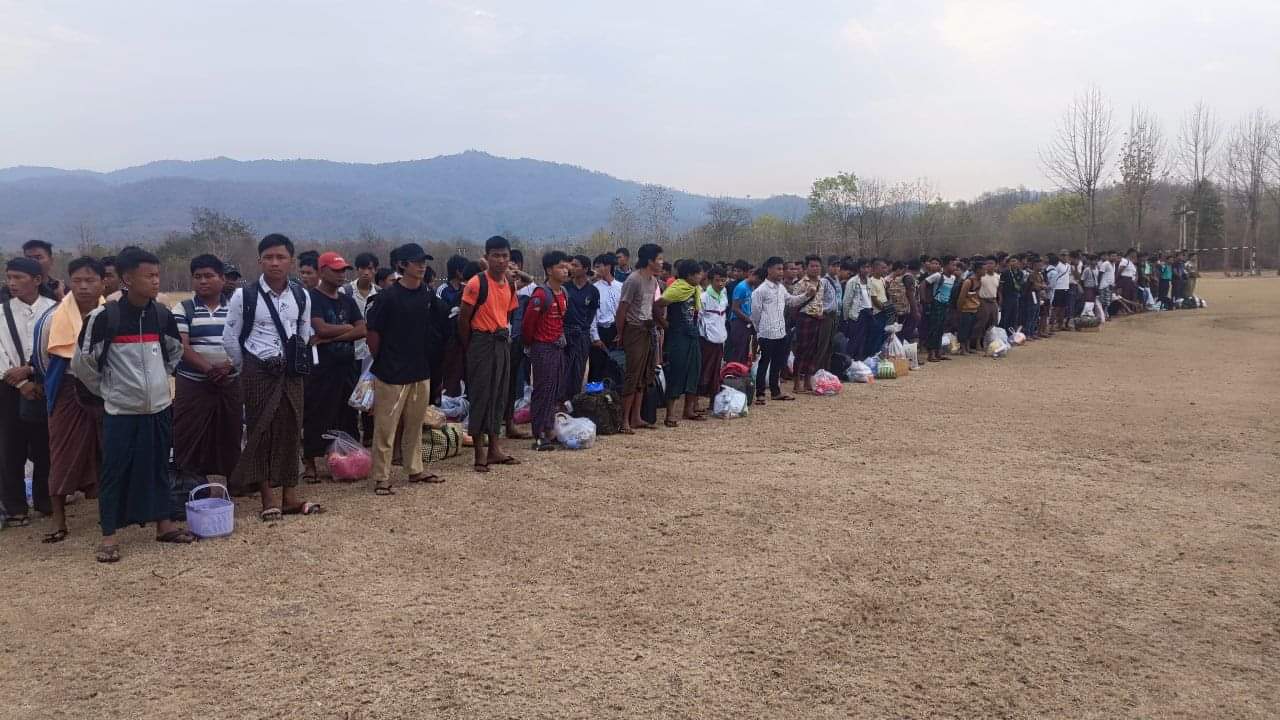
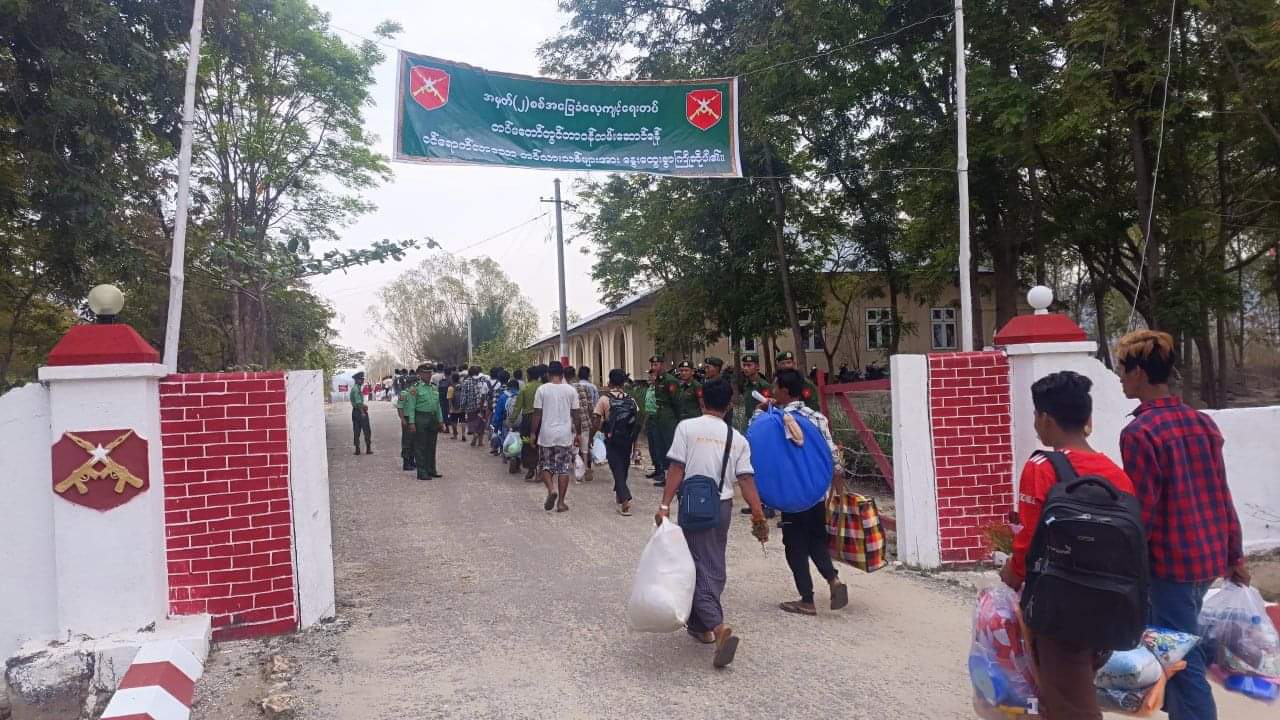

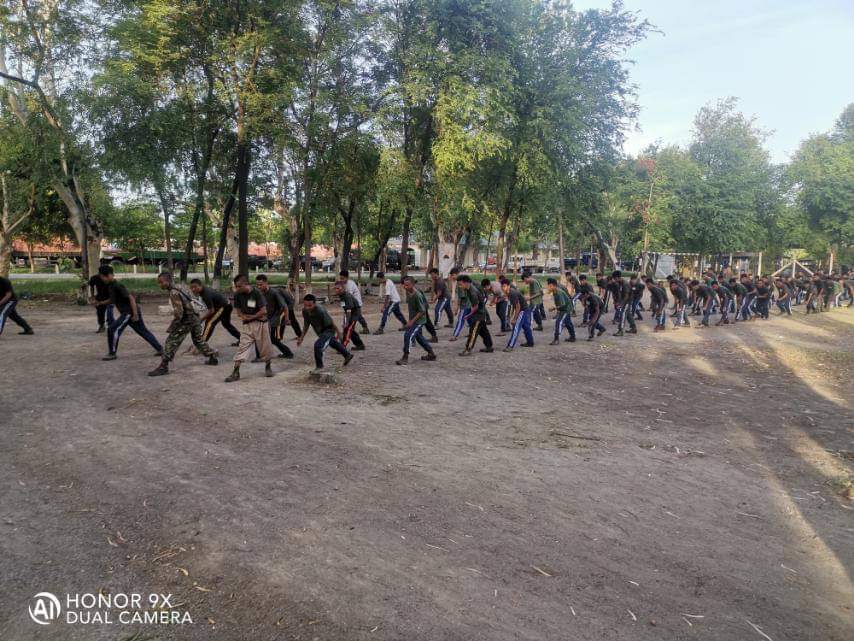
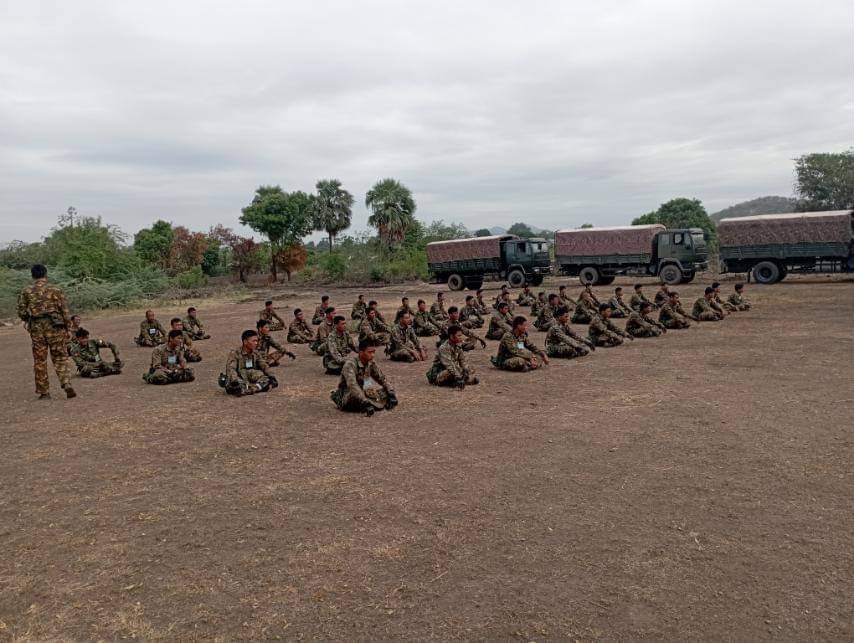
### 1. **Data-Driven Insights**
AI systems analyze large datasets to extract meaningful insights and trends. By gathering and processing data from various sources, AI can identify patterns that can form the basis for decision-making.
– **Example**: In healthcare, AI can analyze patient histories and treatment outcomes to recommend the most effective treatments based on previous cases.
### 2. **Predictive Modeling**
AI employs predictive analytics to forecast future events or outcomes based on historical data. This involves using statistical algorithms and machine learning techniques to identify relationships and make informed predictions.
– **Example**: Retailers use AI to predict inventory needs based on sales trends, ensuring they have the right products in stock to meet customer demand.
### 3. **Natural Language Processing (NLP)**
NLP allows AI to interpret and extract information from text data, which can include research papers, articles, and reports. This capability enables AI to provide evidence-based responses grounded in the latest literature or public sentiment.
– **Example**: An AI tool can analyze thousands of research studies to summarize the current understanding of a medical condition, guiding a physician’s treatment plan.
### 4. **Automated Decision-Making**
AI can automate decision-making processes by using algorithms that prioritize evidence over intuition. This can lead to faster, more accurate decisions in various sectors, including finance, healthcare, and logistics.
– **Example**: Financial institutions employ AI algorithms to evaluate loan applications based on credit scores and other financial data, reducing bias in decision-making.
### 5. **Simulation and Scenario Analysis**
AI can simulate various scenarios based on different inputs, helping organizations understand potential outcomes before making decisions.
– **Example**: In urban planning, AI can model traffic patterns under different development scenarios to predict congestion and inform infrastructure investment.
### 6. **Continuous Learning and Adaptation**
AI systems are designed to learn and adapt over time as they are exposed to more data. This continuous learning allows them to refine their approaches and provide more accurate, evidence-based responses.
– **Example**: Machine learning algorithms in recommendation systems learn user preferences to suggest products or services based on past behaviors, improving relevance and user satisfaction.
### 7. **Risk Assessment and Management**
AI helps organizations assess risks by analyzing patterns and anomalies in data. This can be particularly useful in fields like finance, cybersecurity, and compliance.
– **Example**: In cybersecurity, AI analyzes network traffic to detect unusual patterns that may indicate a security breach, allowing for quick, evidence-based responses to mitigate risks.
### 8. **Patient Diagnosis and Treatment**
AI technologies such as image recognition and diagnostic algorithms enhance the accuracy of medical diagnoses by comparing patient data against vast databases of medical knowledge.
– **Example**: AI-driven tools can analyze medical images (like X-rays or MRIs) to identify potential abnormalities, providing doctors with data-backed insights to support their diagnostic processes.
### Conclusion
AI enables organizations to rely on data and statistical evidence, improving the reliability of decision-making and operational efficiency. By providing evidence-based responses, AI reduces the likelihood of errors based on human biases and enhances the capability to make informed choices across various industries. This approach not only improves outcomes but also fosters trust in AI systems as valuable decision-support tools.
Leave a Reply