
The AI value chain refers to the series of steps and processes involved in developing, deploying, and benefiting from artificial intelligence technologies.
Understanding this value chain can help organizations leverage AI effectively to enhance their operations, create new products or services, and gain a competitive advantage.
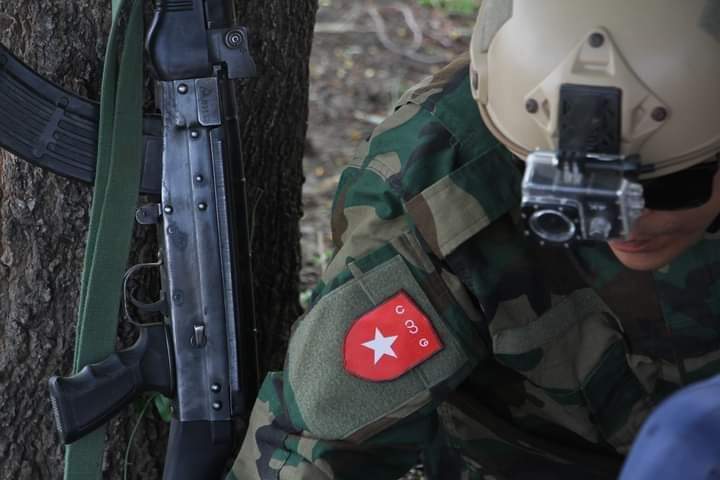

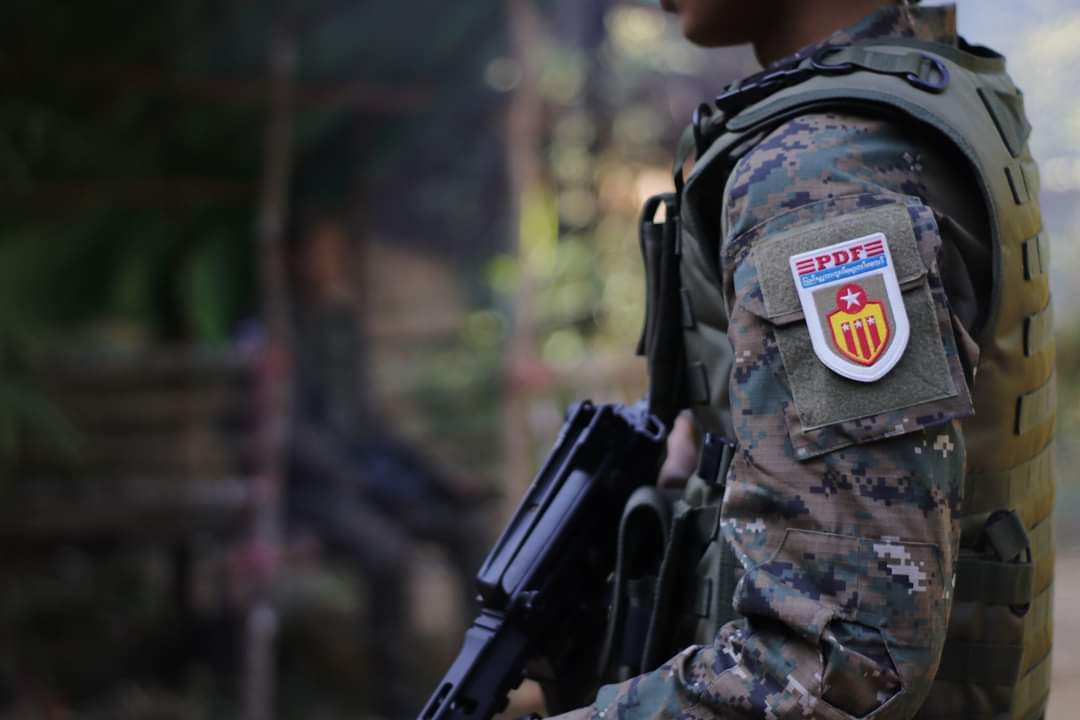

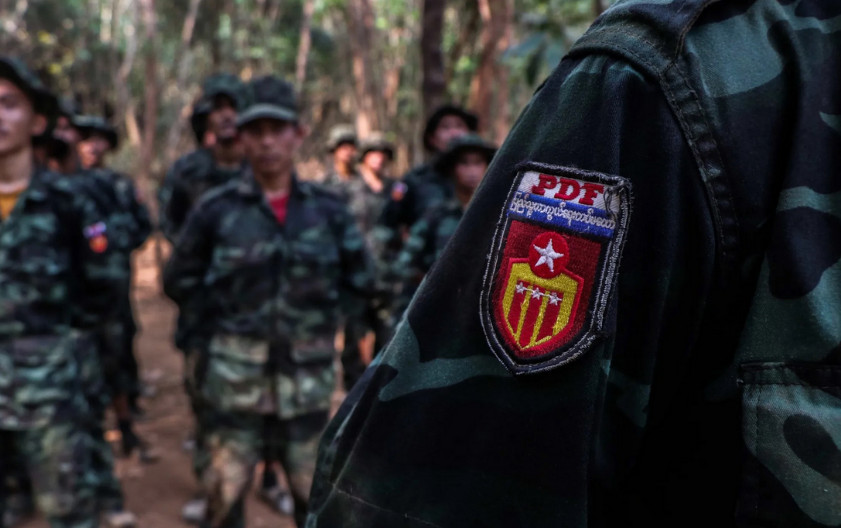


Here’s a breakdown of the key components of the AI value chain:
### 1. **Data Collection & Management**
– **Data Sources:** Identification and collection of relevant data from various sources, including internal databases, IoT devices, customer interactions, social media, etc.
– **Data Quality:** Ensuring data is accurate, complete, and consistent. This may involve data cleaning and preprocessing to eliminate duplicates and errors.
– **Data Governance:** Establishing policies for data use, security, privacy, and compliance with regulations (e.g., GDPR).
### 2. **Data Processing & Annotation**
– **Data Processing:** Transforming raw data into a usable format through normalization, aggregation, and transformation.
– **Data Annotation:** Labeling data for supervised learning tasks. This often requires manual or semi-automated efforts to ensure that AI models can learn effectively from the data.
### 3. **Model Development**
– **Algorithm Selection:** Choosing appropriate algorithms and techniques (e.g., machine learning, deep learning, natural language processing) based on the problem at hand.
– **Training:** Using labeled datasets to train models, adjusting parameters to optimize performance.
– **Validation:** Testing the models against a separate dataset to evaluate accuracy and robustness.
### 4. **Model Deployment**
– **Integration:** Deploying the AI model into existing systems or workflows, ensuring compatibility and functionality.
– **Scalability:** Ensuring that the model can handle significant loads and that infrastructure can scale with demand.
– **Monitoring:** Continuously monitoring model performance post-deployment to ensure it meets business objectives and can adapt to changing conditions.
### 5. **Decision Making & Insights**
– **Inference:** Using the deployed model to make predictions, automate tasks, or generate insights that inform decision-making.
– **Interpretability:** Ensuring that the AI’s decisions and recommendations are understandable to stakeholders, which is crucial for trust and accountability.
### 6. **Feedback Loop**
– **Performance Evaluation:** Regularly assessing the model’s performance against key performance indicators (KPIs) and business goals.
– **Retraining:** Continuously updating the model with new data to improve accuracy and adapt to new trends or patterns.
– **User Feedback:** Incorporating feedback from users to refine models and improve user experience.
### 7. **Value Realization**
– **Business Impact:** Assessing how AI affects business outcomes, such as efficiency, cost reduction, revenue increases, customer satisfaction, etc.
– **Innovation:** Exploring new opportunities for AI applications based on the insights gained from AI initiatives.
### 8. **Ethics & Compliance**
– **Ethical Considerations:** Addressing issues like bias, fairness, transparency, and accountability in AI usage.
– **Regulatory Compliance:** Ensuring adherence to legal requirements and industry standards related to data privacy, security, and ethical AI use.
### Conclusion
Understanding the AI value chain allows organizations to systematically approach AI initiatives, ensuring that all aspects—from data collection to value realization—are aligned and effectively managed. By doing so, they can maximize the benefits of AI technology while mitigating risks and ethical concerns.
Leave a Reply