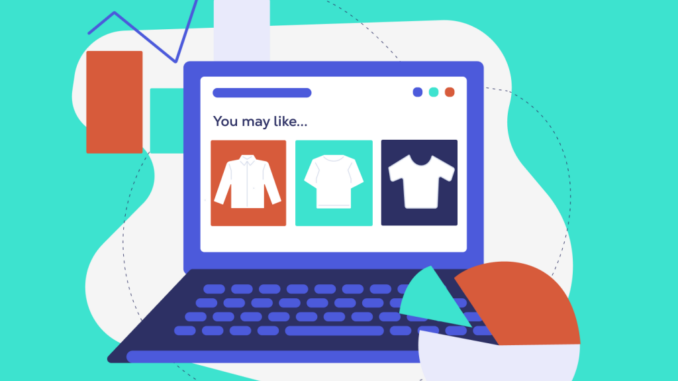
AI product recommendations use machine learning and artificial intelligence techniques to analyze user behavior, preferences, and historical data to suggest products or services that an individual customer is likely to be interested in.
These systems are crucial for enhancing user experience, increasing sales, and fostering brand loyalty in various industries, especially in e-commerce, media streaming, and online services.
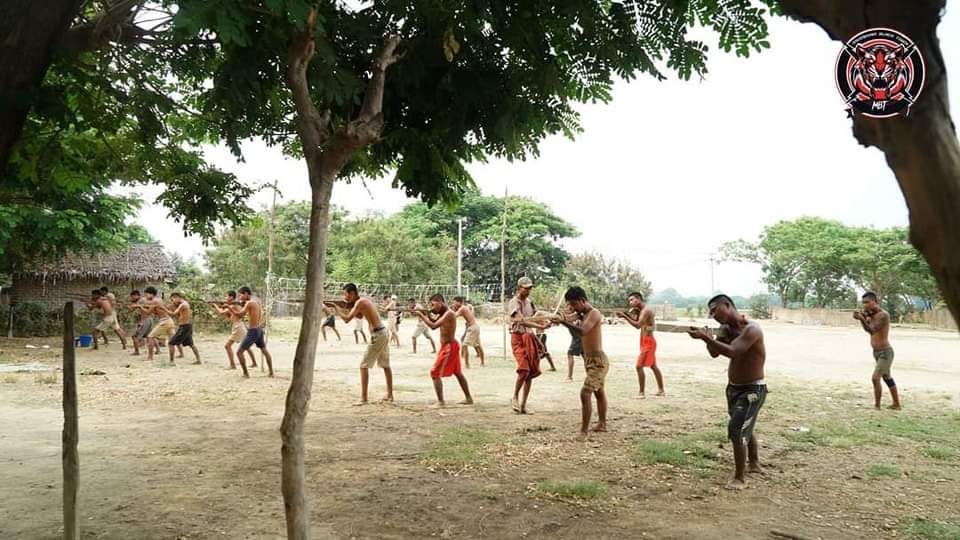
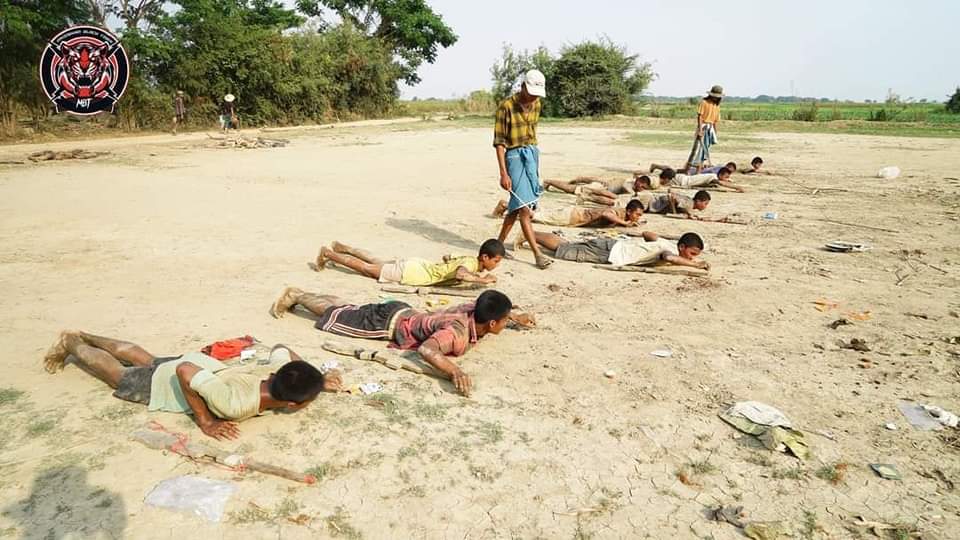
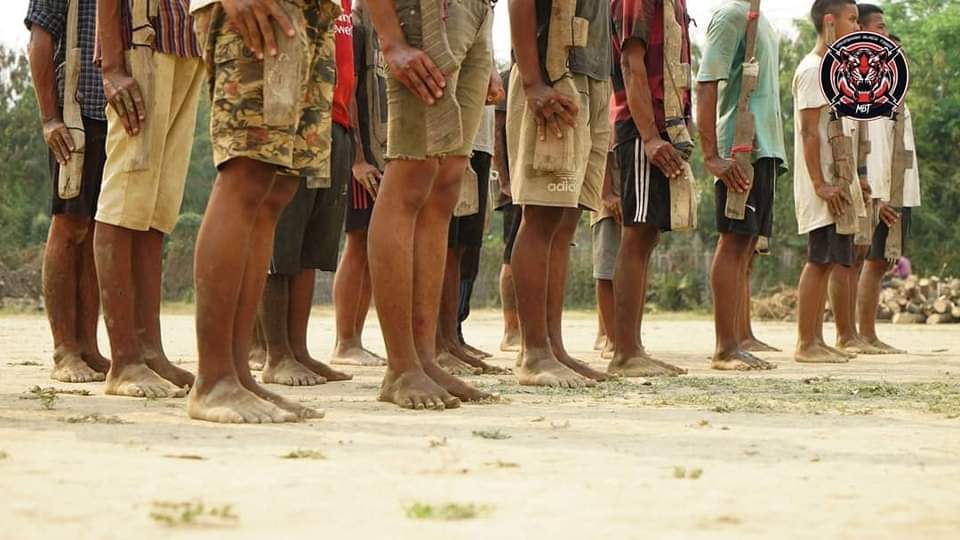
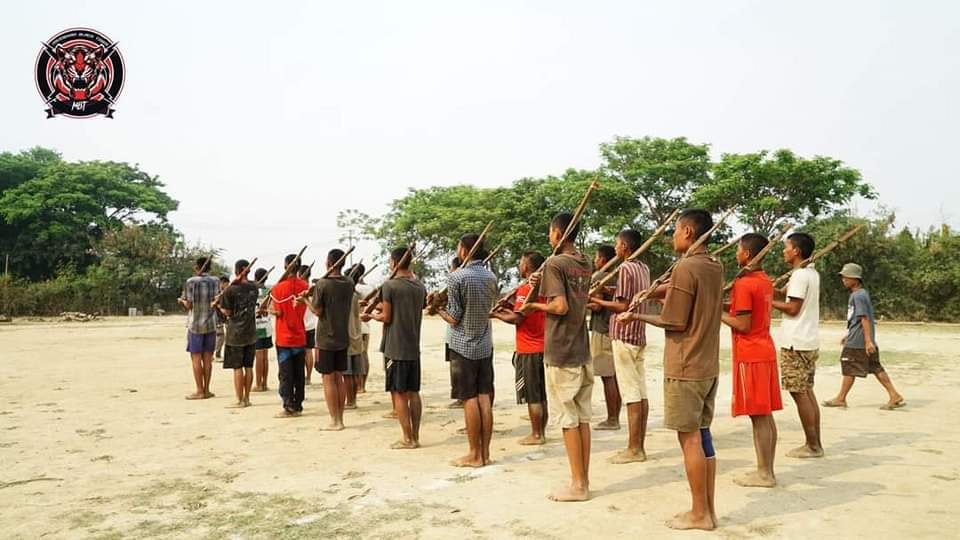
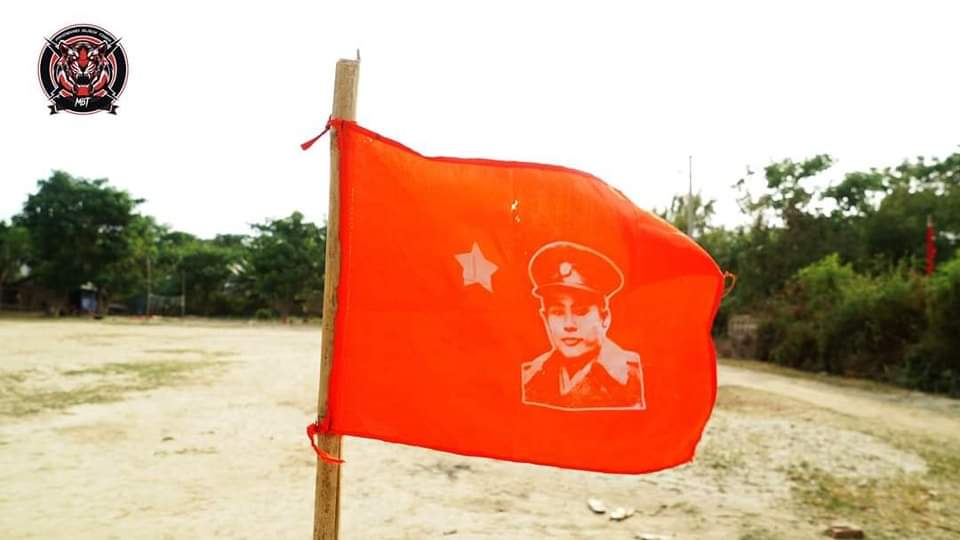
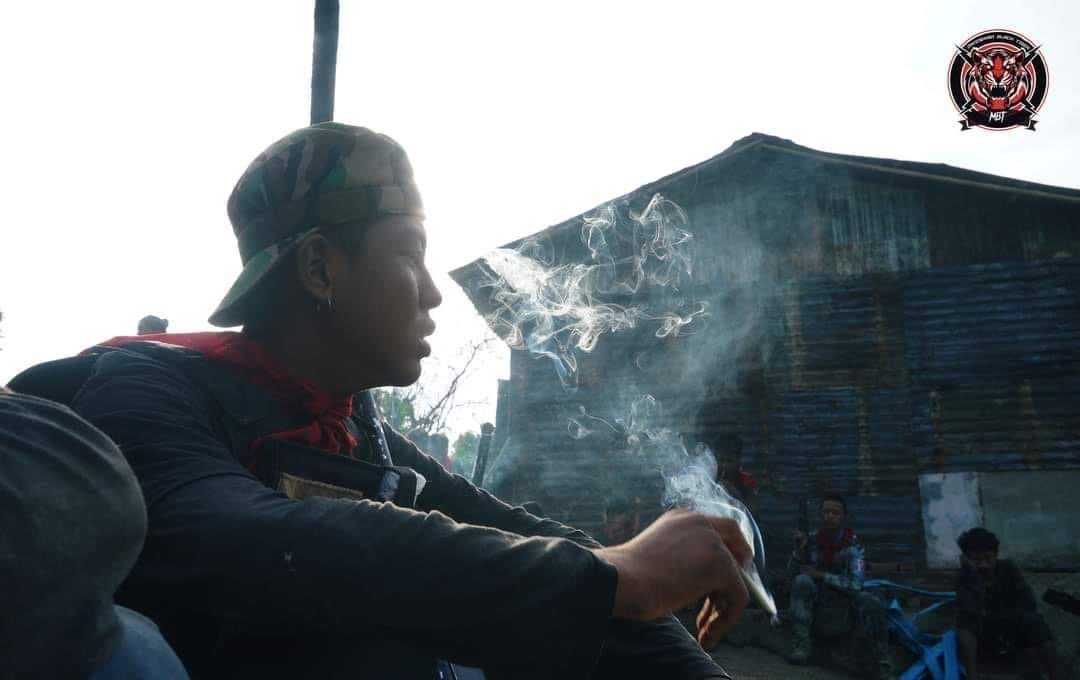
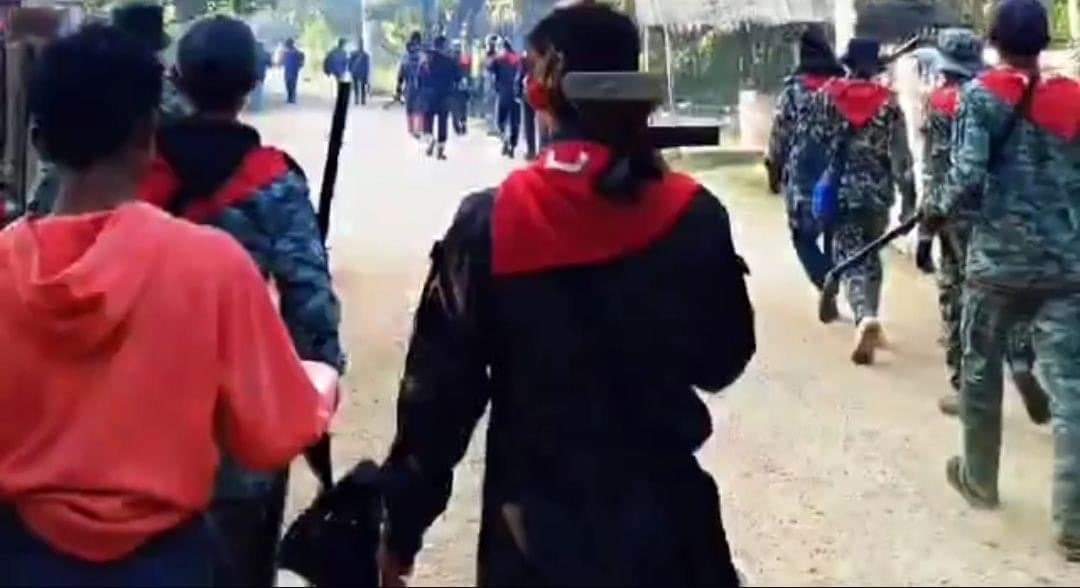
### Key Components of AI Product Recommendations
1. **Data Collection**:
– Gathering data from various sources such as user interactions (clicks, purchases, ratings), demographics, and contextual information (location, time) to build a comprehensive profile of user behavior.
2. **Data Processing**:
– Cleaning and preparing the data to remove inconsistencies, duplicates, and irrelevant information. This stage may involve normalizing data and transforming it into suitable formats for analysis.
3. **Recommendation Algorithms**:
– Different algorithms can be employed to generate product recommendations. The most common types include:
– **Collaborative Filtering**:
– **User-Based Collaborative Filtering**: Recommendations are based on similar users. For example, if User A and User B have similar preferences, the system suggests products that User B liked to User A.
– **Item-Based Collaborative Filtering**: Recommendations are based on product similarities. If two products have been frequently purchased together, they are likely to be recommended to users who bought one of them.
– **Content-Based Filtering**:
– Recommendations are based on the attributes of the products themselves. If a user previously liked action movies, the system suggests other action movies by analyzing their features (e.g., genre, actors, director).
– **Hybrid Methods**:
– Combining collaborative filtering and content-based filtering to leverage the strengths of both approaches. These systems can provide more accurate and diverse recommendations.
– **Deep Learning**:
– Advanced neural network architectures, such as convolutional neural networks (CNNs) and recurrent neural networks (RNNs), can capture complex patterns in user behavior and product features, enabling sophisticated recommendations.
– **Contextual Bandits**:
– A reinforcement learning approach that balances exploration and exploitation, adapting recommendations based on user actions in real-time.
4. **Evaluation**:
– Measuring the effectiveness of the recommendation system using metrics such as precision, recall, and F1-score. Techniques such as A/B testing can also be employed to assess user engagement and satisfaction.
### Applications of AI Product Recommendations
1. **E-commerce**:
– Major online retailers (like Amazon) use AI-driven recommendations to suggest products based on browsing history, past purchases, and preferences.
2. **Media Streaming**:
– Platforms like Netflix and Spotify recommend movies, TV shows, or music based on users’ viewing or listening history and preferences.
3. **Social Media**:
– Platforms like Facebook and Instagram suggest content and advertisements tailored to individual users based on their interactions and interests.
4. **Travel and Hospitality**:
– Websites like Expedia recommend hotels, flights, and travel packages based on previous searches, bookings, and user preferences.
5. **Personal Finance**:
– Financial institutions provide product suggestions (like credit cards or loans) based on users’ financial behaviors and profiles.
### Benefits of AI Product Recommendations
1. **Personalization**:
– By tailoring recommendations to individual preferences, businesses can enhance user satisfaction and engagement, leading to higher conversion rates.
2. **Increased Sales**:
– Effective recommendation systems can lead to higher average order values (AOV) and more repeated purchases due to improved customer experiences.
3. **Enhanced User Engagement**:
– Personalized recommendations can keep users engaged by presenting them with products or content that are relevant to their interests.
4. **Improved Customer Retention**:
– Satisfied customers are more likely to return. Personalized experiences can foster loyalty and long-term relationships.
5. **Efficient Inventory Management**:
– By analyzing purchasing patterns and trends, businesses can optimize inventory based on anticipated demand.
### Challenges in AI Product Recommendations
1. **Data Quality and Privacy**:
– Gathering accurate and comprehensive data while respecting user privacy and complying with regulations (like GDPR) can be challenging.
2. **Cold Start Problem**:
– New users or products might not have enough data for effective recommendations. Strategies such as using demographic information or popular products can help mitigate this issue.
3. **Scalability**:
– As the amount of data and the number of users/products grow, the recommendation system must be able to scale efficiently without a significant drop in performance.
4. **Dynamic Contexts**:
– User preferences can change over time, requiring the system to adapt quickly to new behavior patterns.
5. **Bias in Recommendations**:
– Algorithms can inadvertently reinforce existing biases, leading to less diverse product exposure for users.
### Future Trends in AI Product Recommendations
1. **Stronger Emphasis on Explainability**:
– Increasing demand for transparency in how recommendations are generated will lead to more interpretable models.
2. **Incorporation of Multi-Modal Data**:
– Using various data types (such as images, video, and text) to enhance the recommendation process.
3. **Real-Time Personalization**:
– Emphasis on utilizing real-time data to deliver timely and contextually relevant recommendations.
4. **Integration of Voice and Visual Search**:
– Enhancing recommendations through voice-activated devices and visual search tools, making the experience more intuitive.
5. **Focus on Ethical AI**:
– Greater attention will be paid to ensuring fairness and mitigating biases in recommendation systems, fostering trust among users.
### Conclusion
AI product recommendations have become a cornerstone for businesses looking to enhance customer experiences and drive sales. By leveraging data and advanced algorithms, companies can tailor their offerings to meet the unique preferences of individual customers. As technology continues to evolve, the effectiveness and sophistication of recommendation systems will only improve, presenting new opportunities and challenges for organizations across various industries.
Leave a Reply