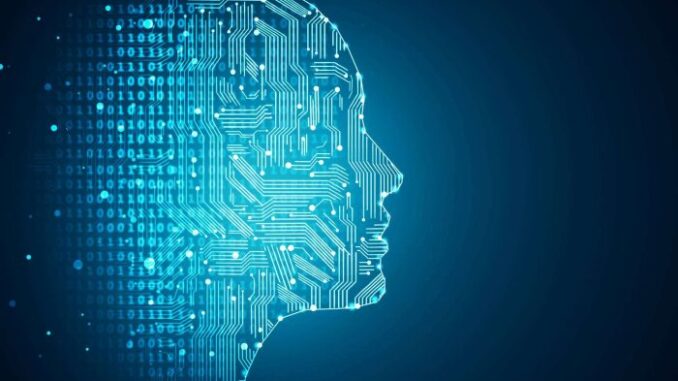
Limited Memory AI refers to systems that can utilize past experiences to inform future decisions but do not permanently store information.
This type of AI temporarily retains and processes specific data from previous interactions to improve its performance and adaptability in certain contexts.
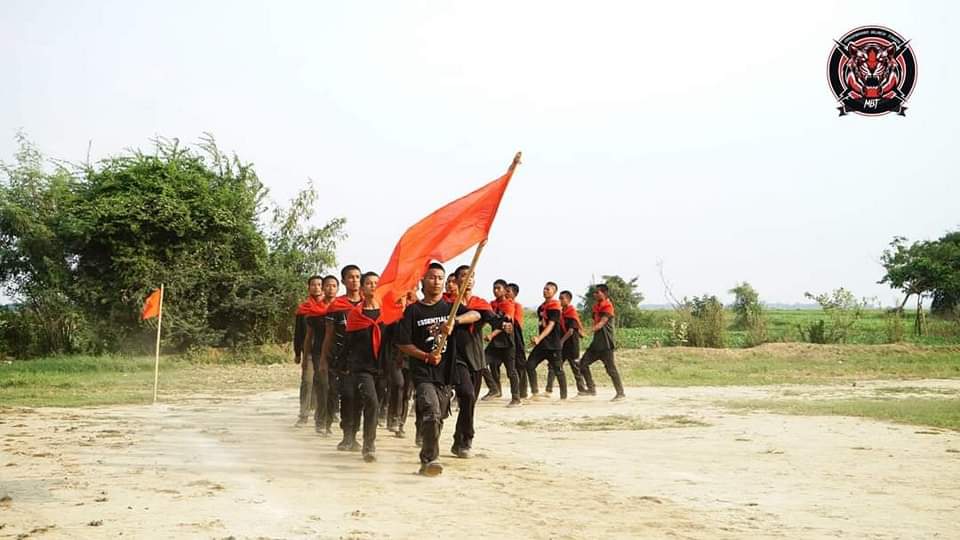
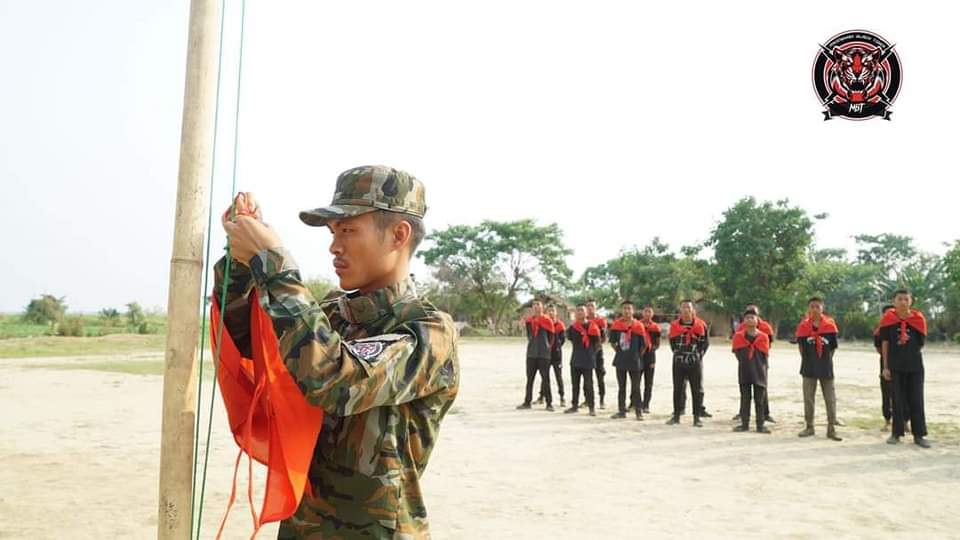
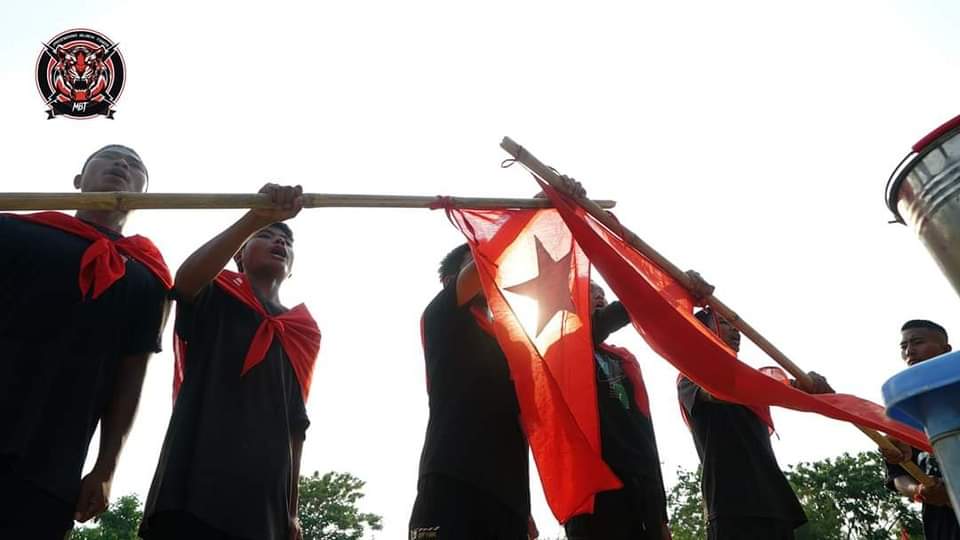
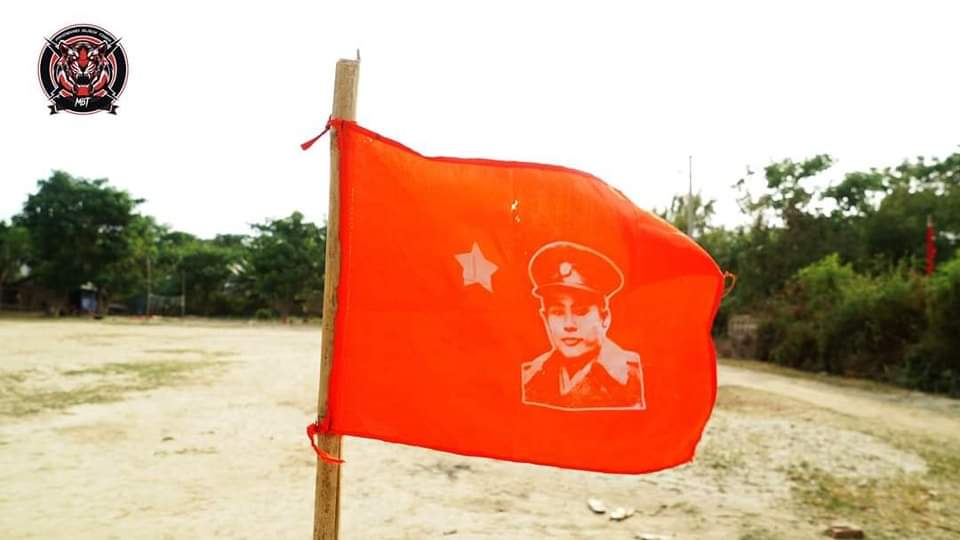
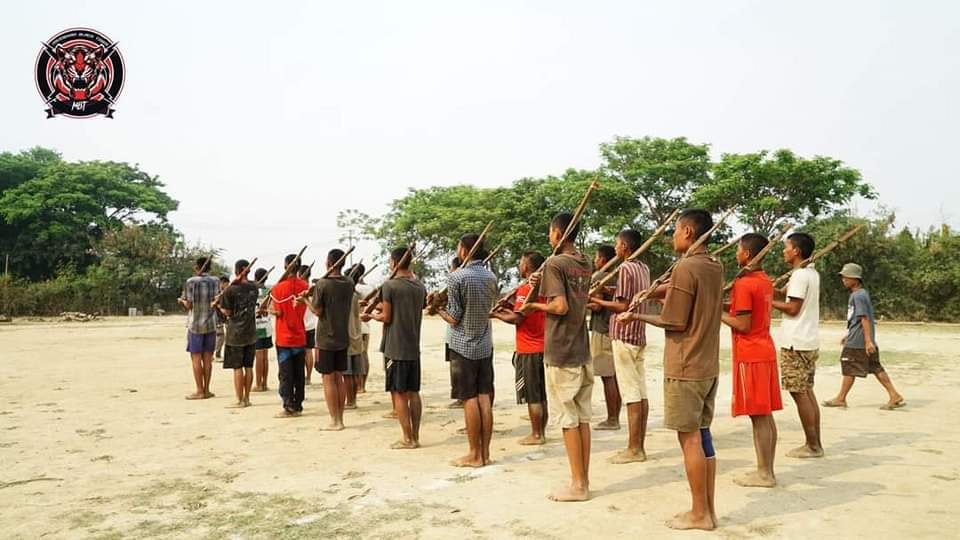
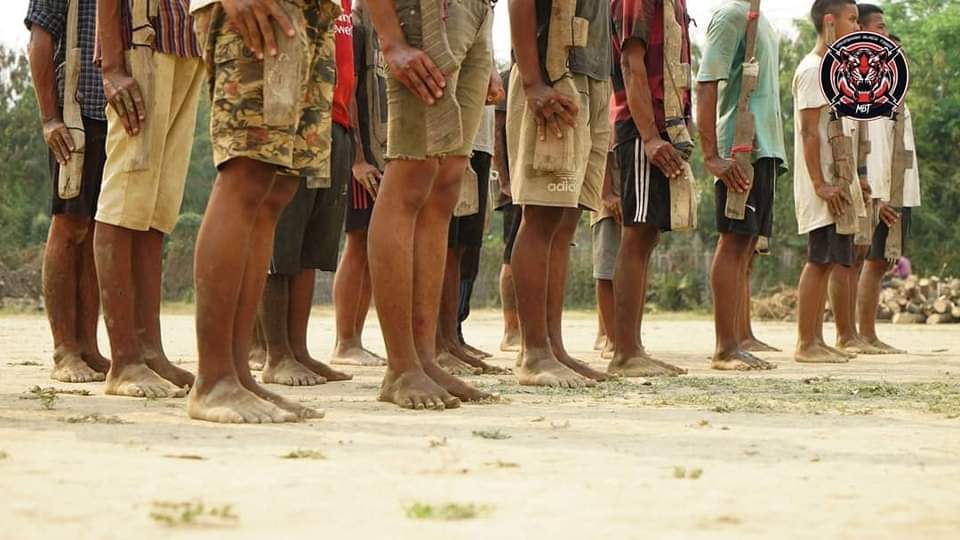
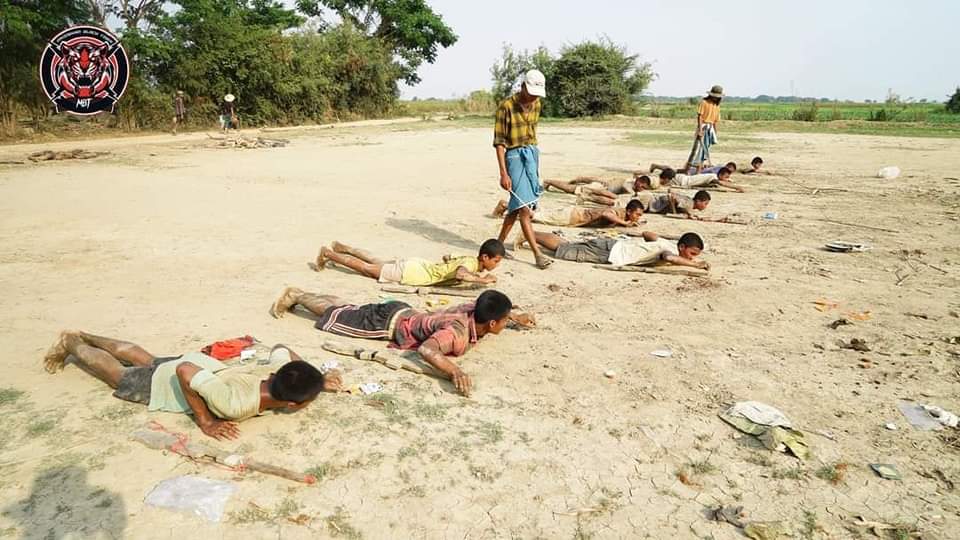
Limited memory AI is commonly used in many applications and is crucial for developing more responsive and context-aware systems.
### Characteristics of Limited Memory AI:
1. **Temporary Storage**: Limited memory AI retains information for a short duration, allowing it to use this data to make informed decisions during its operation.
2. **Learning from Experience**: It can learn from historical data without needing to remember this information permanently. This means it can adapt to new information or changes in its environment.
3. **Context Awareness**: Limited memory AI can respond to specific situations more effectively by drawing on relevant past interactions that are pertinent to the current context.
4. **Decision-Making**: It can analyze trends and patterns from past data to aid in decision-making processes.
### Examples of Limited Memory AI:
1. **Self-Driving Cars**:
– These vehicles use a combination of sensors and cameras to perceive their surroundings. They can temporarily store information about objects, road conditions, and traffic rules. For instance, although the car doesn’t remember a particular traffic light once passed, it can learn from past trips to drive more effectively in similar situations.
2. **Chatbots and Virtual Assistants**:
– Many chatbots store conversations temporarily to provide contextually relevant responses. If a user asks a follow-up question, the system can refer back to what was said earlier in the conversation, improving the overall interaction.
3. **Fraud Detection Systems**:
– Financial institutions often use limited memory AI to identify unusual patterns in spending behavior. These systems analyze recent transactions to flag potentially fraudulent activity based on learned behavior algorithms.
4. **Recommendation Systems**:
– Services like Netflix or Amazon analyze users’ past preferences and interactions but may not retain user data indefinitely due to privacy concerns. The limited memory allows these platforms to provide personalized recommendations based on recent behaviors.
5. **Gaming AI**:
– In video games, NPC (non-player character) behavior can change based on past player actions during a particular session. This adaptation allows the game to become more challenging and engaging.
### Advantages of Limited Memory AI:
– **Adaptability**: It can quickly respond to changing conditions without being burdened by extensive historical data.
– **Efficiency**: Less computational power is required compared to systems that retain vast amounts of data indefinitely.
– **Privacy**: By not retaining information long-term, these systems can mitigate privacy concerns and data protection issues.
### Challenges and Limitations:
– **Temporal Constraints**: The effectiveness of decision-making can be limited by the time frame within which the AI retains memory.
– **Data Management**: It needs robust algorithms to manage and utilize temporary data effectively without accruing unnecessary noise or irrelevant information.
– **Dependence on Quality Data**: The accuracy of the AI’s decisions heavily relies on the relevance and quality of the data it temporarily holds.
In conclusion, Limited Memory AI plays a critical role in various technological applications, allowing systems to be more responsive and contextually aware without the complexities that come with permanent memory retention. As AI technology advances, understanding and optimizing limited memory approaches will be vital for creating intelligent systems that interact more naturally and effectively with users and their environments.
Leave a Reply