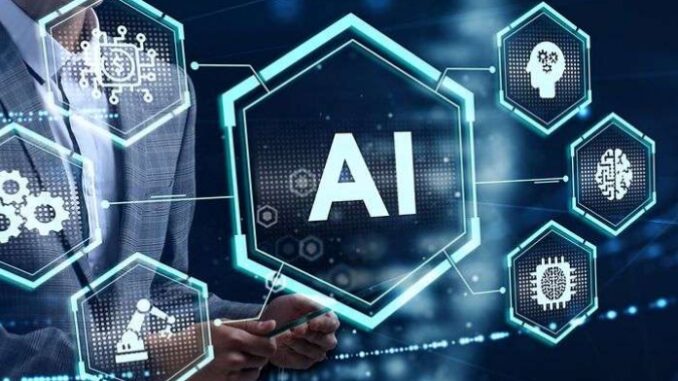
Advancements in AI research continue to push the boundaries of what is possible, leading to innovations that impact
a wide range of industries and aspects of daily life. Here are some key areas of advancement in AI research:
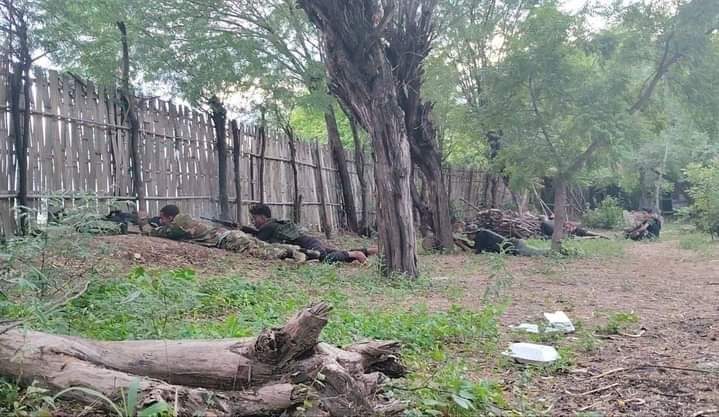
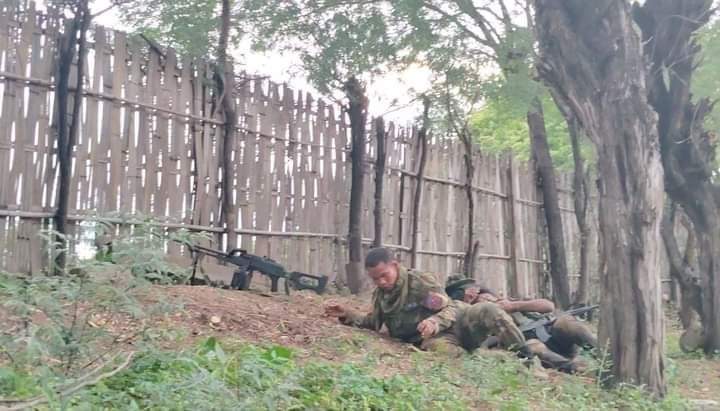
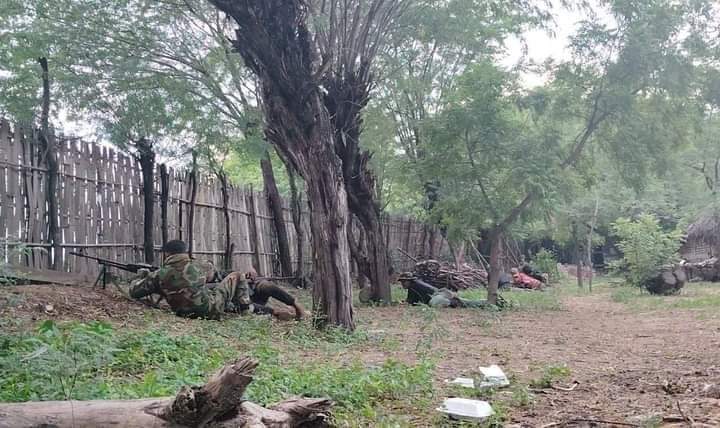
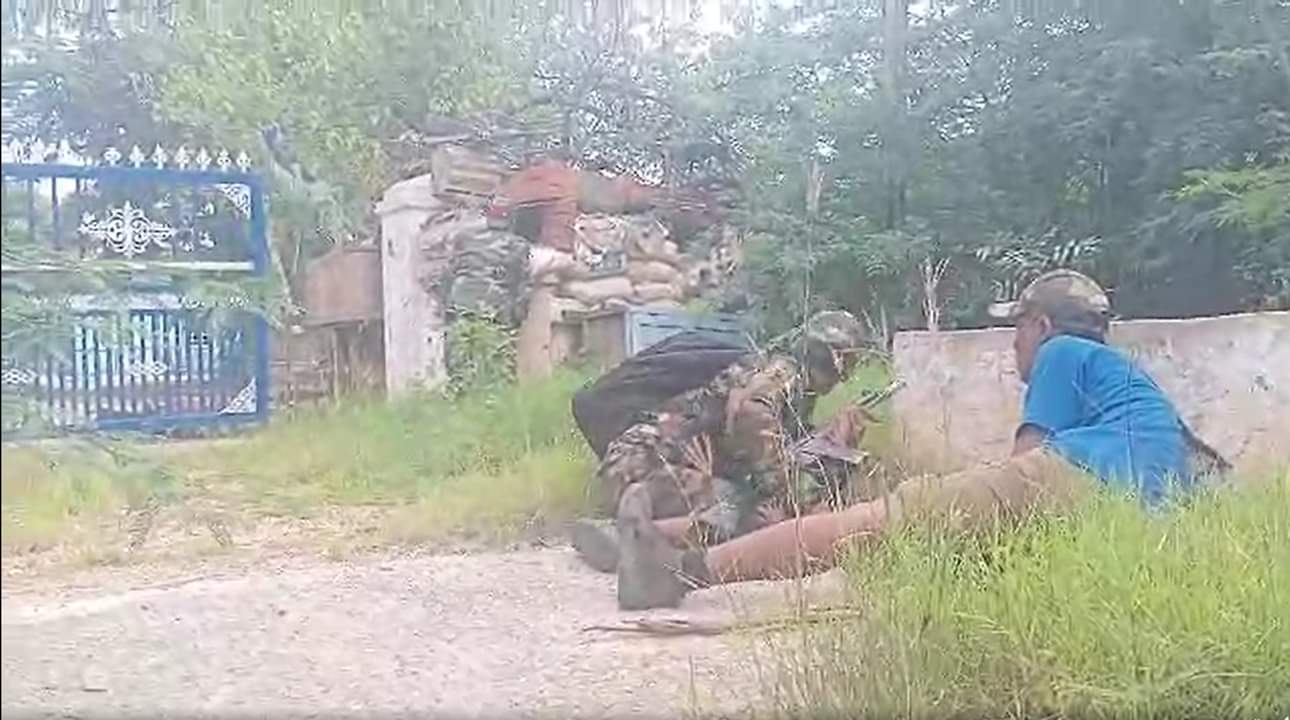
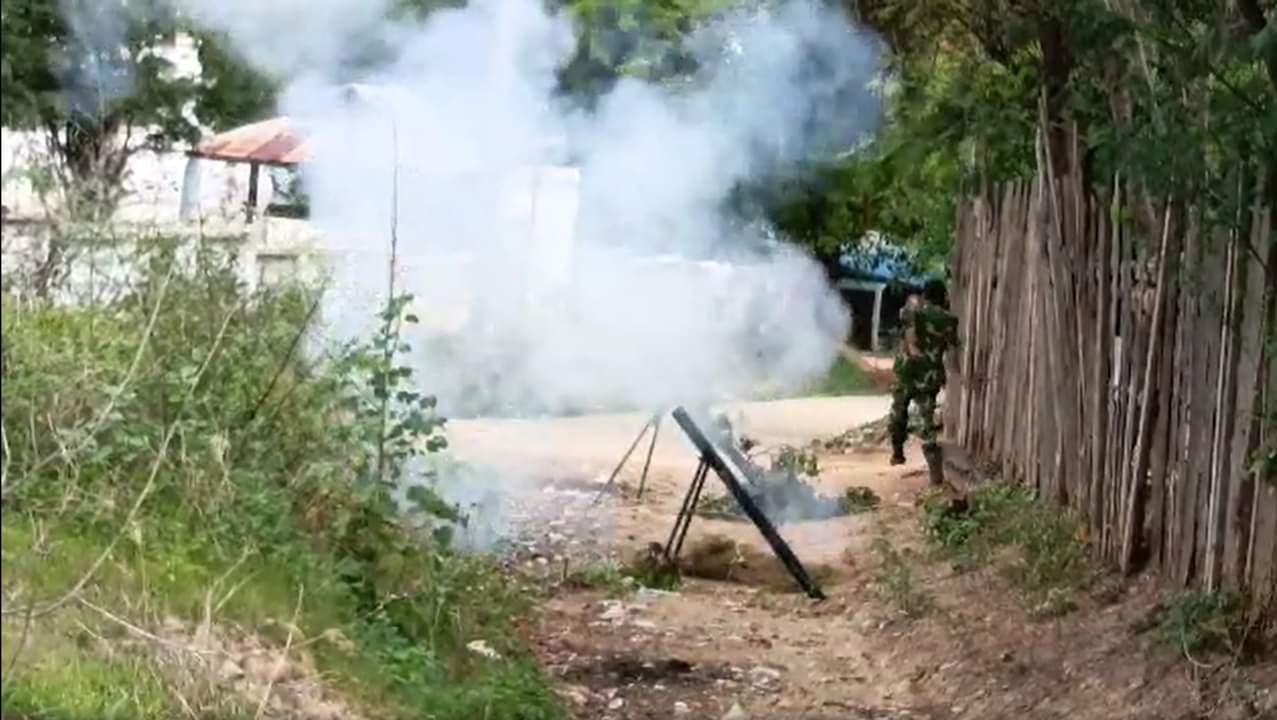
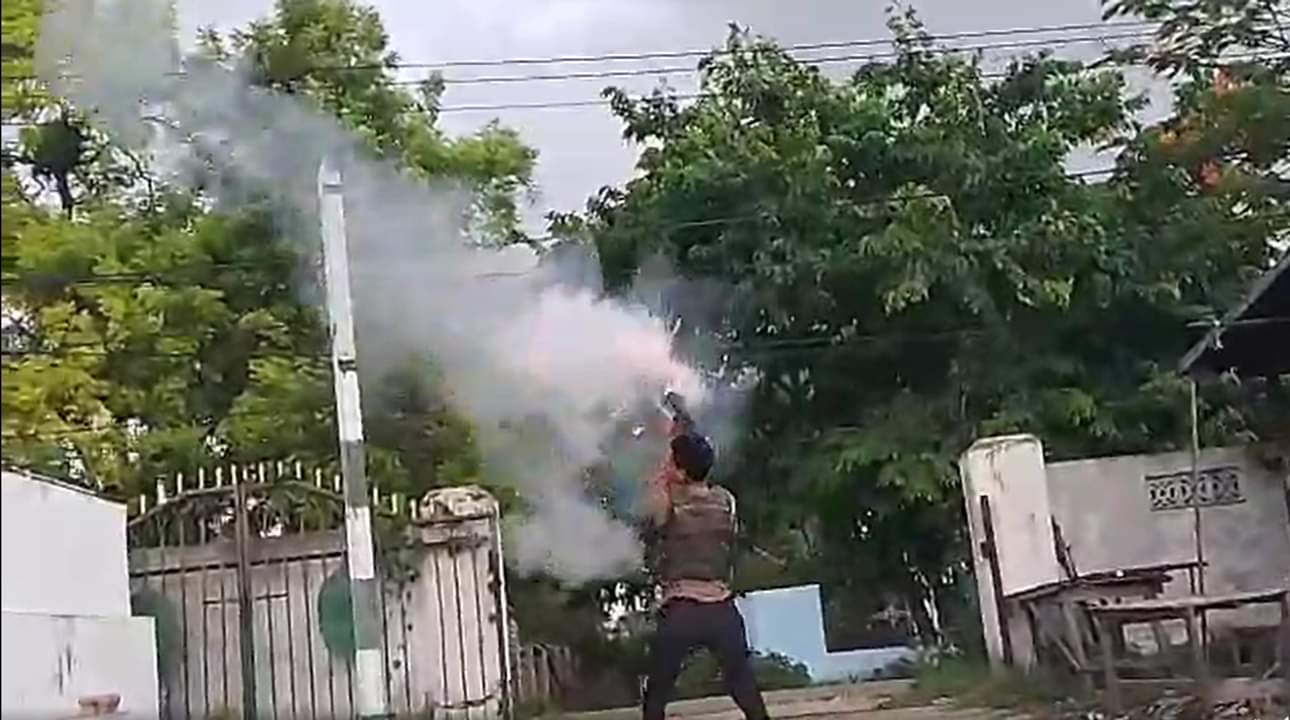
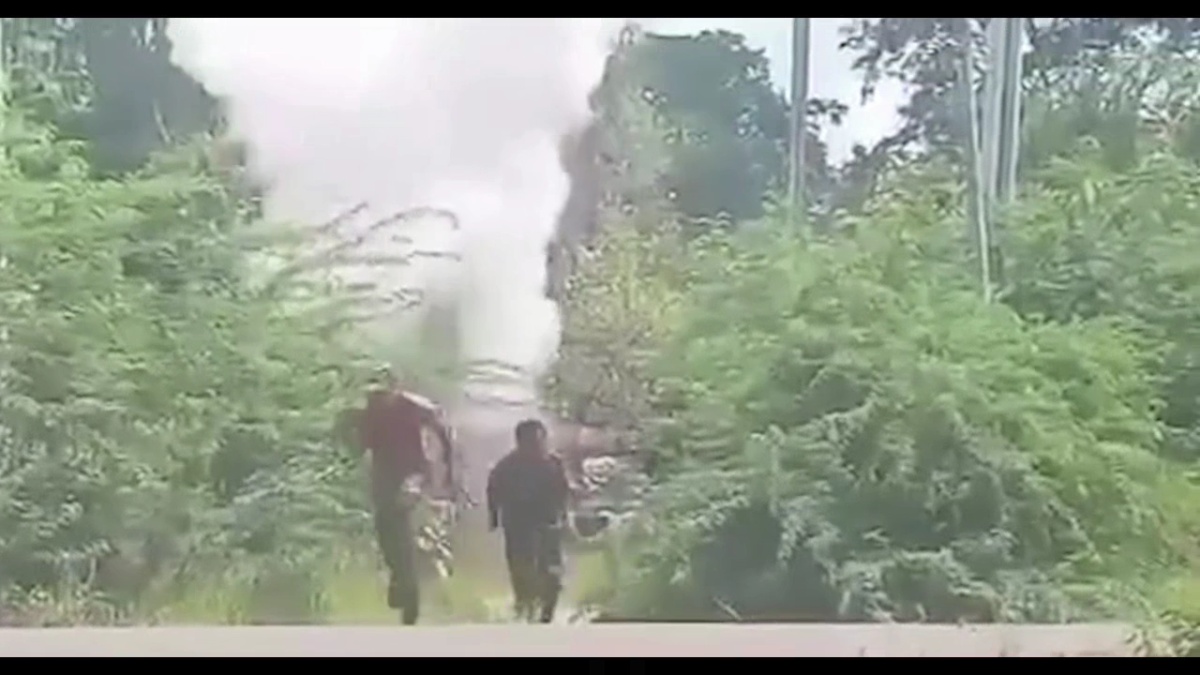
1. Machine Learning Algorithms
Self-Supervised Learning: Techniques that allow models to learn from unlabeled data by predicting parts of the data from other parts. Examples include BERT and GPT.
Few-Shot and Zero-Shot Learning: Methods enabling models to generalize from very few examples or even from none at all, improving efficiency in learning new tasks.
Reinforcement Learning: Advances in algorithms like Deep Q-Networks (DQN), Proximal Policy Optimization (PPO), and AlphaGo, leading to breakthroughs in game playing, robotics, and autonomous systems.
2. Neural Network Architectures
Transformers: The development of transformer architectures, such as BERT, GPT, and Vision Transformers (ViTs), has revolutionized NLP and is making significant inroads into computer vision.
Graph Neural Networks (GNNs): Used for tasks involving graph-structured data, such as social networks, molecule structure analysis, and recommendation systems.
Neural Architecture Search (NAS): Automated methods for finding optimal neural network architectures, such as EfficientNet, which balance performance and efficiency.
3. Natural Language Processing (NLP)
Large Language Models (LLMs): The creation of massive language models like GPT-3 and GPT-4 that can generate human-like text, perform translation, summarization, and answer questions.
Multimodal Models: Combining text, image, and other types of data in models like CLIP and DALL-E, enabling more comprehensive understanding and generation capabilities.
Conversational AI: Improvements in chatbots and virtual assistants, leading to more natural and context-aware interactions.
4. Computer Vision
Image Generation and Editing: Techniques such as Generative Adversarial Networks (GANs) and diffusion models enable the creation of realistic images and video, as well as sophisticated editing tools.
Object Detection and Segmentation: Advances in algorithms like YOLO (You Only Look Once), Mask R-CNN, and Segment Anything Model (SAM) for precise detection and segmentation in images and videos.
3D Vision: Progress in understanding and generating 3D models from 2D images, useful in areas like augmented reality (AR), virtual reality (VR), and robotics.
5. Cross-Disciplinary AI
AI in Healthcare: Development of AI models for diagnosing diseases, predicting patient outcomes, and personalizing treatment plans. Examples include AI for radiology, genomics, and drug discovery.
AI in Finance: Applications in fraud detection, algorithmic trading, risk management, and personalized financial advice.
AI in Climate Science: Use of AI to model climate change, optimize renewable energy sources, and predict environmental impacts.
6. Robustness and Fairness
Adversarial Robustness: Techniques to make AI models more resistant to adversarial attacks, ensuring reliability in critical applications.
Fairness and Bias Mitigation: Methods to detect and reduce biases in AI models, promoting fairness and equity in AI systems.
7. Explainability and Interpretability
Explainable AI (XAI): Development of tools and techniques to make AI models more interpretable, helping users understand how decisions are made. Examples include SHAP (SHapley Additive exPlanations) and LIME (Local Interpretable Model-agnostic Explanations).
8. Autonomous Systems
Self-Driving Cars: Progress in autonomous vehicle technology, including better perception, decision-making, and control systems.
Robotics: Advances in robotic manipulation, navigation, and human-robot interaction, leading to more capable and versatile robots.
9. Federated Learning
Privacy-Preserving AI: Techniques that allow models to be trained across multiple decentralized devices or servers while keeping data localized, enhancing privacy and security.
10. Quantum AI
Quantum Machine Learning: Exploration of quantum computing for AI, potentially offering exponential speed-ups for certain types of problems.
11. Ethical AI
AI Ethics and Governance: Research into the ethical implications of AI, including the development of guidelines and frameworks for responsible AI development and deployment.
12. AI Hardware
Specialized AI Chips: Development of hardware optimized for AI workloads, such as Google’s TPU, NVIDIA’s A100, and other custom AI accelerators, improving the efficiency and speed of AI computations.
Conclusion
AI research is advancing rapidly across multiple fronts, driven by both academic research and industry innovations. These advancements are enabling more powerful, efficient, and versatile AI systems that are transforming industries and improving our understanding of complex problems. As AI continues to evolve, ongoing research into its capabilities, limitations, and ethical implications will be crucial to ensure its benefits are realized in a responsible and equitable manner.
Leave a Reply