Successful AI product development requires a combination of technical expertise, strategic planning, and effective execution.
User-Centric Design: Understand your users’ needs and preferences to develop AI products that provide real value and a seamless user experience.



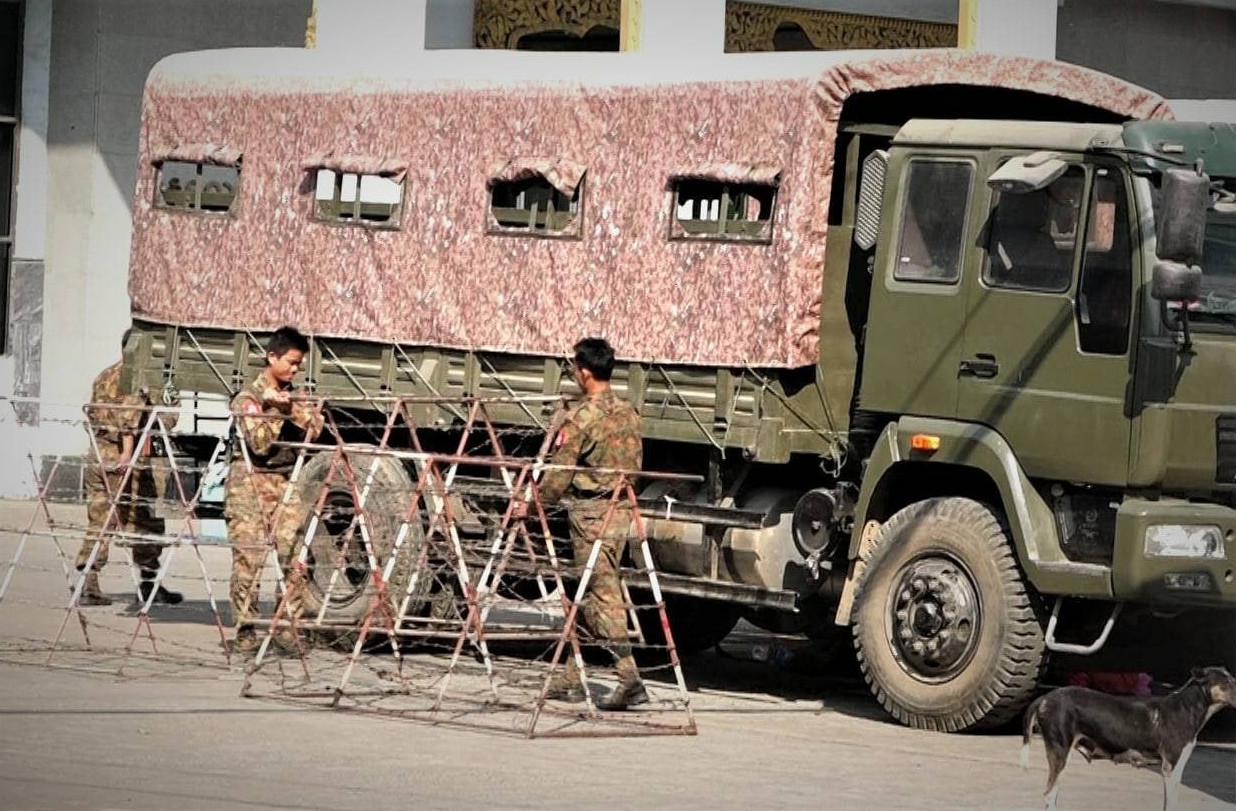
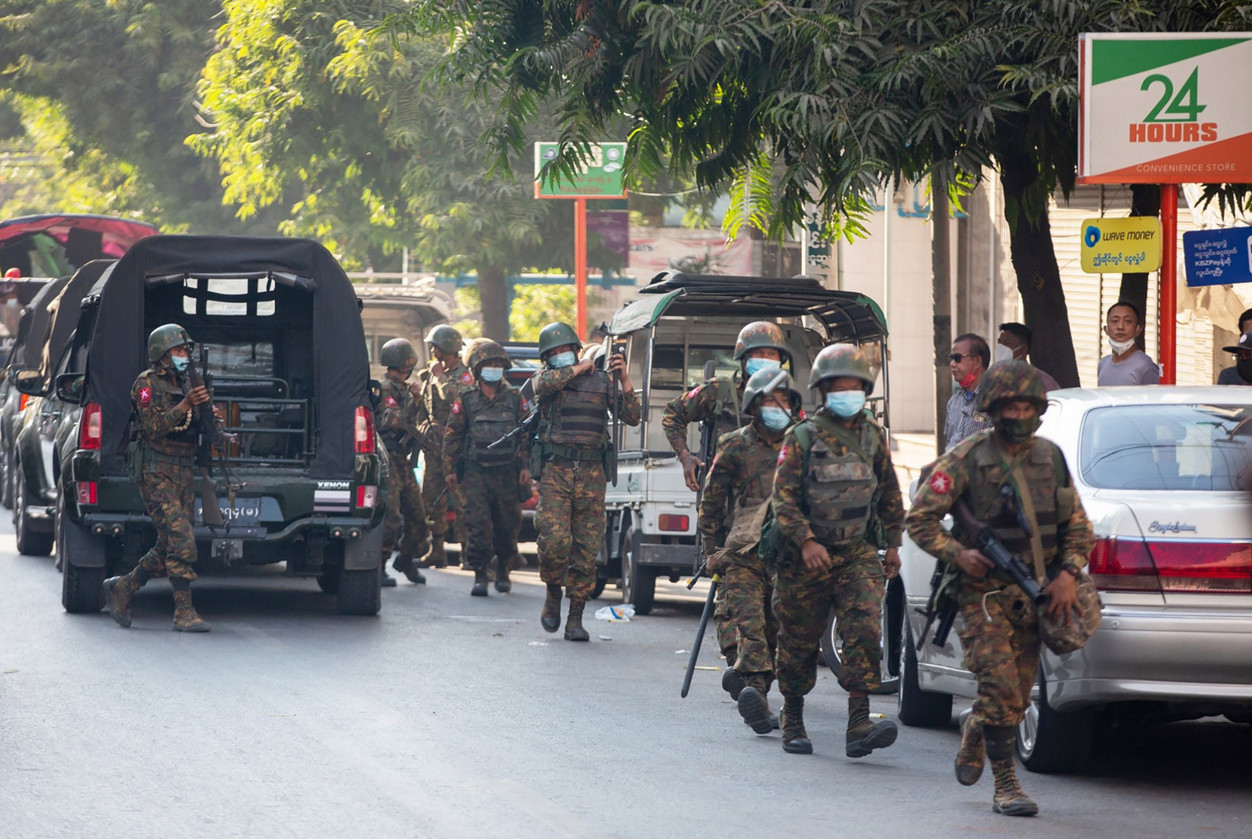

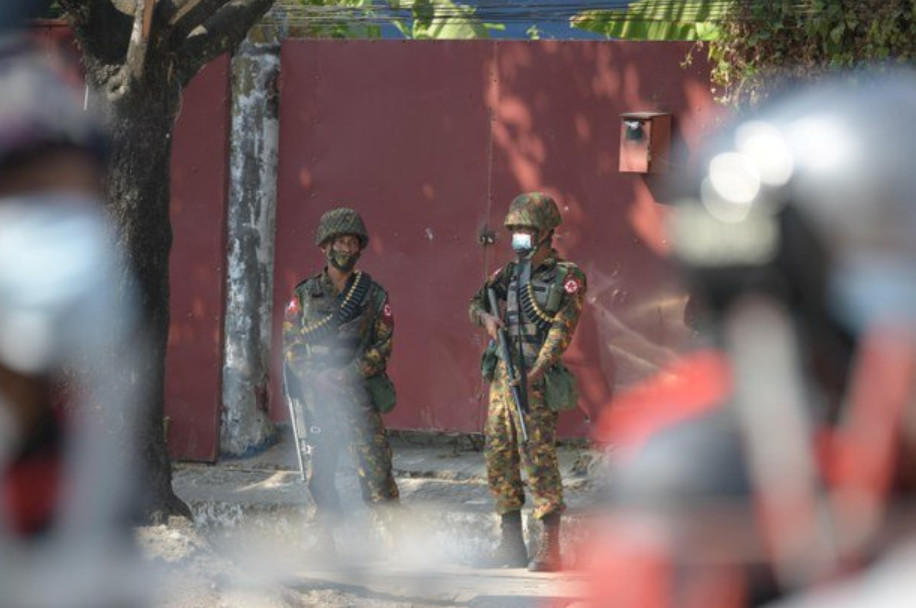
Involve user feedback throughout the development process to iterate and improve.
Data Quality and Ethics: Ensure that the data used to train AI models is of high quality, representative, and ethically sourced. Take measures to mitigate biases and ensure fairness and transparency in decision-making processes.
Scalability and Flexibility: Design AI products with scalability and flexibility in mind to accommodate growing user bases and evolving requirements. Use modular architectures and cloud-based solutions to facilitate scalability and easy updates.
Interpretability and Explainability: Aim for transparency in AI models to build trust and facilitate understanding among users and stakeholders. Provide explanations for model predictions and decisions, especially in critical applications like healthcare and finance.
Robustness and Security: Prioritize robustness and security to protect against potential vulnerabilities and malicious attacks. Implement measures such as data encryption, access controls, and regular security audits to safeguard sensitive data and ensure system reliability.
Regulatory Compliance: Stay informed about relevant regulations and compliance standards related to AI development and deployment. Ensure that your AI products adhere to legal and ethical guidelines, such as GDPR, HIPAA, and industry-specific regulations.
Continuous Improvement: Embrace a culture of continuous improvement by monitoring performance metrics, collecting user feedback, and iterating on your AI products over time. Regularly update models and algorithms to incorporate new data and insights and address emerging challenges.
Cross-Functional Collaboration: Foster collaboration between diverse teams, including data scientists, engineers, designers, product managers, and domain experts. Encourage open communication and knowledge sharing to leverage the collective expertise of the team.
Market Validation and Iteration: Validate your AI product in the market through pilot programs, beta testing, and early adopter feedback. Use these insights to iterate and refine your product to better meet user needs and achieve market fit.
Long-Term Vision: Have a clear long-term vision for your AI product and its potential impact on your target market. Continuously assess market trends, technological advancements, and emerging opportunities to adapt your strategy and stay competitive.
By adhering to these principles and best practices, you can increase the likelihood of success in AI product development and create innovative solutions that deliver tangible value to users and stakeholders.
Leave a Reply