The application of artificial intelligence (AI) and machine learning (ML) in risk assessment and underwriting is transforming the insurance industry.
These technologies offer significant improvements in accuracy, efficiency, and personalization. Here’s how they enhance these critical processes:

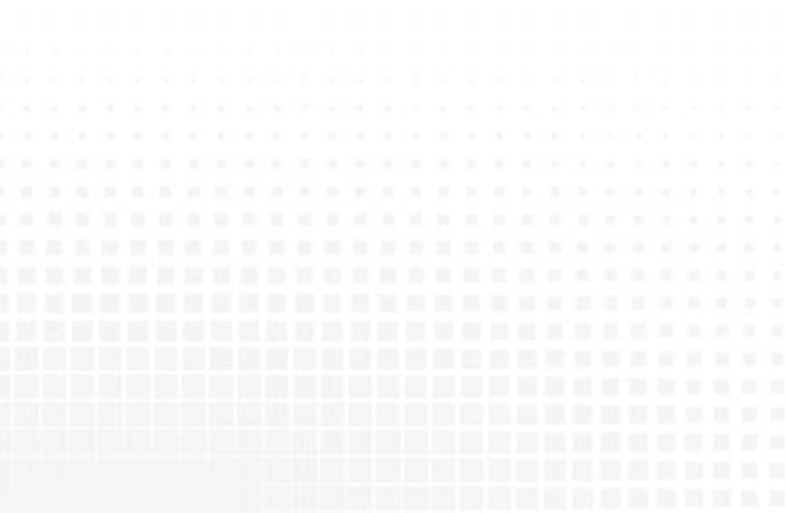



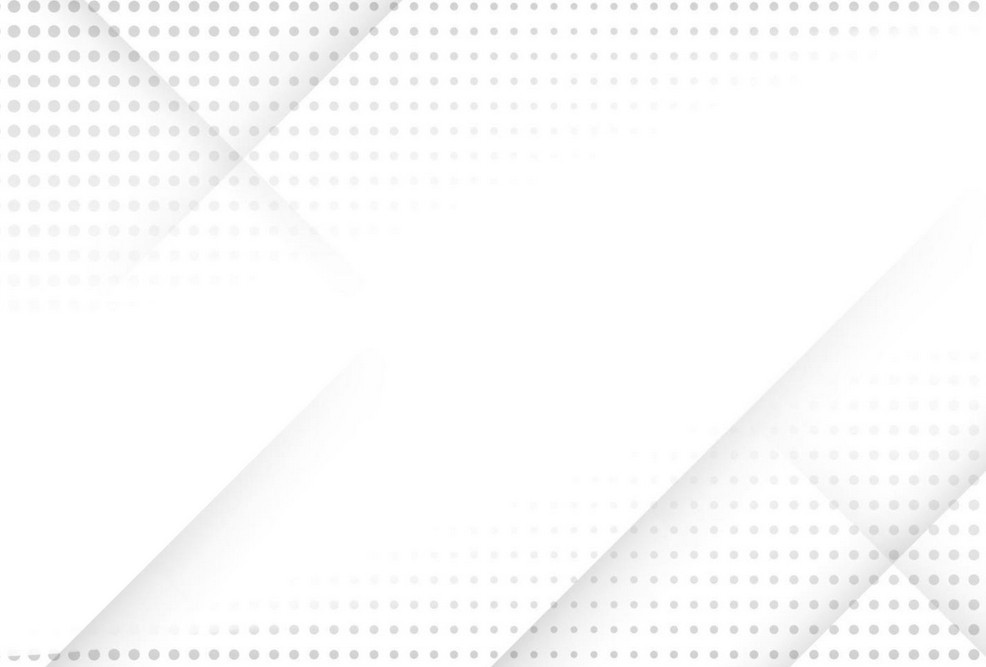

1. Data Integration and Analysis:
Diverse Data Sources: AI and ML systems can integrate data from a wide array of sources, including social media, telematics, IoT devices, financial records, and medical histories. This comprehensive data collection enables a more nuanced understanding of risk factors.
Real-Time Data Processing: These technologies can process and analyze data in real-time, allowing for immediate risk assessment and underwriting decisions. This is especially beneficial in dynamic environments where risks can change rapidly.
2. Predictive Modeling:
Risk Prediction: AI models can predict potential risks based on historical data and emerging trends. For instance, machine learning algorithms can analyze past claims data to identify patterns and predict future claim likelihoods.
Behavioral Analysis: By examining behavioral data, such as driving habits from telematics or health-related activities from wearable devices, insurers can better assess individual risk profiles and offer personalized premiums.
3. Personalized Underwriting:
Custom Risk Profiles: AI enables the creation of personalized risk profiles for each policyholder, taking into account their unique characteristics and behaviors. This leads to more accurate underwriting and fairer premium pricing.
Dynamic Adjustments: Machine learning models can continuously update risk assessments as new data becomes available, allowing for dynamic adjustments to underwriting decisions and policy terms.
4. Efficiency and Speed:
Automated Processes: AI automates many underwriting tasks, such as data collection, verification, and initial risk assessment. This reduces the time and effort required from human underwriters and speeds up the underwriting process.
Scalability: AI-powered systems can handle large volumes of underwriting tasks simultaneously, making it easier for insurers to scale their operations and manage peak periods without compromising accuracy.
5. Improved Accuracy:
Enhanced Precision: AI and ML algorithms can detect subtle patterns and correlations in data that might be missed by human underwriters. This leads to more precise risk assessments and reduces the likelihood of underestimating or overestimating risks.
Error Reduction: By automating repetitive and data-intensive tasks, AI minimizes human errors in the underwriting process, leading to more reliable outcomes.
6. Fraud Detection:
Anomaly Detection: AI systems can identify anomalies and suspicious patterns in applications and claims data, flagging potential fraud cases for further investigation. This proactive approach helps in mitigating fraud-related risks.
Historical Data Comparison: Machine learning models can compare current underwriting data with historical records to detect inconsistencies and unusual behaviors indicative of fraud.
7. Enhanced Customer Experience:
Faster Turnaround: The speed and efficiency of AI-driven underwriting processes lead to quicker policy issuance, enhancing customer satisfaction.
Transparent Communication: AI can provide clear explanations for underwriting decisions, helping customers understand the factors influencing their premiums and fostering trust.
Challenges and Considerations:
Data Quality: The effectiveness of AI and ML in underwriting depends on the quality and completeness of the data used. Insurers must ensure they have access to accurate and comprehensive data sets.
Bias and Fairness: AI models must be designed and monitored to avoid biases that could lead to unfair treatment of certain customer groups. Ensuring fairness and transparency in AI-driven decisions is crucial.
Regulatory Compliance: Insurers must navigate complex regulatory environments and ensure that their use of AI in underwriting complies with relevant laws and standards, particularly regarding data privacy and non-discrimination.
Conclusion:
The integration of AI and machine learning in risk assessment and underwriting represents a significant advancement for the insurance industry. These technologies enable more accurate, efficient, and personalized underwriting processes, leading to fairer pricing and better risk management. However, insurers must address challenges related to data quality, bias, and regulatory compliance to fully leverage the benefits of AI and ML in underwriting. As these technologies continue to evolve, their impact on risk assessment and underwriting will only grow, driving further innovation and improvement in the insurance sector.
Leave a Reply