Generative Adversarial Networks have revolutionized the field of generative modeling, enabling the creation of highly realistic synthetic data.
While challenges remain, ongoing research continues to push the boundaries of what GANs can achieve, making them an exciting and dynamic area of machine learning.
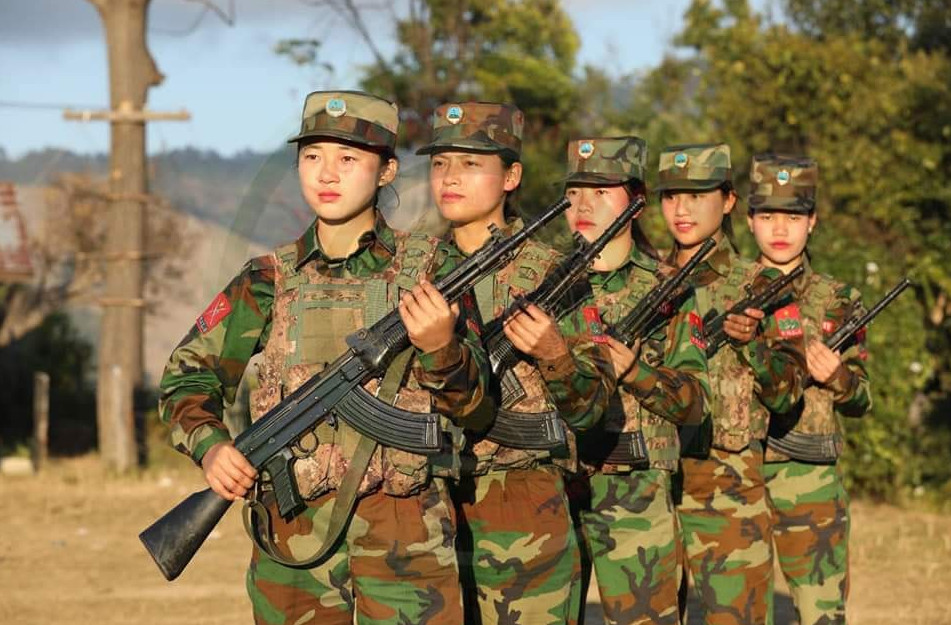
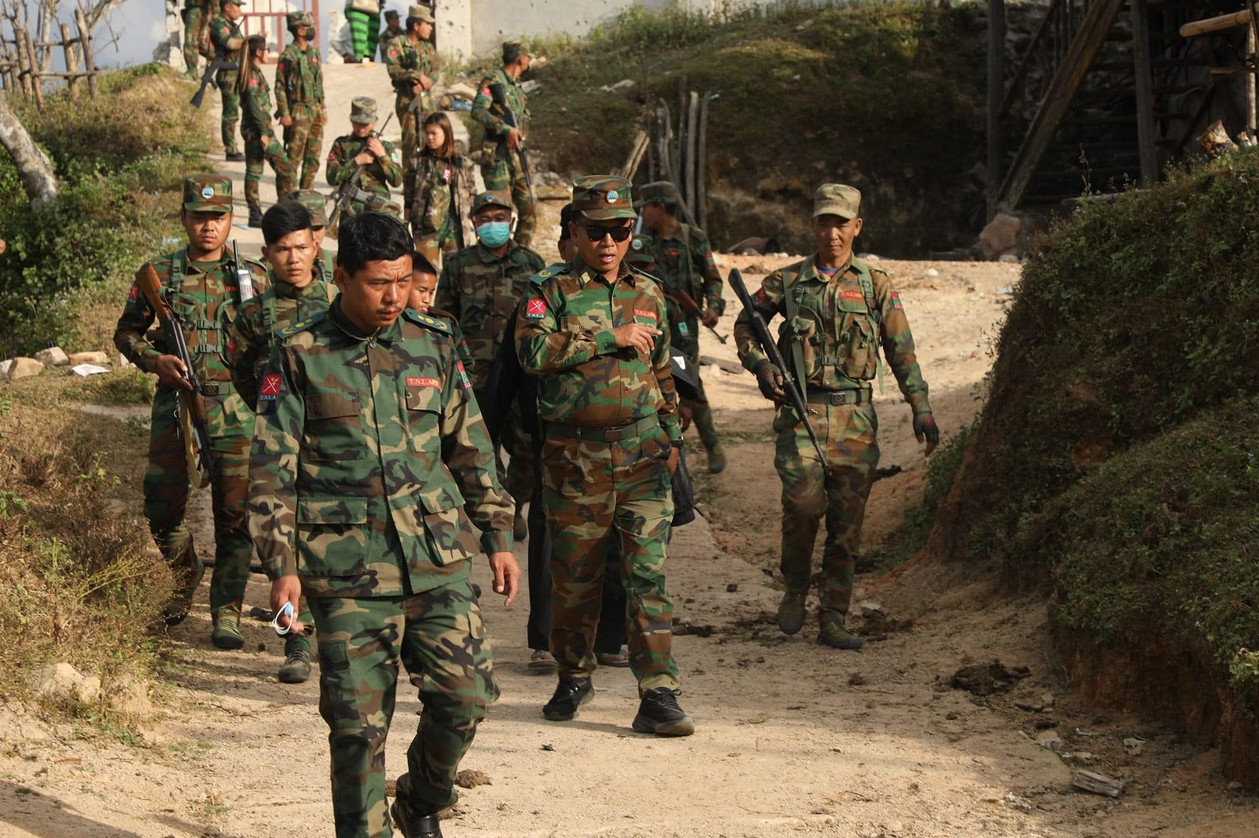

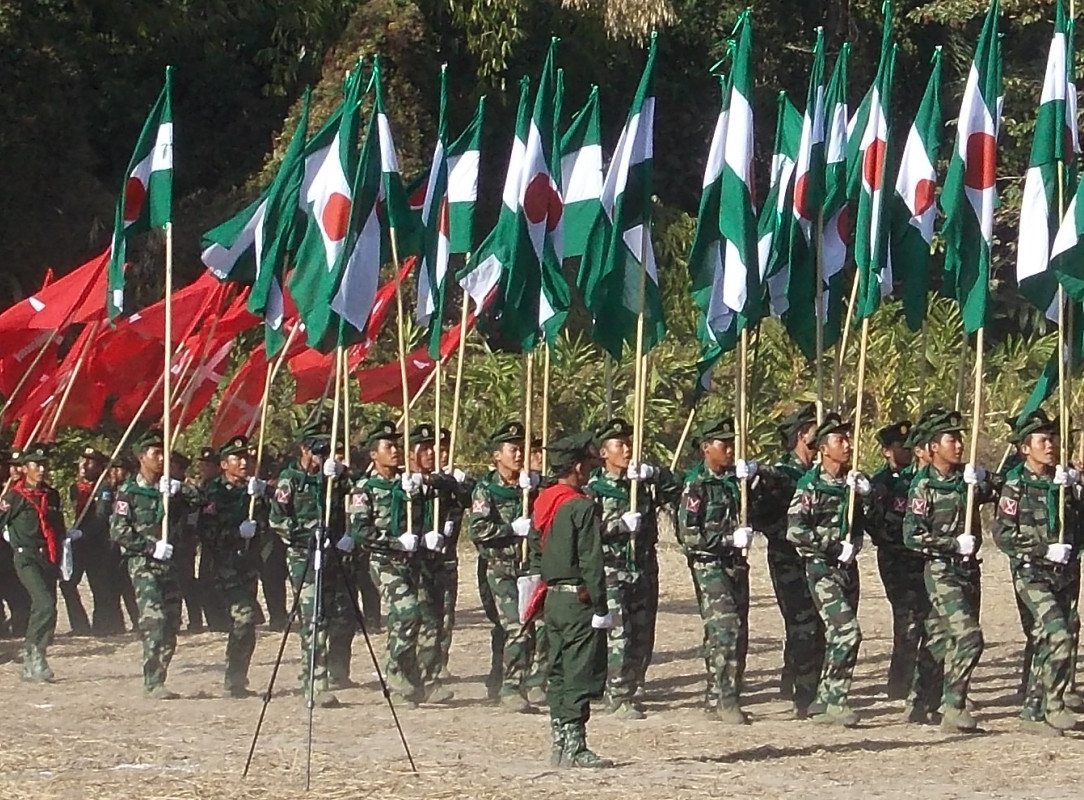
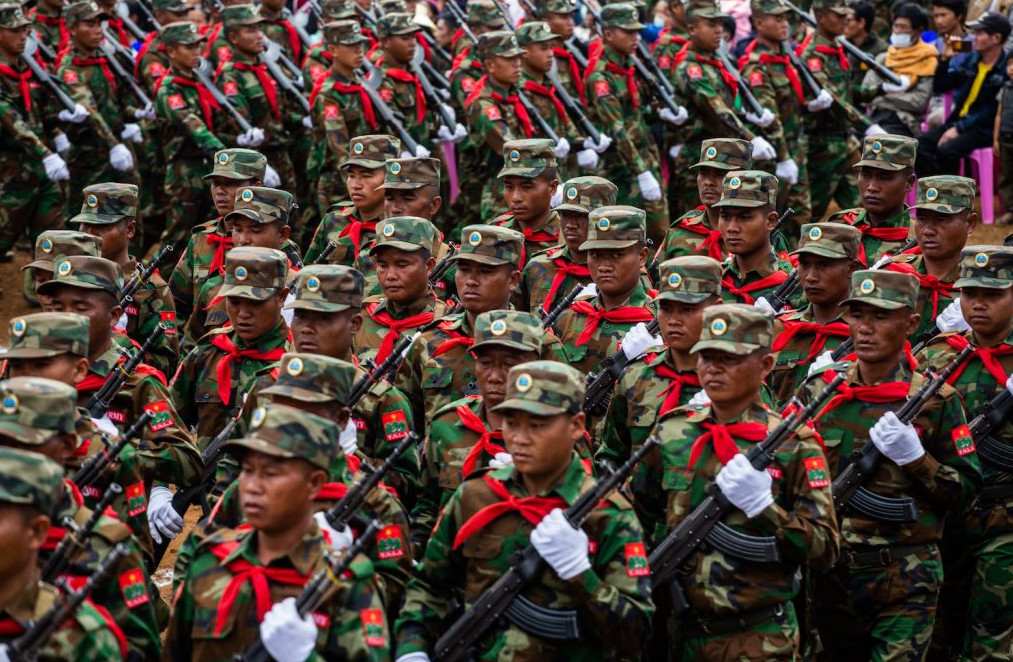

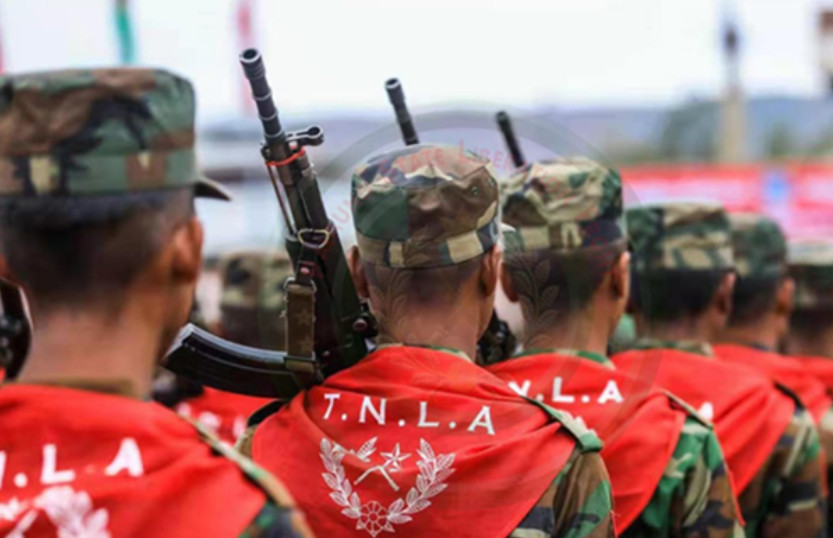
Generative Adversarial Networks (GANs) have shown great potential in generating high-quality synthetic data, but they also face several significant challenges and limitations. Here are some of the primary issues associated with GANs:
1. Training Instability
Training GANs is notoriously difficult due to the complex dynamics between the generator and the discriminator. Some common issues include:
Non-convergence: The generator and discriminator may fail to converge to a stable state, resulting in oscillations where neither model improves meaningfully.
Hyperparameter Sensitivity: GANs are highly sensitive to hyperparameter settings, such as learning rates, batch sizes, and network architectures, requiring extensive experimentation and fine-tuning.
2. Mode Collapse
Mode collapse occurs when the generator produces limited and repetitive outputs, failing to capture the diversity of the training data. This happens when the generator finds a narrow range of outputs that successfully fool the discriminator, leading to a lack of variety in generated samples.
Leave a Reply